Exploring the Impact of Machine-Learned Predictions on Feedback from an Artificial Limb.
2019 IEEE 16TH INTERNATIONAL CONFERENCE ON REHABILITATION ROBOTICS (ICORR)(2019)
摘要
Learning to get by without an arm or hand can be very challenging, and existing prostheses do not yet fill the needs of individuals with amputations. One promising solution is to improve the feedback from the device to the user. Towards this end, we present a simple machine learning interface to supplement the control of a robotic limb with feedback to the user about what the limb will be experiencing in the near future. A real-time prediction learner was implemented to predict impact-related electrical load experienced by a robot limb; the learning system's predictions were then communicated to the device's user to aid in their interactions with a workspace. We tested this system with five able-bodied subjects. Each subject manipulated the robot arm while receiving different forms of vibrotactile feedback regarding the arm's contact with its workspace. Our trials showed that using machine-learned predictions as a basis for feedback led to a statistically significant improvement in task performance when compared to purely reactive feedback from the device. Our study therefore contributes initial evidence that prediction learning and machine intelligence can benefit not just control, but also feedback from an artificial limb. We expect that a greater level of acceptance and ownership can be achieved if the prosthesis itself takes an active role in transmitting learned knowledge about its state and its situation of use.
更多查看译文
关键词
Amputation,Artificial Limbs,Feedback, Sensory,Humans,Machine Learning,Prosthesis Design,Robotics
AI 理解论文
溯源树
样例
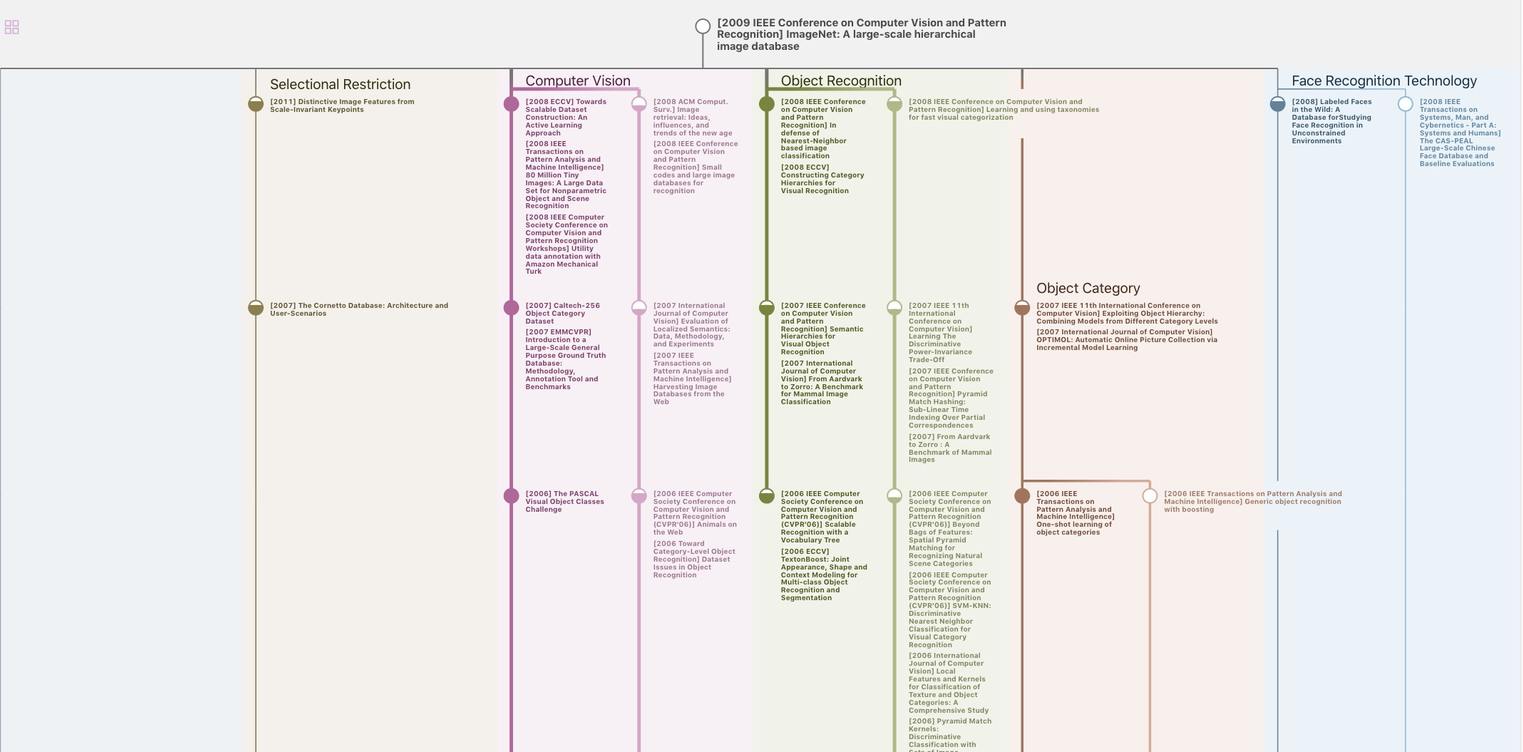
生成溯源树,研究论文发展脉络
Chat Paper
正在生成论文摘要