On the Number of Training Samples for Inverse Kinematics Solutions by Artificial Neural Networks
2019 16th International Conference on Ubiquitous Robots (UR)(2019)
摘要
For a robot manipulator, the conventional inverse kinematics (IK) solutions by geometric, algebraic, or Jacobian have drawbacks. They can be no closed form of the solution, problem dependency on the starting point, limited applicability of Jacobian, etc. To overcome those difficulties, IK analysis is approached by the Artificial Neural Networks (ANN) with its recent popularity. More training samples and hidden neurons are, better the IK function fitted by ANN performs. However, with a high number of samples and hidden neurons, the computational burdens are skyrocketing. This paper deals with the number of training samples for ANN to be applied for IK solutions. A 3 degrees-of-freedom (DOF) manipulator is taken for an example to test its validity. Through handful practices, the smallest sample set can be drawn from approximately 20 times greater sampling than an allowable tracking error size. In the case study, only 125 training set could give an acceptable tracking result.
更多查看译文
关键词
Inverse Kinematics,Artificial Neural Networks,Number of Training Samples,Robot Manipulator,Command Tracking
AI 理解论文
溯源树
样例
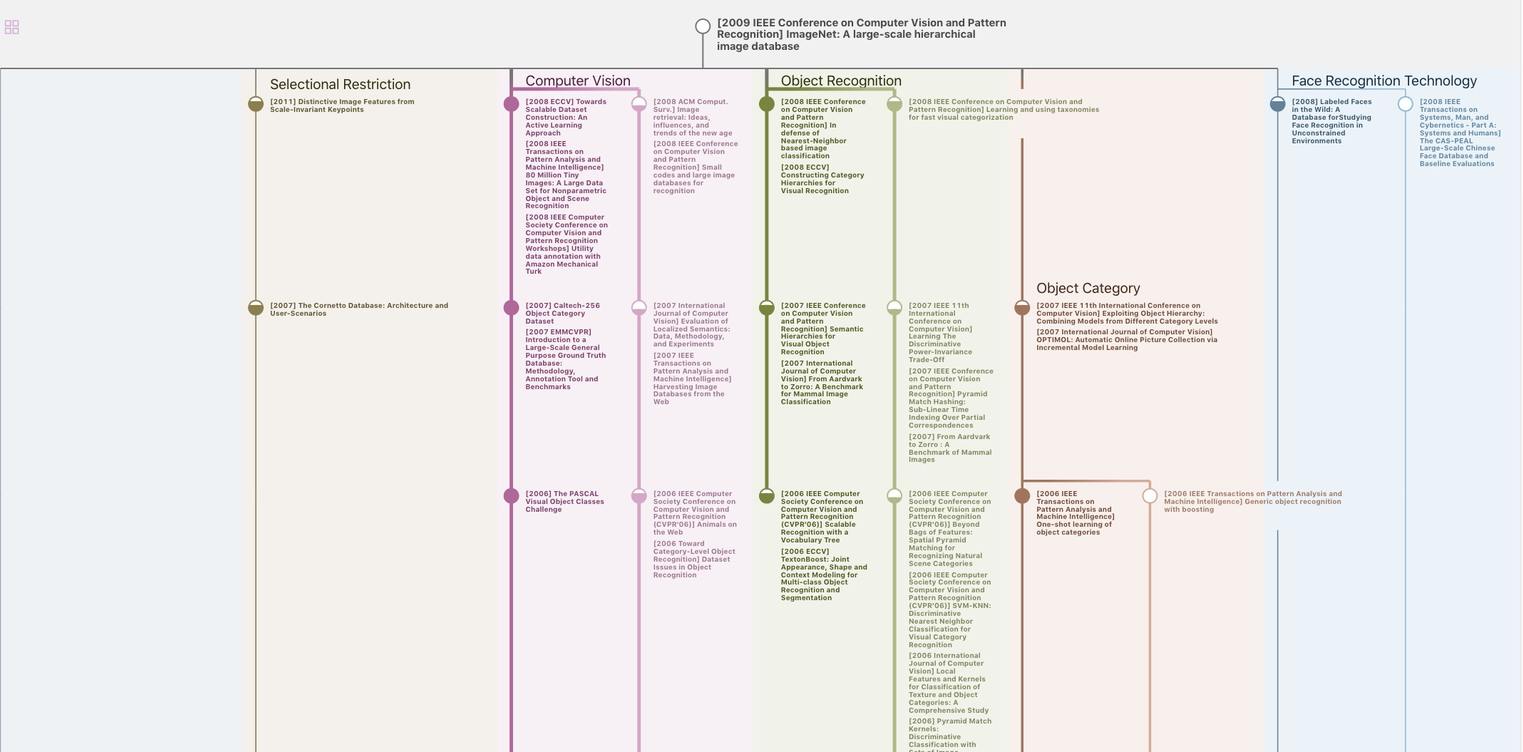
生成溯源树,研究论文发展脉络
Chat Paper
正在生成论文摘要