Semantic Concept Spaces: Guided Topic Model Refinement using Word-Embedding Projections.
IEEE Transactions on Visualization and Computer Graphics(2020)
摘要
We present a framework that allows users to incorporate the semantics of their domain knowledge for topic model refinement while remaining model-agnostic. Our approach enables users to (1)
understand
the semantic space of the model, (2)
identify
regions of potential conflicts and problems, and (3)
readjust
the semantic relation of concepts based on their understanding, directly influencing the topic modeling. These tasks are supported by an interactive visual analytics workspace that uses word-embedding projections to define
concept regions
which can then be refined. The user-refined concepts are independent of a particular document collection and can be transferred to related corpora. All user interactions within the concept space directly affect the semantic relations of the underlying vector space model, which, in turn, change the topic modeling. In addition to direct manipulation, our system guides the users' decision-making process through recommended interactions that point out potential improvements. This targeted refinement aims at minimizing the feedback required for an efficient human-in-the-loop process. We confirm the improvements achieved through our approach in two user studies that show topic model quality improvements through our visual knowledge externalization and learning process.
更多查看译文
关键词
Semantics,Analytical models,Computational modeling,Visual analytics,Machine learning,Task analysis
AI 理解论文
溯源树
样例
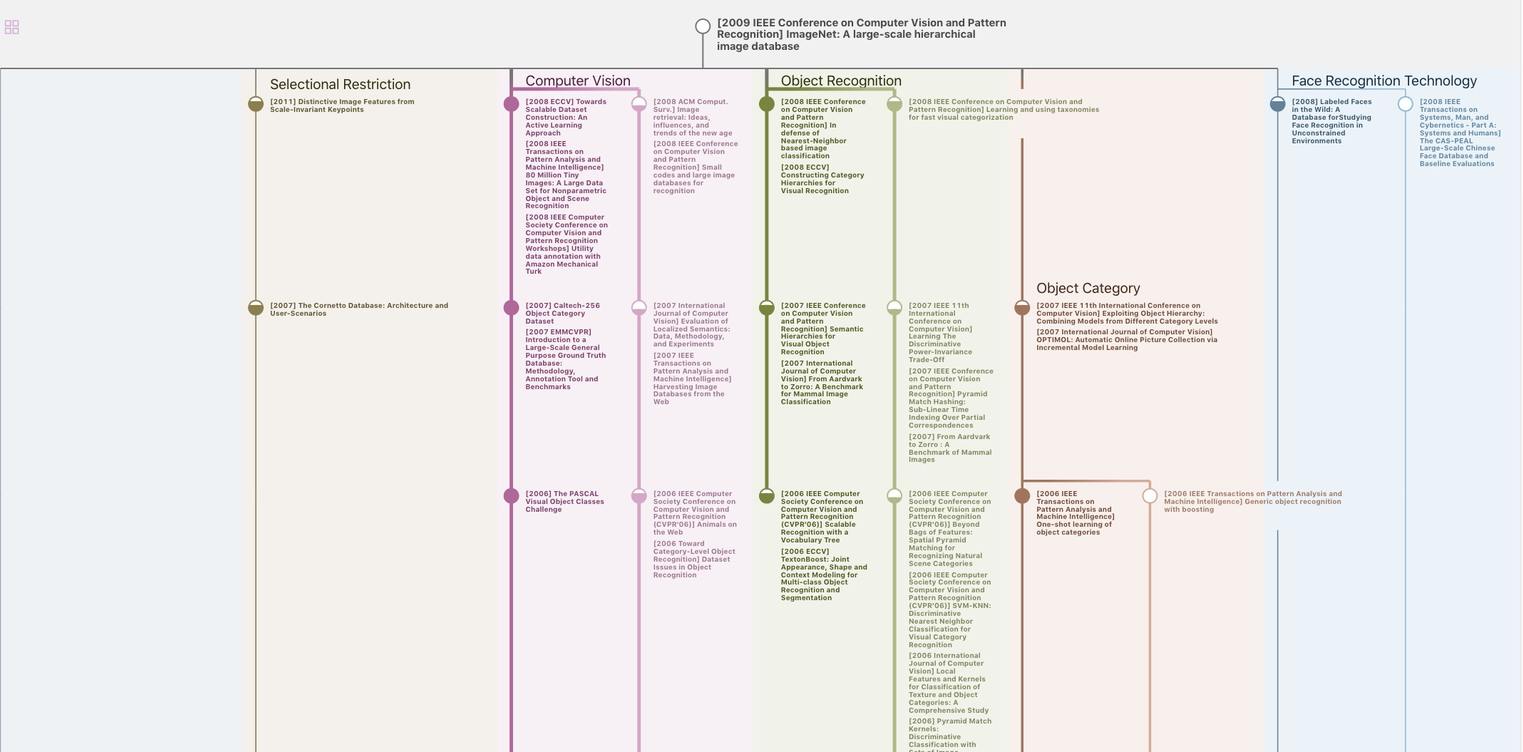
生成溯源树,研究论文发展脉络
Chat Paper
正在生成论文摘要