Embedding Human Heuristics In Machine-Learning-Enabled Probe Microscopy
MACHINE LEARNING-SCIENCE AND TECHNOLOGY(2020)
摘要
Scanning probe microscopists generally do not rely on complete images to assess the quality of data acquired during a scan. Instead, assessments of the state of the tip apex, which not only determines the resolution in any scanning probe technique, but can also generate a wide array of frustrating artefacts, are carried out in real time on the basis of a few lines of an image (and, typically, their associated line profiles.) The very small number of machine learning approaches to probe microscopy published to date, however, involve classifications based on full images. Given that data acquisition is the most time-consuming task during routine tip conditioning, automated methods are thus currently extremely slow in comparison to the tried-and-trusted strategies and heuristics used routinely by probe microscopists. Here, we explore various strategies by which different STM image classes (arising from changes in the tip state) can be correctly identified from partial scans. By employing a secondary temporal network and a rolling window of a small group of individual scanlines, we find that tip assessment is possible with a small fraction of a complete image. We achieve this with little-to-no performance penalty-or, indeed, markedly improved performance in some cases-and introduce a protocol to detect the state of the tip apex in real time.
更多查看译文
关键词
STM, SPM, automated STM, convolutional neural networks, real time machine learning, STM tip state
AI 理解论文
溯源树
样例
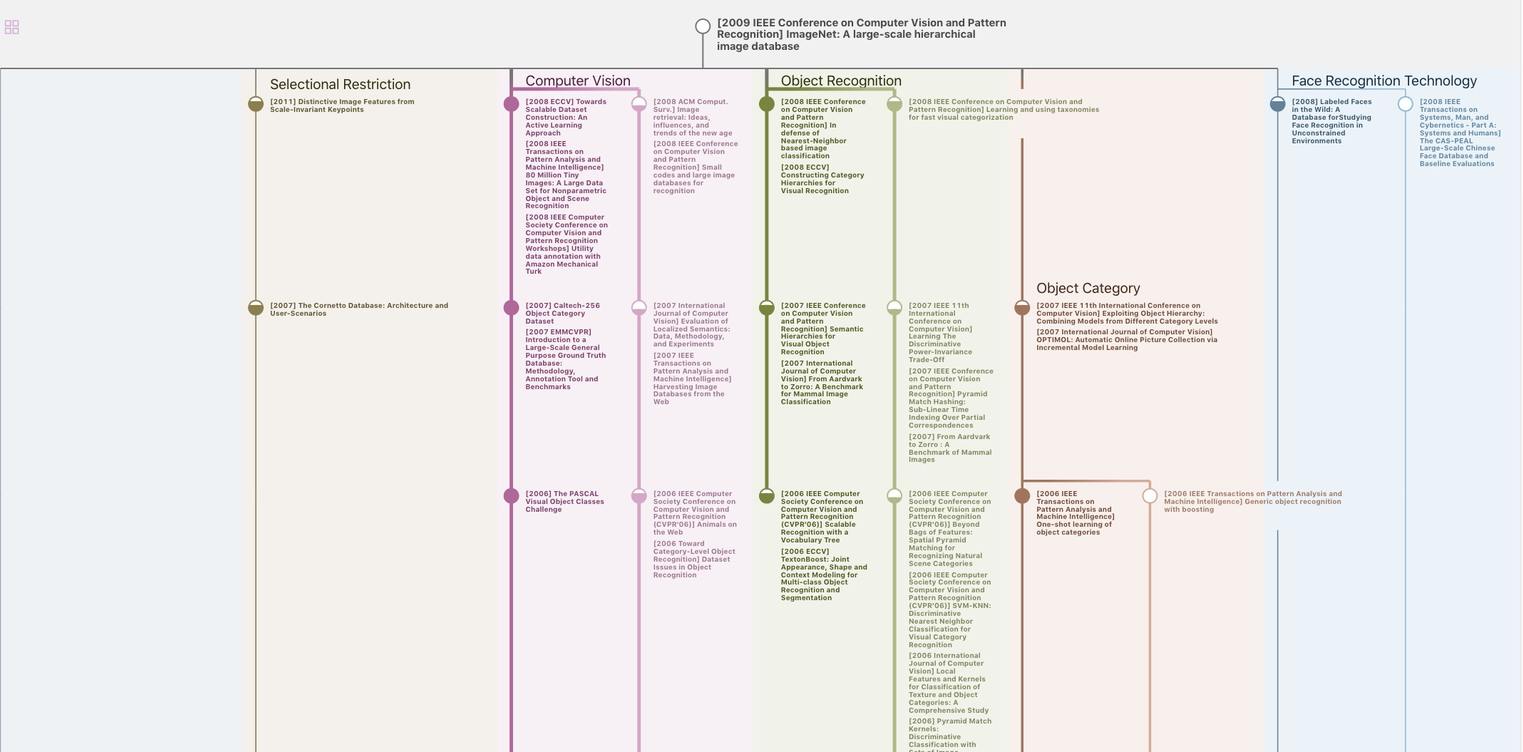
生成溯源树,研究论文发展脉络
Chat Paper
正在生成论文摘要