Unsupervised separation of dynamics from pixels
METRON(2019)
摘要
We present an approach to learn the dynamics of multiple objects from image sequences in an unsupervised way. We introduce a probabilistic model that first generate noisy positions for each object through a separate linear state-space model, and then renders the positions of all objects in the same image through a highly non-linear process. Such a linear representation of the dynamics enables us to propose an inference method that uses exact and efficient inference tools and that can be deployed to query the model in different ways without retraining.
更多查看译文
关键词
Variational auto-encoders,Linear Gaussian state space models,Deep neural networks
AI 理解论文
溯源树
样例
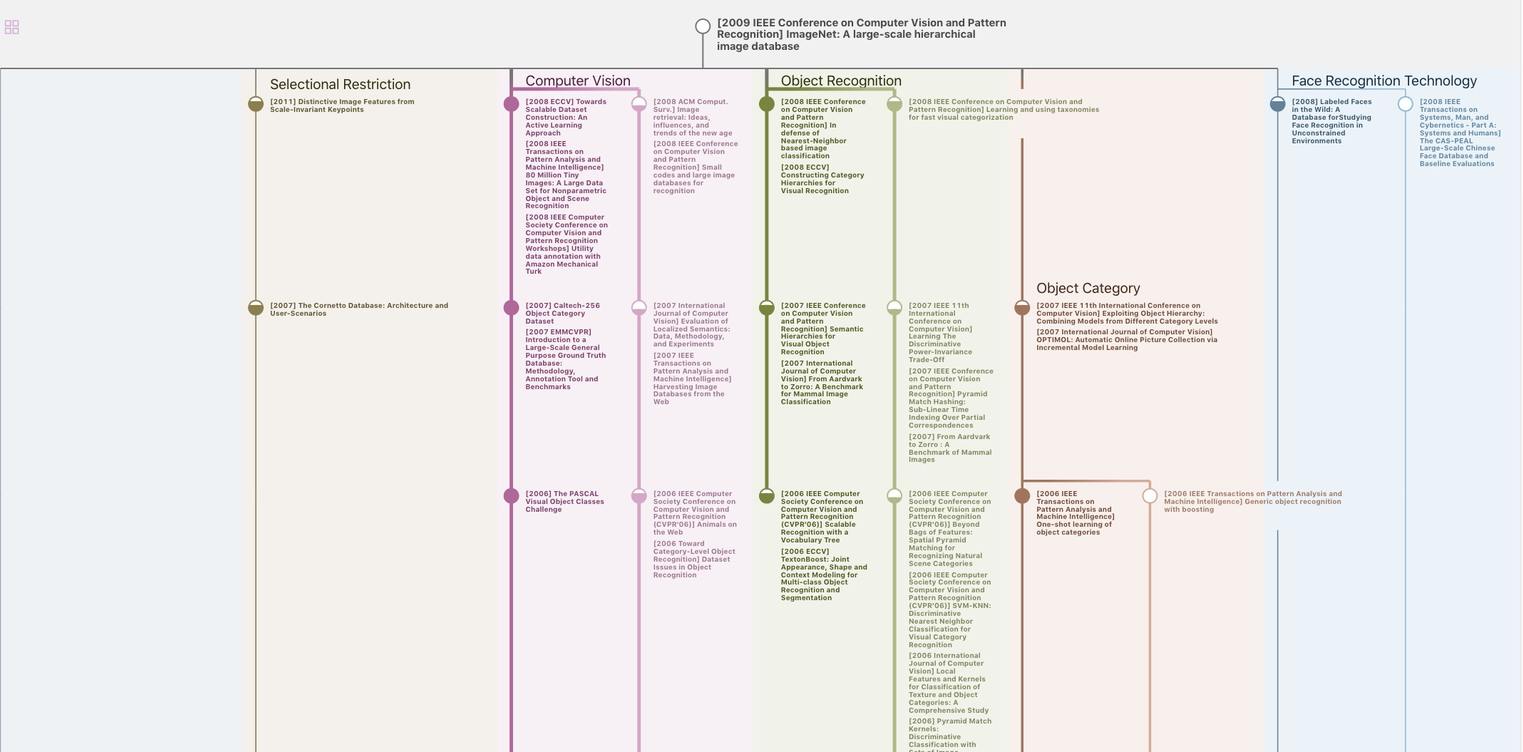
生成溯源树,研究论文发展脉络
Chat Paper
正在生成论文摘要