Stochastic approximation with nondecaying gain: Error bound and data-driven gain-tuning
INTERNATIONAL JOURNAL OF ROBUST AND NONLINEAR CONTROL(2020)
摘要
Our problem of interest is to minimize a sequence of scalar-valued loss functionsf(k)(center dot)or to locate the root(s) of a sequence of vector-valued functionsg(k)(center dot)corresponding to time tau kwith respect to a parameter theta is an element of Double-struck capital Rp. The available information for the minimization/root-finding is the noise-corrupted observation(s) of eitherf(k)(center dot)org(k)(center dot)evaluated at afewof certain design pointsonly. Taking both the dynamics and randomness into consideration, we use stochastic approximation (SA) algorithms to solve the time-varying problem, but with a nondecaying gain (stepsize). The tracking performance of the nondecaying gain SA algorithm is guaranteed by a computable bound for the root-mean-squared error and mean-absolute-deviation. The only assumption imposed on the drifts is that the average distance between two consecutive underlying true parameters is bounded from above-this assumption allows the varying target to change abruptly. The error term arising from using the noisy evaluation off(k)(center dot)org(k)(center dot)in constructing the search direction is assumed to have bounded second-moment-this allows for biased estimator to be used in the SA algorithms. Given the lenient assumptions on the drift and error term, the error bounds apply to a broad class of time-varying scenarios and are useful for finite-sample analysis. Since the tracking capability characterizes the average performance across all possible sample paths, it may not provide much guidance on the gain-tuning strategies useful for one single run. Hence, we propose a data-dependent gain-tuning strategy based on estimating the Hessian information and the noise level. The adaptive rule is advantageous as it enables the step size to vary with information gathered during the progress of the estimation procedure.
更多查看译文
关键词
nondecaying gain,simultaneous perturbation stochastic approximation,stochastic approximation,stochastic gradient descent,time-varying systems,tracking
AI 理解论文
溯源树
样例
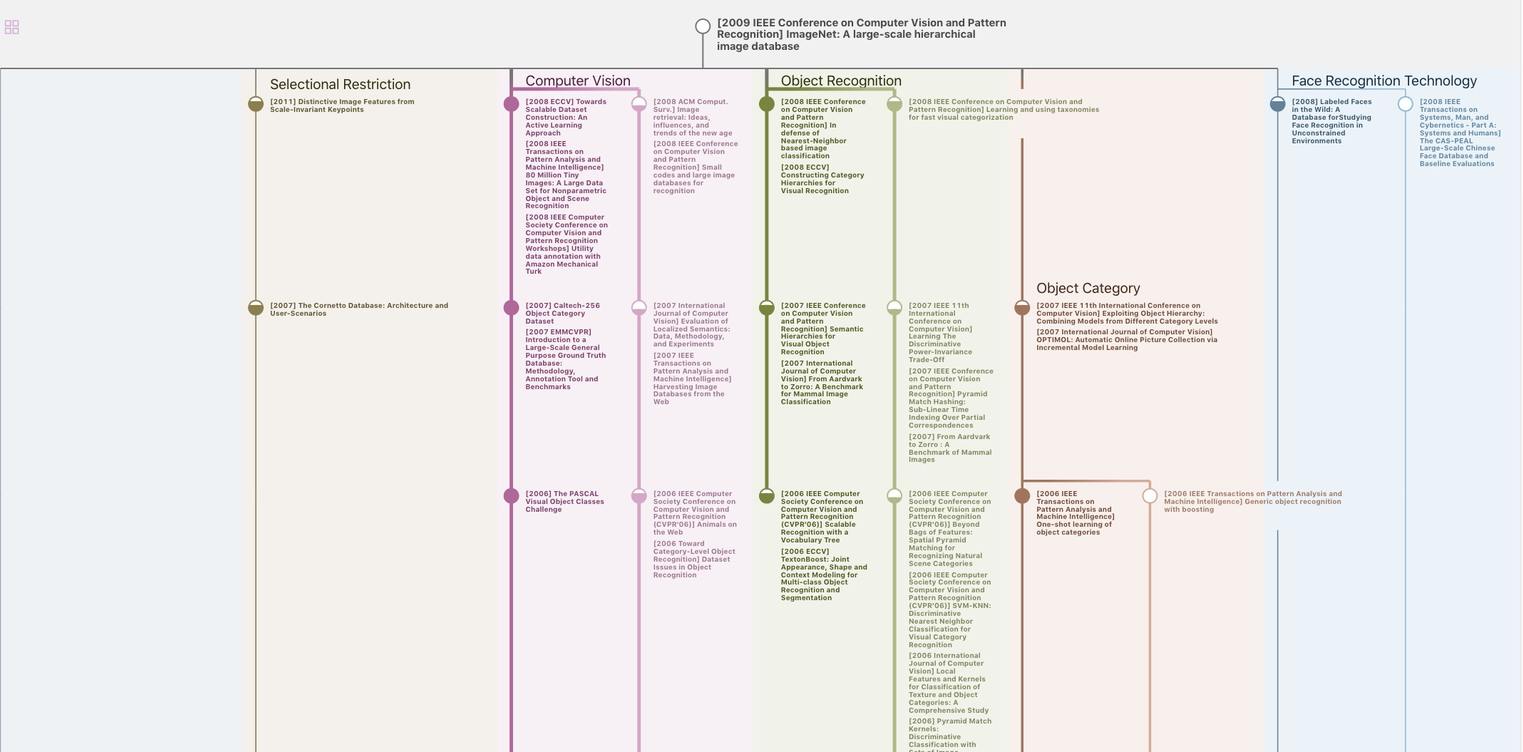
生成溯源树,研究论文发展脉络
Chat Paper
正在生成论文摘要