Many Could be Better Than All: A Novel Instance-Oriented Algorithm for Multi-modal Multi-label Problem
2019 IEEE International Conference on Multimedia and Expo (ICME)(2019)
摘要
With the emergence of diverse data collection techniques, objects in real applications can be represented as multi-modal features. What's more, objects may have multiple semantic meanings. Multi-modal and Multi-label [1] (MMML) problem becomes a universal phenomenon. The quality of data collected from different channels are inconsistent and some of them may not benefit for prediction. In real life, not all the modalities are needed for prediction. As a result, we propose a novel instance-oriented Multi-modal Classifier Chains (MCC) algorithm for MMML problem, which can make convince prediction with partial modalities. MCC extracts different modalities for different instances in the testing phase. Extensive experiments are performed on one real-world herbs dataset and two public datasets to validate our proposed algorithm, which reveals that it may be better to extract many instead of all of the modalities at hand.
更多查看译文
关键词
multi-modal, multi-label, extraction cost
AI 理解论文
溯源树
样例
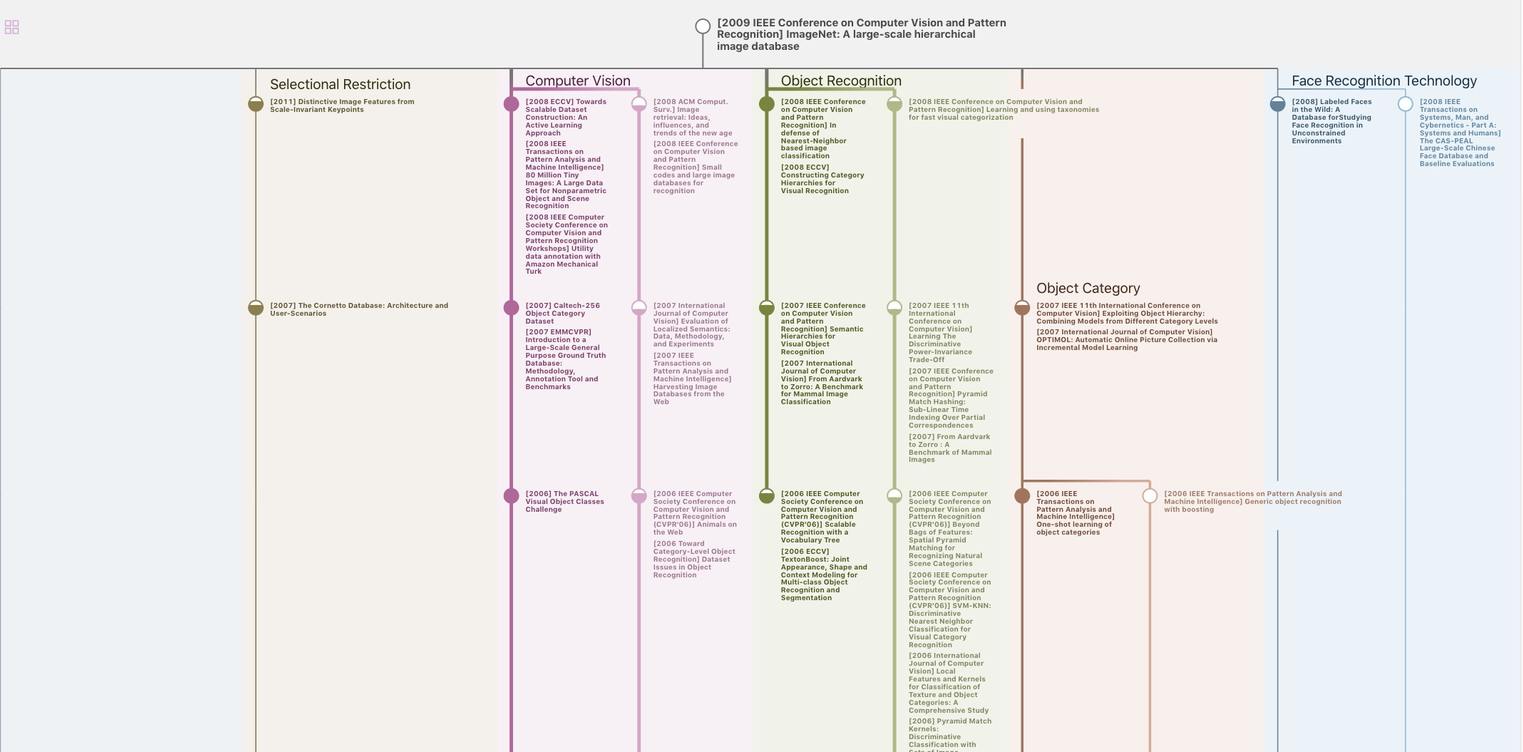
生成溯源树,研究论文发展脉络
Chat Paper
正在生成论文摘要