Adversarial Matching of Dark Net Market Vendor Accounts
KDD '19: The 25th ACM SIGKDD Conference on Knowledge Discovery and Data Mining Anchorage AK USA August, 2019(2019)
摘要
Many datasets feature seemingly disparate entries that actually refer to the same entity. Reconciling these entries, or "matching," is challenging, especially in situations where there are errors in the data. In certain contexts, the situation is even more complicated: an active adversary may have a vested interest in having the matching process fail. By leveraging eight years of data, we investigate one such adversarial context: matching different online anonymous marketplace vendor handles to unique sellers. Using a combination of random forest classifiers and hierarchical clustering on a set of features that would be hard for an adversary to forge or mimic, we manage to obtain reasonable performance (over 75% precision and recall on labels generated using heuristics), despite generally lacking any ground truth for training. Our algorithm performs particularly well for the top 30% of accounts by sales volume, and hints that 22,163 accounts with at least one confirmed sale map to 15,652 distinct sellers---of which 12,155 operate only one account, and the remainder between 2 and 11 different accounts. Case study analysis further confirms that our algorithm manages to identify non-trivial matches, as well as impersonation attempts.
更多查看译文
关键词
adversarial classification, dark net, measurements, record linkage
AI 理解论文
溯源树
样例
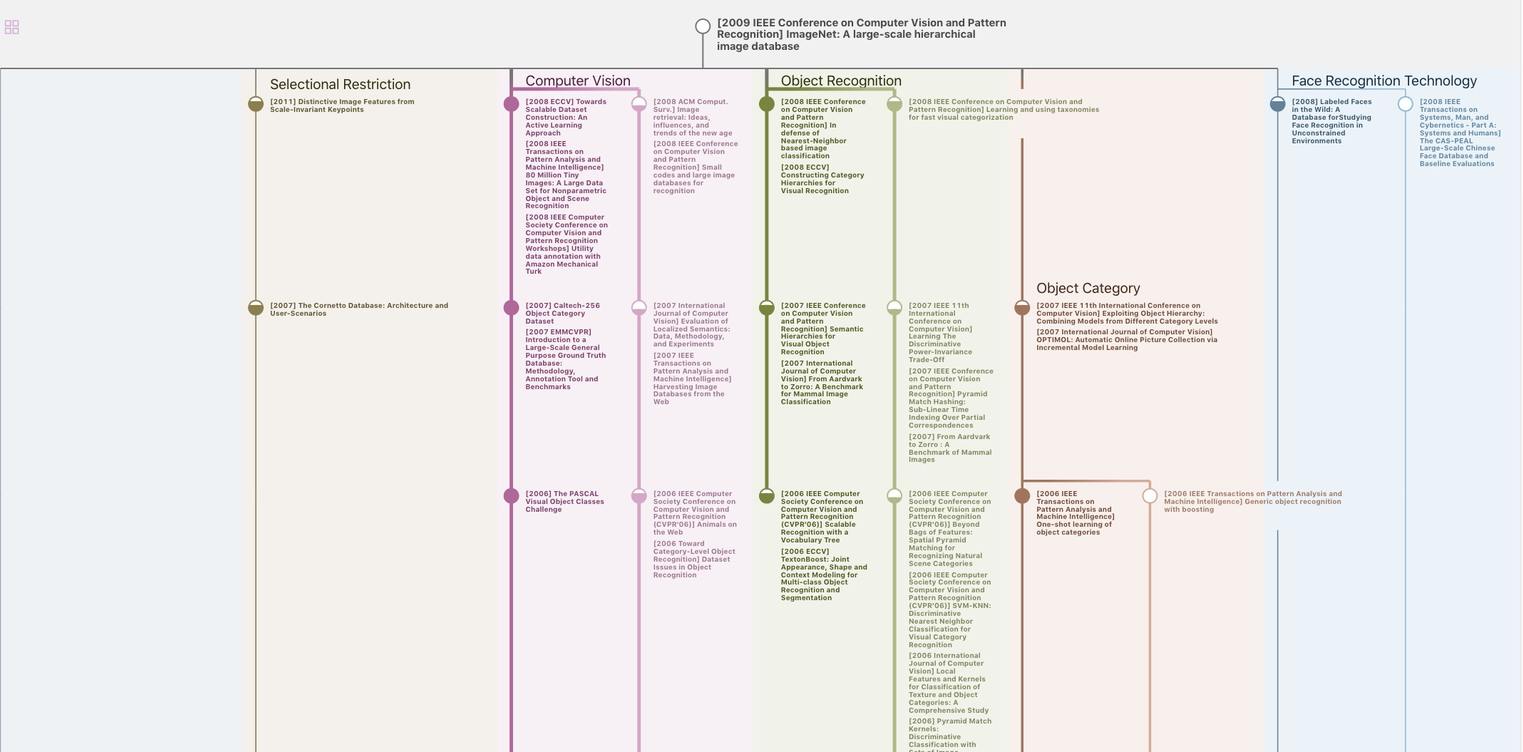
生成溯源树,研究论文发展脉络
Chat Paper
正在生成论文摘要