Online Amnestic DTW to allow Real-Time Golden Batch Monitoring
KDD '19: The 25th ACM SIGKDD Conference on Knowledge Discovery and Data Mining Anchorage AK USA August, 2019(2019)
摘要
In manufacturing, a golden batch is an idealized realization of the perfect process to produce the desired item, typically represented as a multidimensional time series of pressures, temperatures, flow-rates and so forth. The golden batch is sometimes produced from first-principle models, but it is typically created by recording a batch produced by the most experienced engineers on carefully cleaned and calibrated machines. In most cases, the golden batch is only used in post-mortem analysis of a product with an unexpectedly inferior quality, as plant managers attempt to understand where and when the last production attempt went wrong. In this work, we make two contributions to golden batch processing. We introduce an online algorithm that allows practitioners to understand if the process is currently deviating from the golden batch in real-time, allowing engineers to intervene and potentially save the batch. This may be done, for example, by cooling a boiler that is running unexpectedly hot. In addition, we show that our ideas can greatly expand the purview of golden batch monitoring beyond industrial manufacturing. In particular, we show that golden batch monitoring can be used for anomaly detection, attention focusing, and personalized training/skill assessment in a host of novel domains.
更多查看译文
关键词
anomaly detection, dynamic time warping, time series
AI 理解论文
溯源树
样例
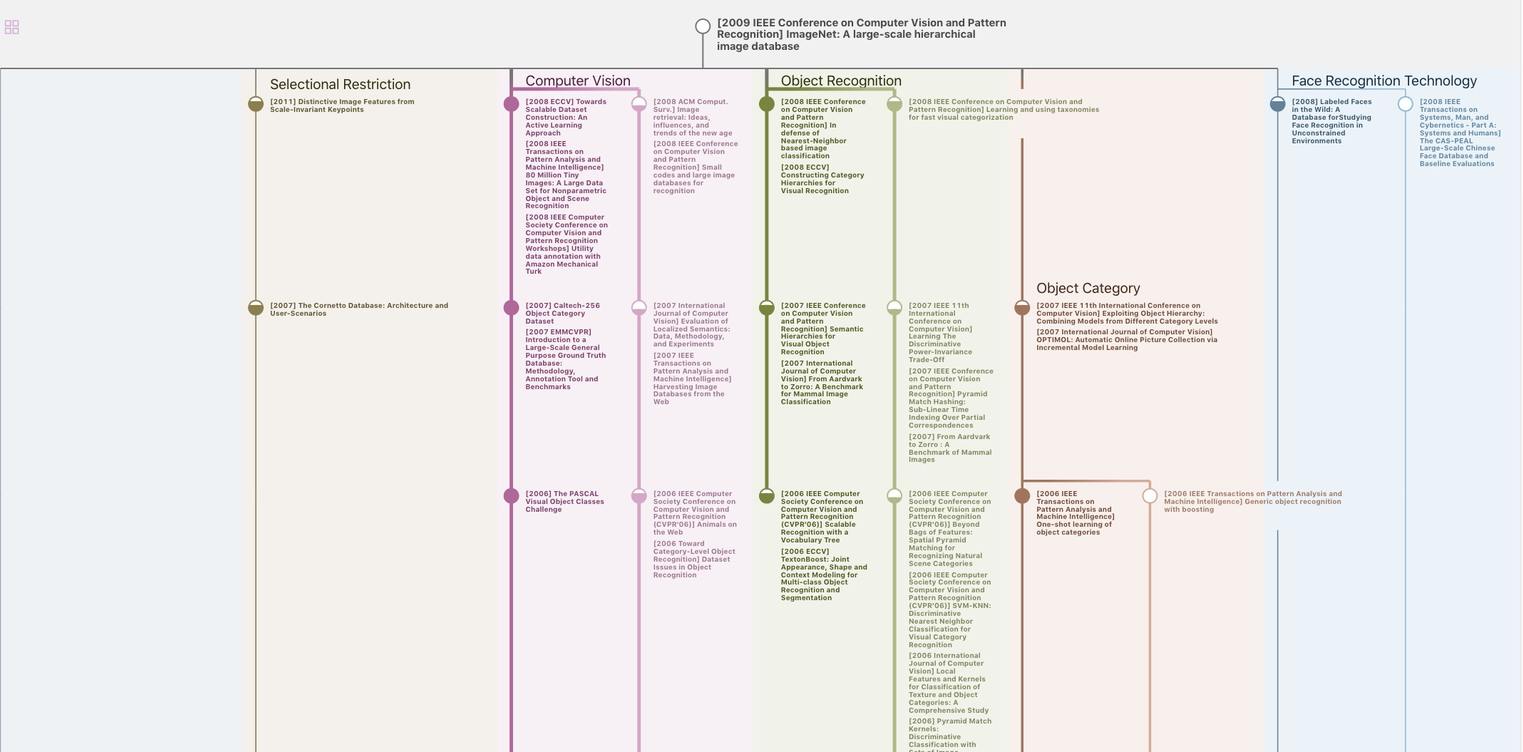
生成溯源树,研究论文发展脉络
Chat Paper
正在生成论文摘要