Noise-Tolerant, Deep-Learning-Based Radio Identification with Logarithmic Power Spectrum
ICC 2019 - 2019 IEEE International Conference on Communications (ICC)(2019)
摘要
Wireless devices have trivial individual differences due to hardware imperfections. Therefore, extracting unique features from the physical waveforms of wireless signals enables us to identify transmitter devices. It has often been assumed that high signal-to-noise ratio (SNR) waveforms can be obtained for identification in previous studies. However, there have been a small number of evaluation examples considering lower SNR environments. Classification accuracy could decrease when the feature is extracted from signals in a low SNR. In this paper, we propose a radio identification method using the logarithmic power spectral density (PSD) with a convolutional neural network (CNN) to improve identification performance in a low SNR environment. The simulation results based on indoor experiments reveals that the proposed method achieves 90 percent accuracy even at a low SNR condition of 7 dB, which is 11 dB lower than the conventional one. Additionally, the outdoor experiment results show that the proposed method achieves 99.4 percent accuracy, which is 6.5 points higher than the conventional one.
更多查看译文
关键词
classification accuracy,radio identification method,logarithmic power spectral density,convolutional neural network,low SNR environment,low SNR condition,outdoor experiment results,deep-learning-based radio identification,logarithmic power spectrum,wireless devices,hardware imperfections,physical waveforms,wireless signals,transmitter devices,high signal-to-noise ratio,SNR environments
AI 理解论文
溯源树
样例
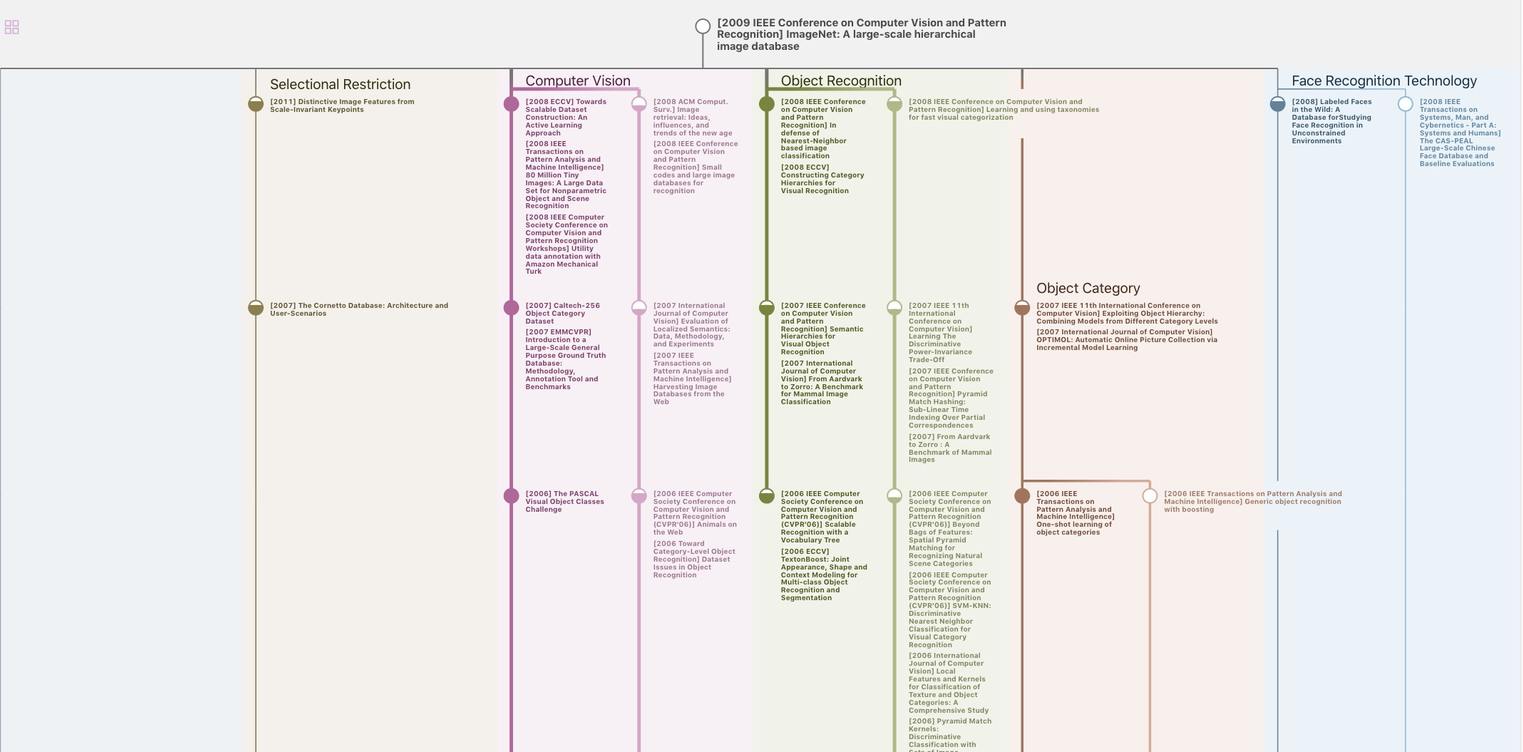
生成溯源树,研究论文发展脉络
Chat Paper
正在生成论文摘要