High-dimensional inference using the extremal skew- t process
Extremes(2020)
摘要
Max-stable processes are a popular tool for the study of environmental extremes, and the extremal skew- t process is a general model that allows for a flexible extremal dependence structure. For inference on max-stable processes with high-dimensional data, exact likelihood-based estimation is computationally intractable. Composite likelihoods, using lower dimensional components, and Stephenson-Tawn likelihoods, using occurrence times of maxima, are both attractive methods to circumvent this issue for moderate dimensions. In this article we establish the theoretical formulae for simulations of and inference for the extremal skew- t process. We also incorporate the Stephenson-Tawn concept into the composite likelihood framework, giving greater statistical and computational efficiency for higher-order composite likelihoods. We compare 2-way (pairwise), 3-way (triplewise), 4-way, 5-way and 10-way composite likelihoods for models of up to 100 dimensions. Furthermore, we propose cdf approximations for the Stephenson-Tawn likelihood function, leading to large computational gains, and enabling accurate fitting of models in large dimensions in only a few minutes. We illustrate our methodology with an application to a 90-dimensional temperature dataset from Melbourne, Australia.
更多查看译文
关键词
Extremes, Max-stable processes, Composite likelihood, Stephenson-Tawn likelihood, Quasi-Monte Carlo approximation, 60G70
AI 理解论文
溯源树
样例
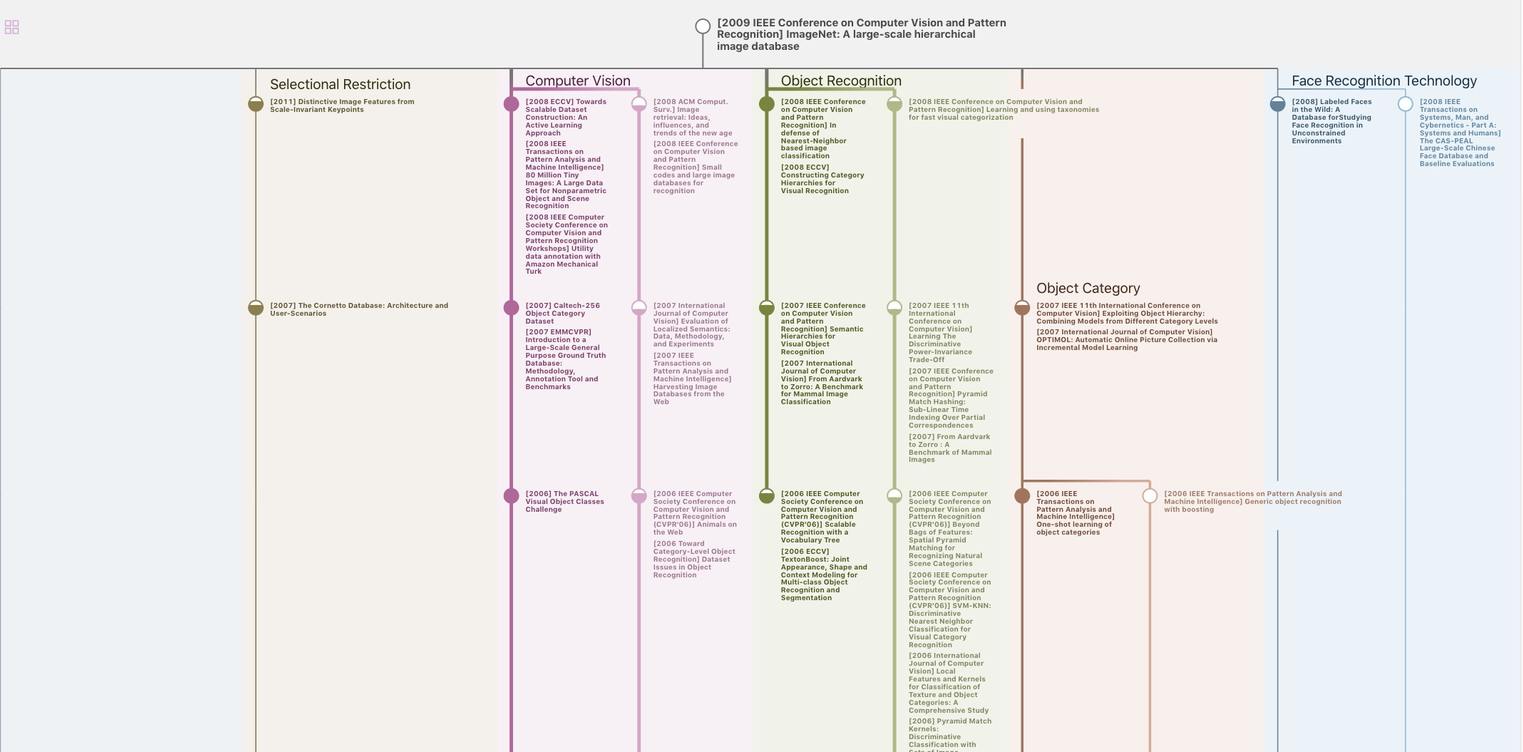
生成溯源树,研究论文发展脉络
Chat Paper
正在生成论文摘要