Few-Shot Learning for Domain-Specific Fine-Grained Image Classification
IEEE Transactions on Industrial Electronics(2021)
Abstract
Learning to recognize novel visual categories from a few examples is a challenging task for machines in real-world industrial applications. In contrast, humans have the ability to discriminate even similar objects with little supervision. This article attempts to address the few-shot fine-grained image classification problem. We propose a feature fusion model to explore discriminative features by focusing on key regions. The model utilizes the focus-area location mechanism to discover the perceptually similar regions among objects. High-order integration is employed to capture the interaction information among intraparts. We also design a center neighbor loss to form robust embedding space distributions. Furthermore, we build a typical fine-grained and few-shot learning dataset miniPPlankton from the real-world application in the area of marine ecological environments. Extensive experiments are carried out to validate the performance of our method. The results demonstrate that our model achieves competitive performance compared with state-of-the-art models. Our work is a valuable complement to the model domain-specific industrial applications.
MoreTranslated text
Key words
Computer vision,few-shot learning,representation learning
AI Read Science
Must-Reading Tree
Example
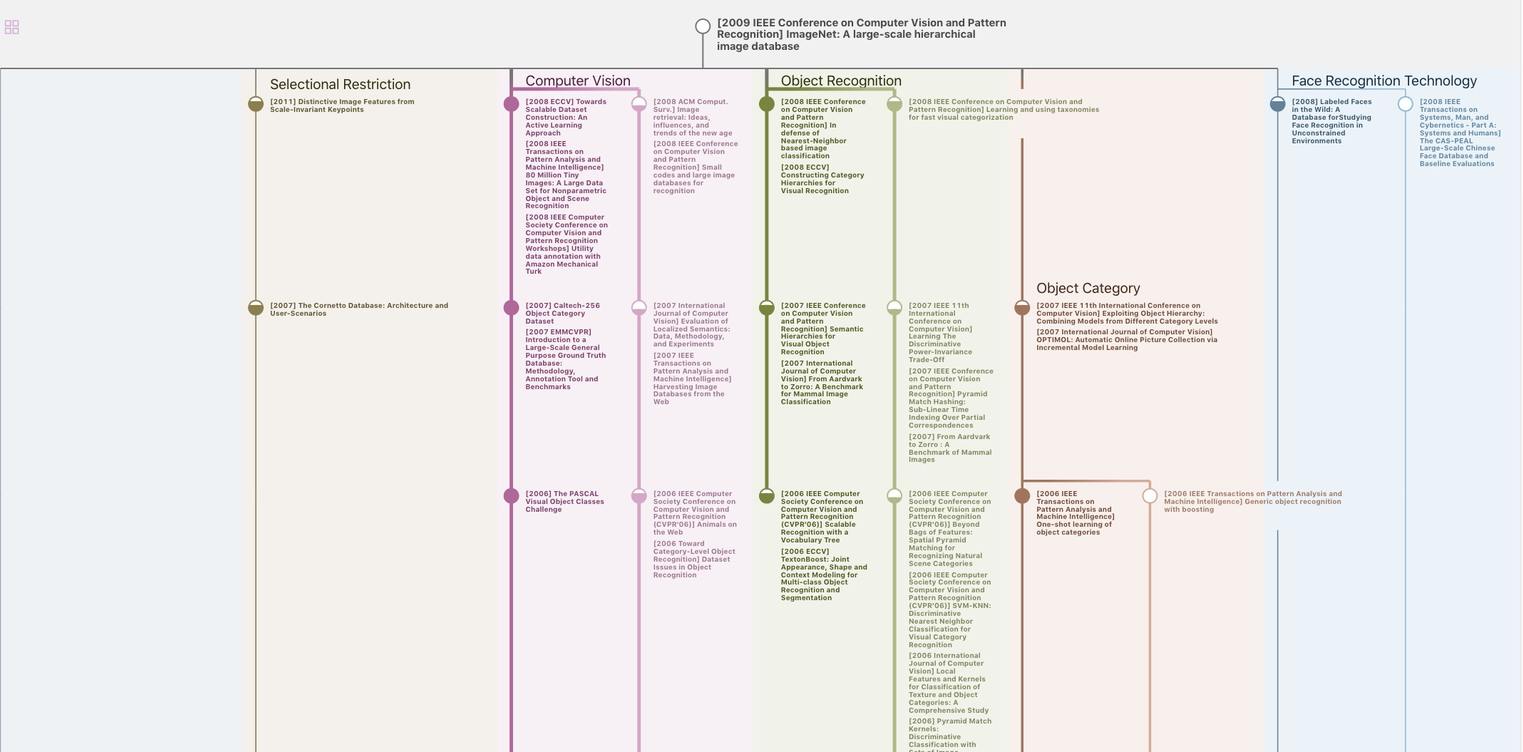
Generate MRT to find the research sequence of this paper
Chat Paper
Summary is being generated by the instructions you defined