Adapting Covariate Shift for Legal AI
ICAIL '19: Proceedings of the Seventeenth International Conference on Artificial Intelligence and Law(2019)
摘要
One of the fundamental assumptions with any machine learning (ML) system is that training data comes from the same distribution as the real world data. However, in m real-world applications, this important assumption is often violated including legal applications. A scenario where training and test samples follow different input feature distributions is known as covariate shift. This shift in data is often responsible for the deterioration in predictive performance of machine learning systems. The motivation of this research is to study the effect of covariate shift on deep learning systems used in legal research. In this work, we propose a unified framework to detect covariate shift on legal search queries impacting legal AI systems and formulate a strategy to adapt this shift on a periodic basis.
更多查看译文
关键词
Changing Environments, Covariate Shift, Data Fracture, Data Shift, Deep Learning, Legal Research, Named Entity Recognition, Non-Stationary Distributions, Query Intent, Recurrent Neural Networks, Selection Bias, Technical Debt in Machine Learning
AI 理解论文
溯源树
样例
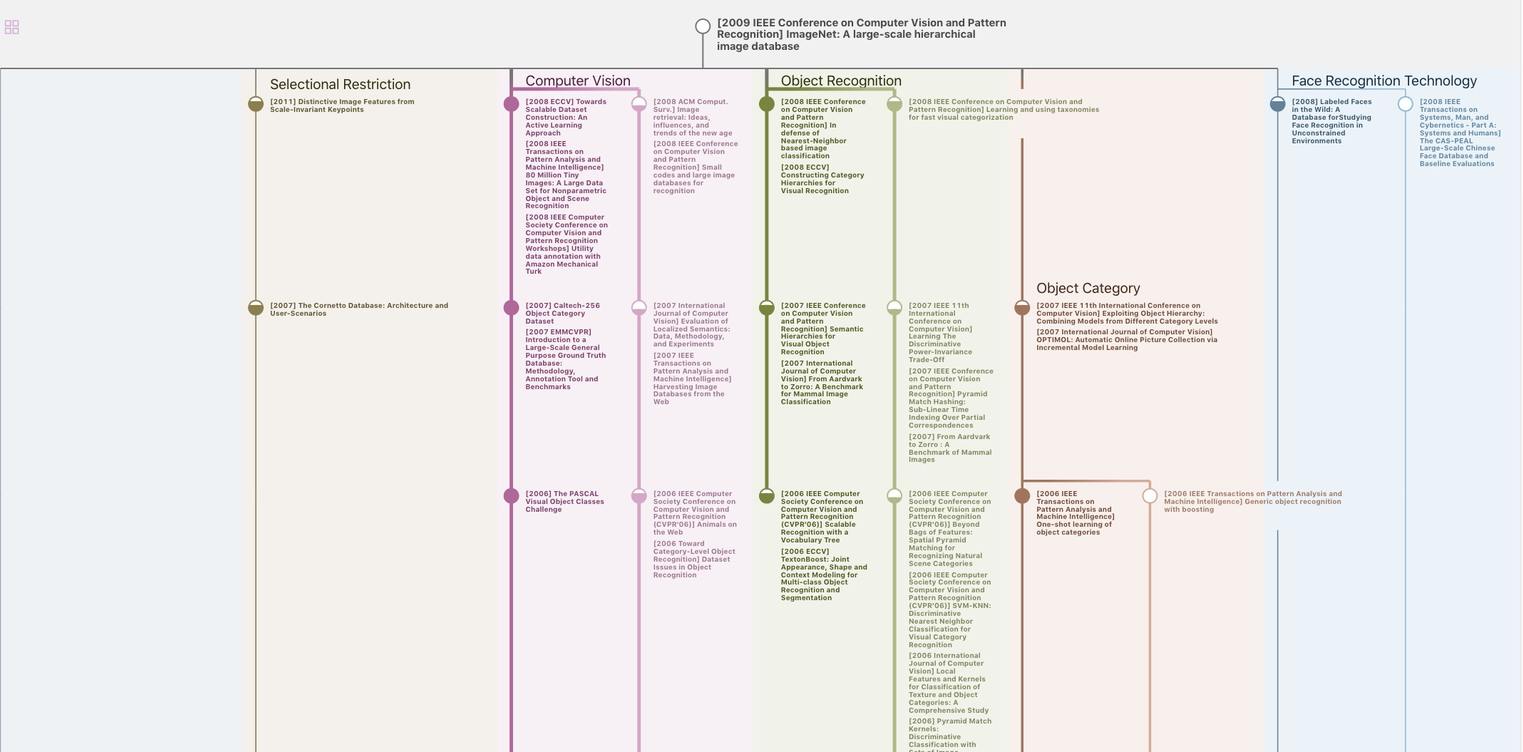
生成溯源树,研究论文发展脉络
Chat Paper
正在生成论文摘要