Neural Cross-Domain Collaborative Filtering with Shared Entities
MACHINE LEARNING AND KNOWLEDGE DISCOVERY IN DATABASES, ECML PKDD 2020, PT I(2021)
摘要
Cross-Domain Collaborative Filtering (CDCF) provides a way to alleviate data sparsity and cold-start problems present in recommendation systems by exploiting the knowledge from related domains. Existing CDCF models are either based on matrix factorization or deep neural networks. Independent use of either of the techniques in isolation may result in suboptimal performance for the prediction task. Also, most of the existing models face challenges particularly in handling diversity between domains and learning complex non-linear relationships that exist amongst entities (users/items) within and across domains. In thiswork, we propose an end-to-end neural networkmodel - NeuCDCF, to address these challenges in a cross-domain setting. More importantly, NeuCDCFis based on a wide and deep framework and learns the representations jointly using both matrix factorization and deep neural networks. We perform experiments on four real-world datasets and demonstrate that our model performs better than state-of-the-art CDCF models.
更多查看译文
关键词
Cross-domain collaborative filtering, Deep learning, Neural networks, Wide and deep framework, Recommendation system
AI 理解论文
溯源树
样例
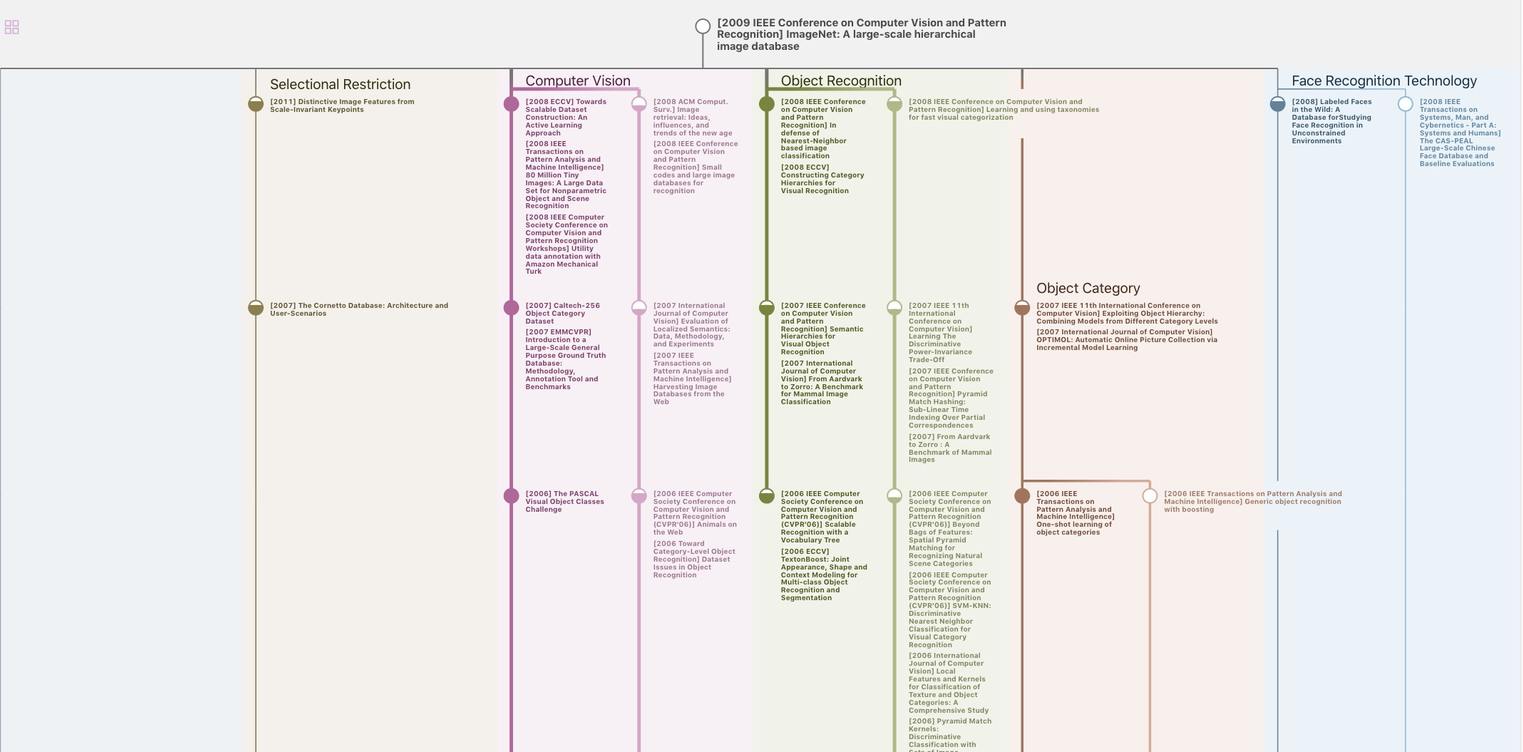
生成溯源树,研究论文发展脉络
Chat Paper
正在生成论文摘要