A Classification Method Based on Feature Selection for Imbalanced Data.
IEEE ACCESS(2019)
摘要
Imbalanced data are very common in the real world, and it may deteriorate the performance of the conventional classification algorithms. In order to resolve the imbalanced classification problems, we propose an ensemble classification method that combines evolutionary under-sampling and feature selection. We employ the Bootstrap method in original data to generate many sample subsets. V-statistic is developed to measure the distribution of imbalanced data, and it is also taken as the optimization objective of the genetic algorithm for the under-sampling sample subsets. Moreover, we take F-1 and Gmean indicators as two optimization objectives and employ the multiobjective ant colony optimization algorithm for feature selection of resampled data to construct an ensemble system. Ten low-dimensional and four high-dimensional typical imbalanced datasets are used in experiments. The six state-of-the-art algorithms and four measures are taken for a fair comparison. The experimental results show that our proposed system has a better classification performance compared with other algorithms, especially for the high-dimensional imbalanced data.
更多查看译文
关键词
Feature selection,imbalanced data,multiobjective ant colony optimization,genetic algorithm
AI 理解论文
溯源树
样例
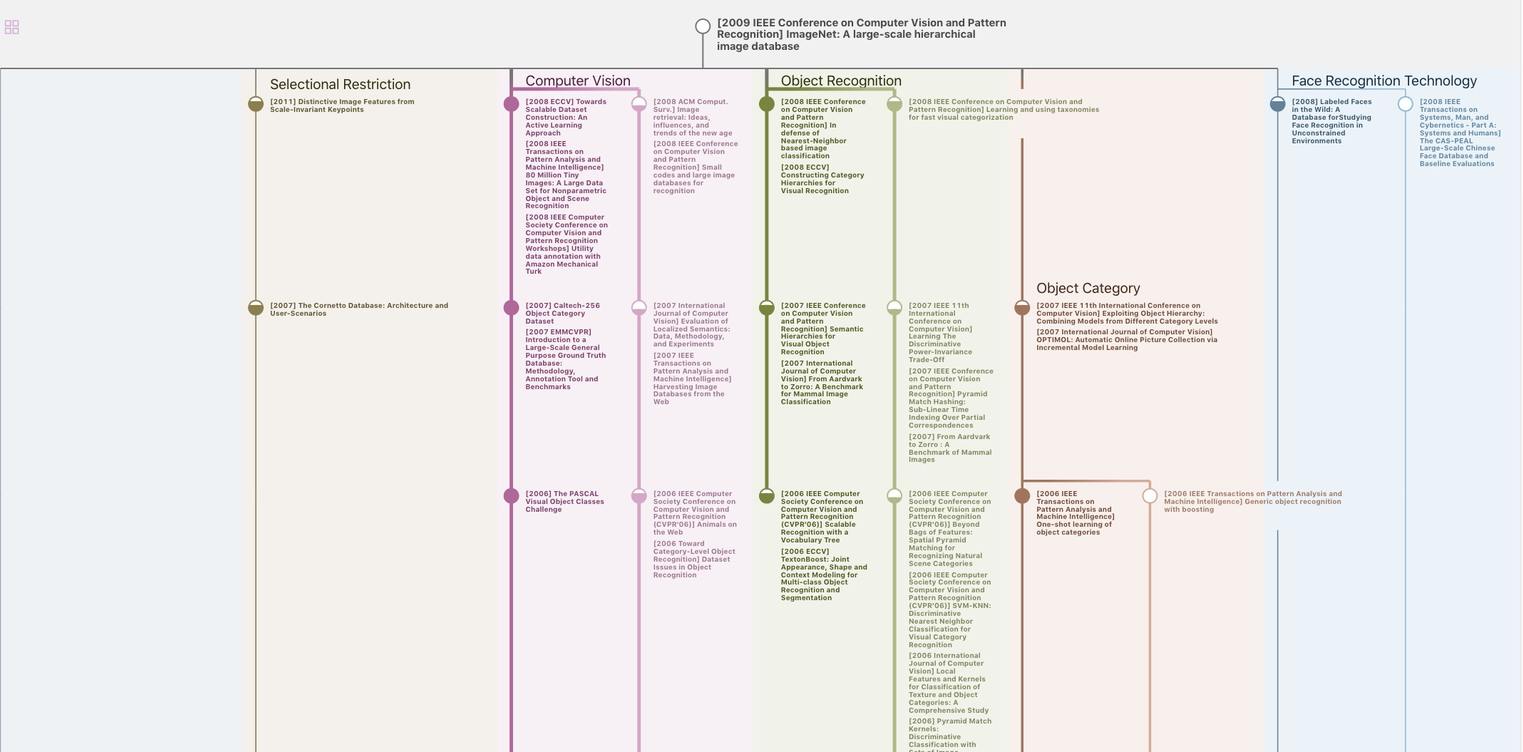
生成溯源树,研究论文发展脉络
Chat Paper
正在生成论文摘要