Deep metric learning with alternating projections onto feasible sets
ICIP(2021)
摘要
Minimizers of the typical distance metric learning loss functions can be considered as "feasible points" satisfying a set of constraints imposed by the training data. We reformulate distance metric learning problem as finding a feasible point of a constraint set where the embedding vectors of the training data satisfy desired intra-class and inter-class proximity. The feasible set induced by the constraint set is expressed as the intersection of the relaxed feasible sets which enforce the proximity constraints only for particular samples (a sample from each class) of the training data. Then, the feasible point problem is to be approximately solved by performing alternating projections onto those feasible sets. Such an approach introduces a regularization term and results in minimizing a typical loss function with a systematic batch set construction where these batches are constrained to contain the same sample from each class for a certain number of iterations. The proposed technique is applied with the well-accepted losses and evaluated on three popular benchmark datasets for image retrieval and clustering. Outperforming state-of-the-art, the proposed approach consistently improves the performance of the integrated loss functions with no additional computational cost.
更多查看译文
关键词
Metric learning,projections
AI 理解论文
溯源树
样例
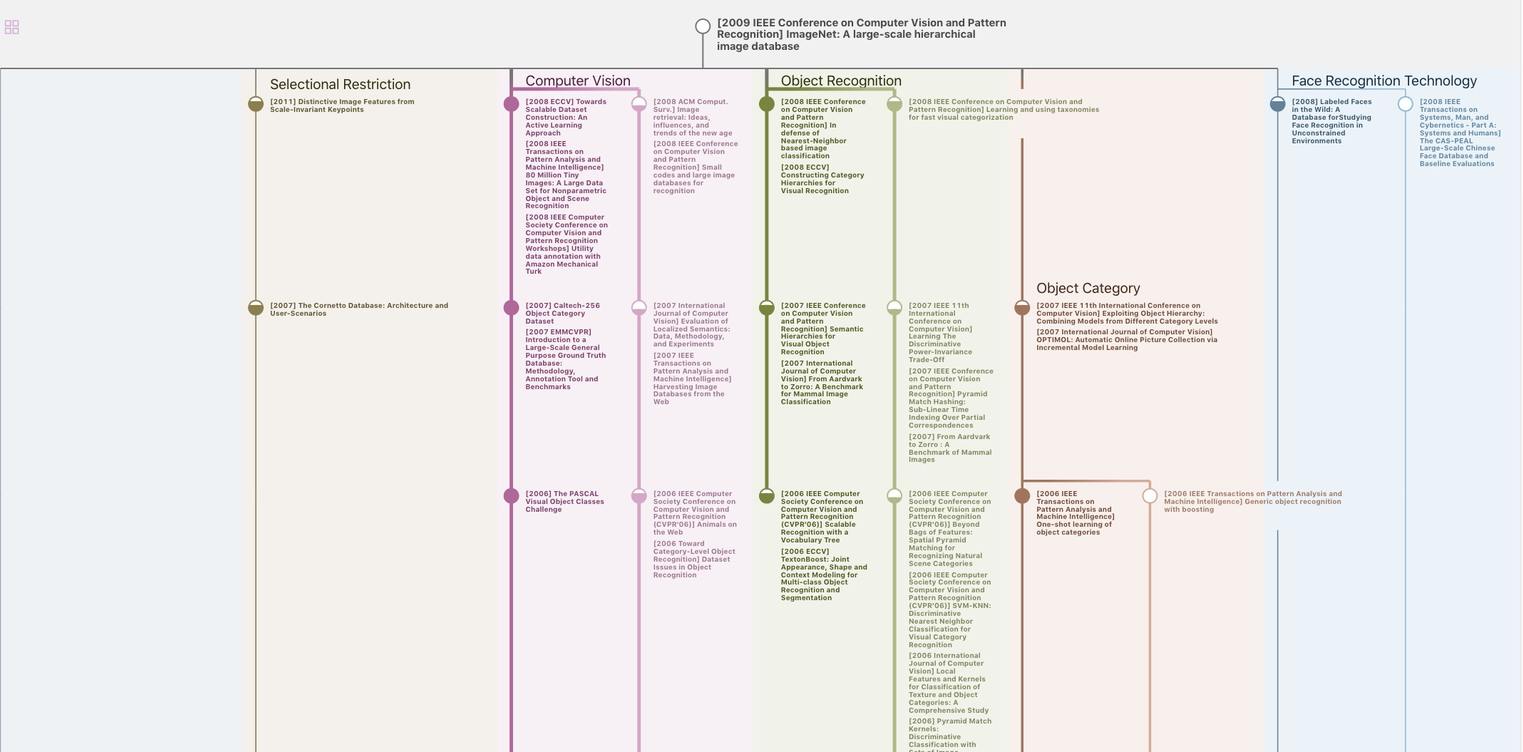
生成溯源树,研究论文发展脉络
Chat Paper
正在生成论文摘要