Speed estimation evaluation on the KITTI benchmark based on motion and monocular depth information
arxiv(2019)
摘要
In this technical report we investigate speed estimation of the ego-vehicle on the KITTI benchmark using state-of-the-art deep neural network based optical flow and single-view depth prediction methods. Using a straightforward intuitive approach and approximating a single scale factor, we evaluate several application schemes of the deep networks and formulate meaningful conclusions such as: combining depth information with optical flow improves speed estimation accuracy as opposed to using optical flow alone; the quality of the deep neural network methods influences speed estimation performance; using the depth and optical flow results from smaller crops of wide images degrades performance. With these observations in mind, we achieve a RMSE of less than 1 m/s for vehicle speed estimation using monocular images as input from recordings of the KITTI benchmark. Limitations and possible future directions are discussed as well.
更多查看译文
AI 理解论文
溯源树
样例
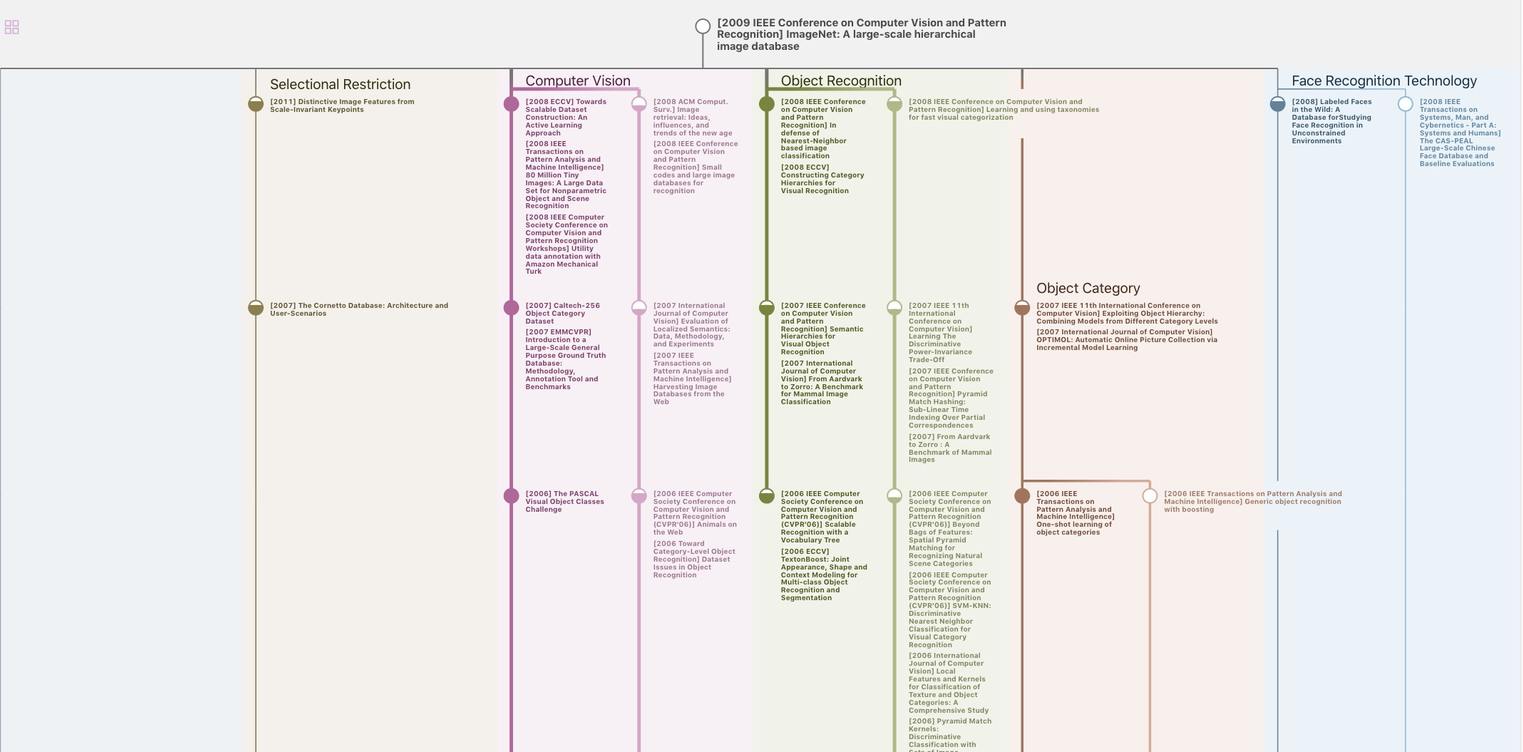
生成溯源树,研究论文发展脉络
Chat Paper
正在生成论文摘要