Quality-aware skill translation models for expert finding on StackOverflow.
Information Systems(2020)
摘要
StackOverflow has become an emerging resource for talent recognition in recent years. While users exploit technical language on StackOverflow, recruiters try to find the relevant candidates for jobs using their own terminology. This procedure implies a gap which exists between recruiters and candidates terms. Due to this gap, the state-of-the-art expert finding models cannot effectively address the expert finding problem on StackOverflow. We propose two translation models to bridge this gap. The first approach is a statistical method and the second is based on word embedding approach. Utilizing several translations for a given query during the scoring step, the result of each intermediate query is blended together to obtain the final ranking. Here, we propose a new approach which takes the quality of documents into account in scoring step. We have made several observations to visualize the effectiveness of the translation approaches and also the quality-aware scoring approach. Our experiments indicate the following: First, while statistical and word embedding translation approaches provide different translations for each query, both can considerably improve the recall. Besides, the quality-aware scoring approach can improve the precision remarkably. Finally, our best proposed method can improve the MAP measure up to 46% on average, in comparison with the state-of-the-art expert finding approach.
更多查看译文
关键词
Expertise retrieval,Statistical machine translation,Semantic matching,StackOverflow,Expert finding,Word embedding
AI 理解论文
溯源树
样例
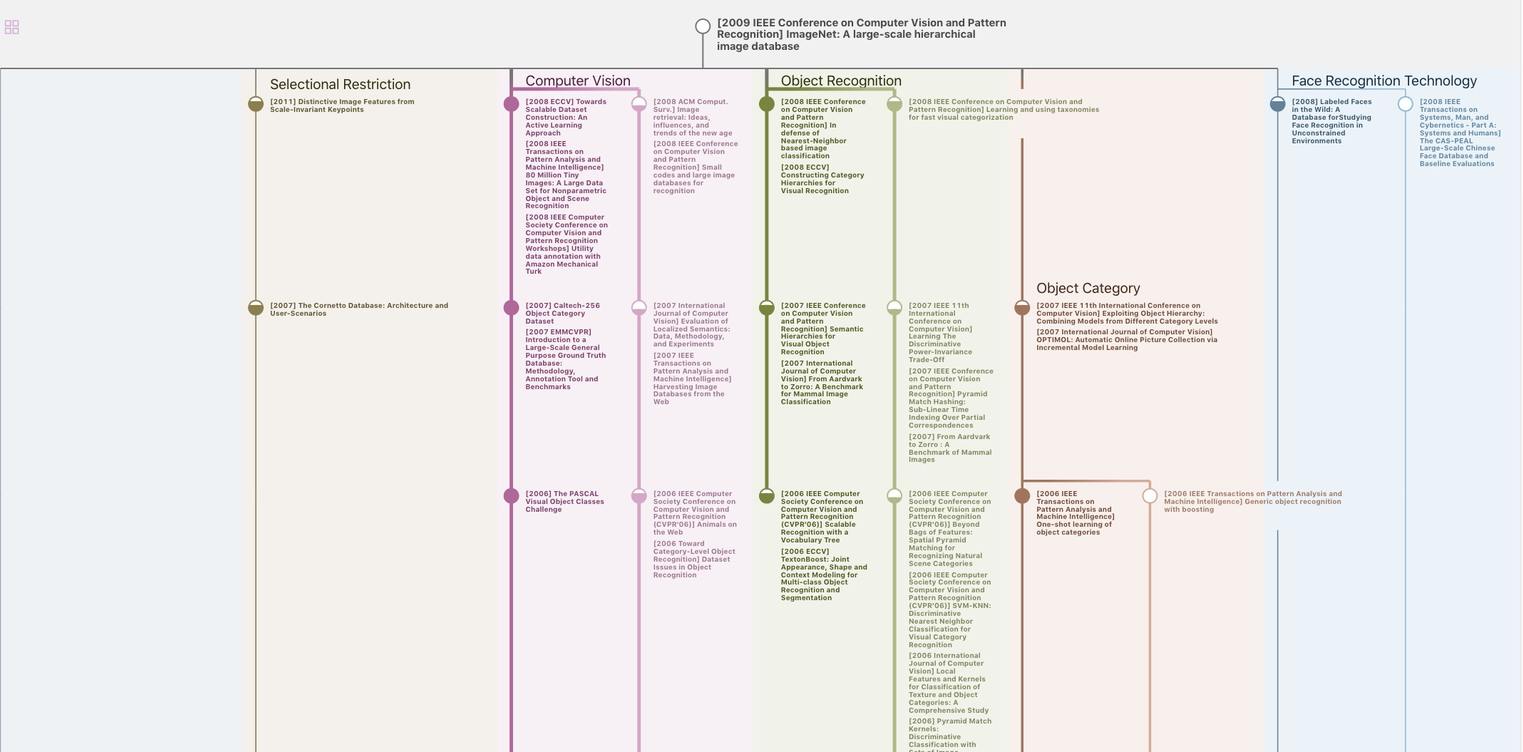
生成溯源树,研究论文发展脉络
Chat Paper
正在生成论文摘要