Stock Volatility Prediction Based on Self-attention Networks with Social Information
2019 IEEE Conference on Computational Intelligence for Financial Engineering & Economics (CIFEr)(2019)
摘要
Stock volatility prediction is a challenging task in time-series prediction according to the Efficient Market Hypothesis which supposes all the investors are rational. However, many theories have showed that stock markets are not efficient due to the effects of psychological and social factors. In this paper, we constructed self-attention networks (SAN) to quantify the impact on the volatility of Chinese stock market of social information, such as social opinion and social concern. Our SAN model can explore the relationships among features at different time steps more flexibly, and thus, explore stock historical information more effectively. Empirical results show the superiority of our model compared to other existing models on given stock data.
更多查看译文
关键词
stock volatility prediction,self-attention networks,social information,time-series prediction,stock markets,psychological factors,social factors,social opinion,social concern,stock historical information,Chinese stock market volatility,stock data,efficient market hypothesis,SAN
AI 理解论文
溯源树
样例
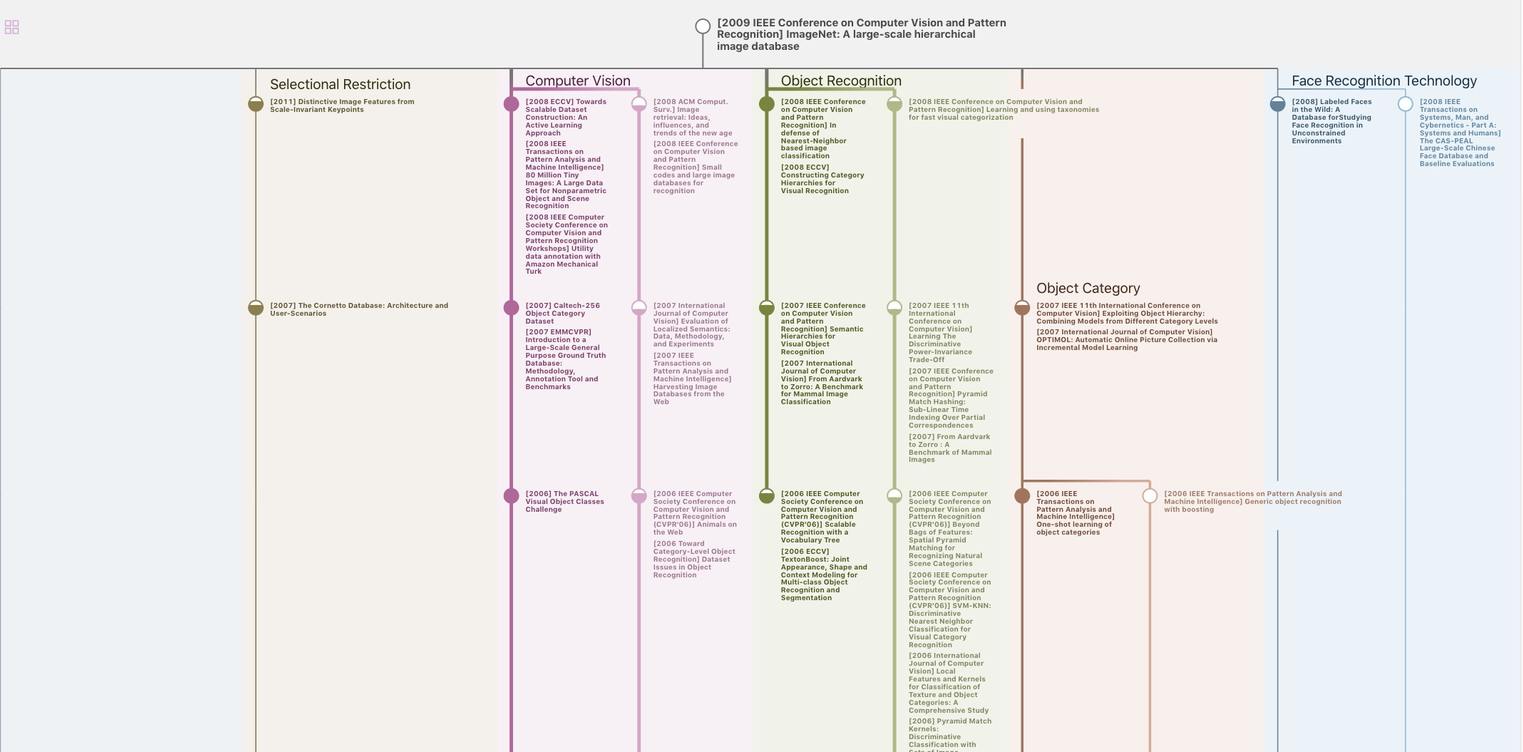
生成溯源树,研究论文发展脉络
Chat Paper
正在生成论文摘要