ACCELERATING FPGA-SURF FEATURE DETECTION MODULE BY MEMORY ACCESS REDUCTION
MALAYSIAN JOURNAL OF COMPUTER SCIENCE(2019)
摘要
Feature detection is an important concept in the area of image processing to compute image abstractions of image information, which is used for image recognition and many other applications. One of the popular algorithm used is called the Speeded-Up Robust Features (SURF), which realized the scale space pyramid to detect the features. For this reason, prior researchers concentrate on applying parallelism onto the SURF multiple layers using technology such as Field Programmable Gate Array (FPGA). However, prior FPGA-SURF implementation does not emphasis on memory access limitation that can affect the overall performance of a system. This paper proposes a study on FPGA-SURF and memory access implementation in feature detection area. We conduct a profiling test and founds that the external memory access to fetch the integral image data in SURF highly affects the overall performance. We also found that the SURF algorithm memory access has redundant repeating pattern that can be reduced. Therefore, a controller design that stores repeating data (for the subsequent process) in an on-chip memory is proposed. This method reduces the external memory access and can increase the overall performance. The result shows that our proposed method improves the existing method (i.e. without the memory access reduction) by 1.23 times when the external memory latency is 20ns.
更多查看译文
关键词
SURF,FPGA,Feature Detection,Memory Access,Fast Hessian
AI 理解论文
溯源树
样例
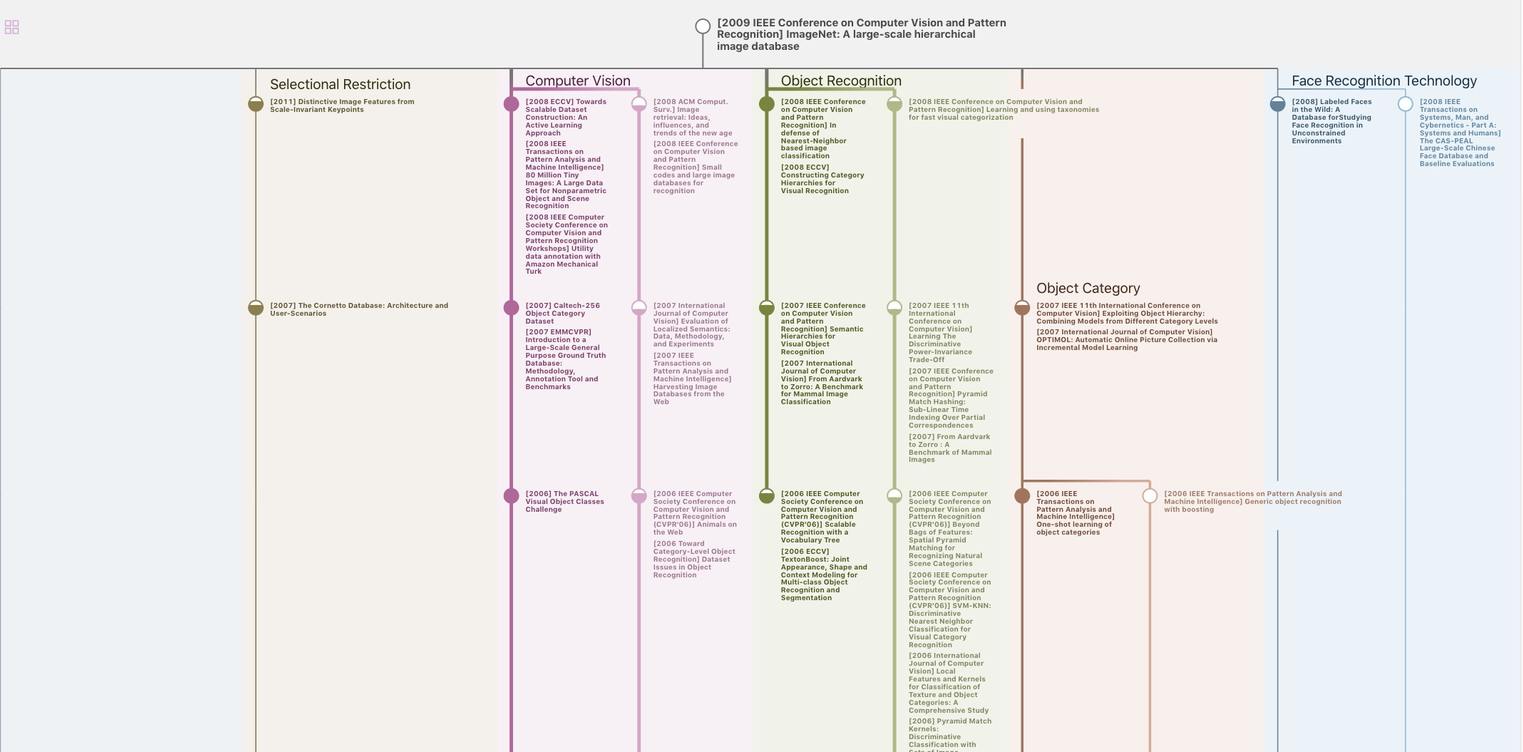
生成溯源树,研究论文发展脉络
Chat Paper
正在生成论文摘要