Region-wise Modeling of Facial Skin Age using Deep CNNs
2019 16th International Conference on Machine Vision Applications (MVA)(2019)
摘要
We propose a deep learning approach for predicting the apparent age of a person's skin. Our method works by first normalizing a frontal image of a face and cropping rectangular-shaped skin patches that are each normalized and fed into separately trained region-specific CNNs. Each regional CNN model is fine tuned using a novel data augmentation technique that artificially reduces the apparent age of the skin through a series of smoothing operations that act as a proxy for subjects with younger looking skin. The deep features extracted from each of these regions are then used to train a separate set of regression models that predict the skin age. We evaluate our method using two strategies: the first looks at how well the predicted regional skin age clusters around the true biological age of the subject, for which we achieve a 1-off accuracy of approximately 83%. In the second strategy, we validate that our models predict apparent skin age based on a user study that asked over 15 judges to compare image pairs of subjects with the same chronological age, but with different skin age predictions. For this second study, we achieve an average 66% accuracy based on consensus rating across all human raters, and as high as 76% for some age groups.
更多查看译文
关键词
data augmentation technique,skin age predictions,trained region-specific CNNs,trained region-specific CNNs,age groups,chronological age,apparent skin age,biological age,predicted regional skin age clusters,regression models,deep features,younger looking skin,regional CNN model,cropping rectangular-shaped skin patches,frontal image,apparent age,deep learning approach,deep CNNs,facial skin age,region-wise modeling
AI 理解论文
溯源树
样例
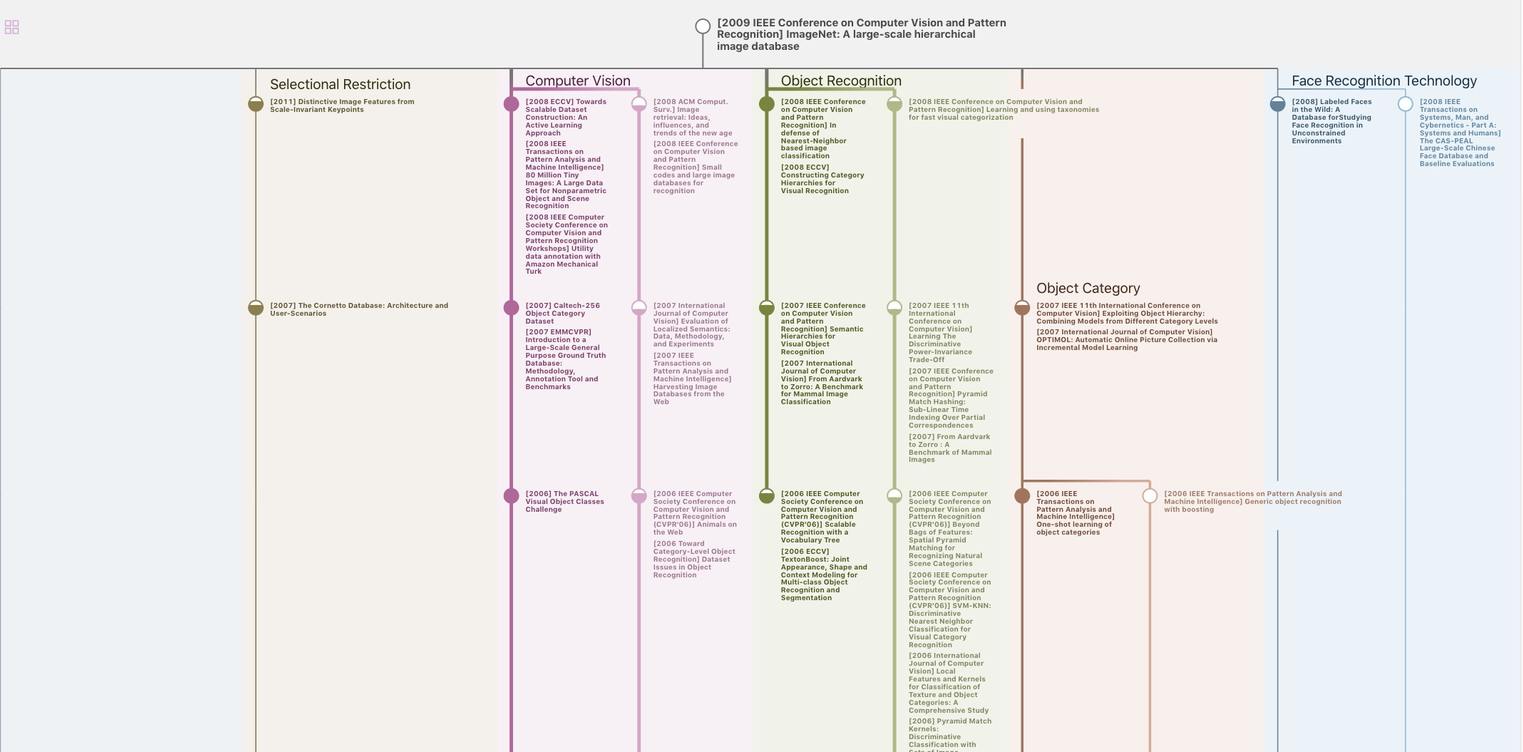
生成溯源树,研究论文发展脉络
Chat Paper
正在生成论文摘要