Differential evolution for multi-modal multi-objective problems.
GECCO(2019)
摘要
Multi-modal multi-objective problems (MMMOPs) have two or more distinct Pareto-optimal sets (PSs) mapping to the same Pareto-front (PF). Identifying all such PSs assists in informed decision-making. However, existing multi-objective evolutionary algorithms are not equipped to discover multiple PSs. Recently, a few studies have been conducted to design algorithms for such MMMOPs. However, the diversity of the solutions in the PF, obtained by these algorithms, are poor. Moreover, two effective strategies, identified to address MMMOPs, are niching methods and population filtering, based on convergence and diversity of solutions in PF along with diversity of solutions in PS. Motivated by these requirements, this study presents Differential Evolution for MMMOPs (DE-TriM). Its novel contributions include mating pool selection strategy and resource allocation scheme based on reference vector based decomposition of objective space. The effectiveness of DE-TriM is validated by its performance analysis on 11 benchmark MMMOPs in terms of four performance measures as compared to three recent optimization algorithms. The results demonstrate similar performance of DE-TriM in decision space and its superior performance in objective space as compared to the state-of-the-art multi-modal multi-objective evolutionary algorithm.
更多查看译文
关键词
Multi-Modal Multi-Objective Problems, Pareto-dominance, Reference Vector based Decomposition, Decision Space, Objective Space
AI 理解论文
溯源树
样例
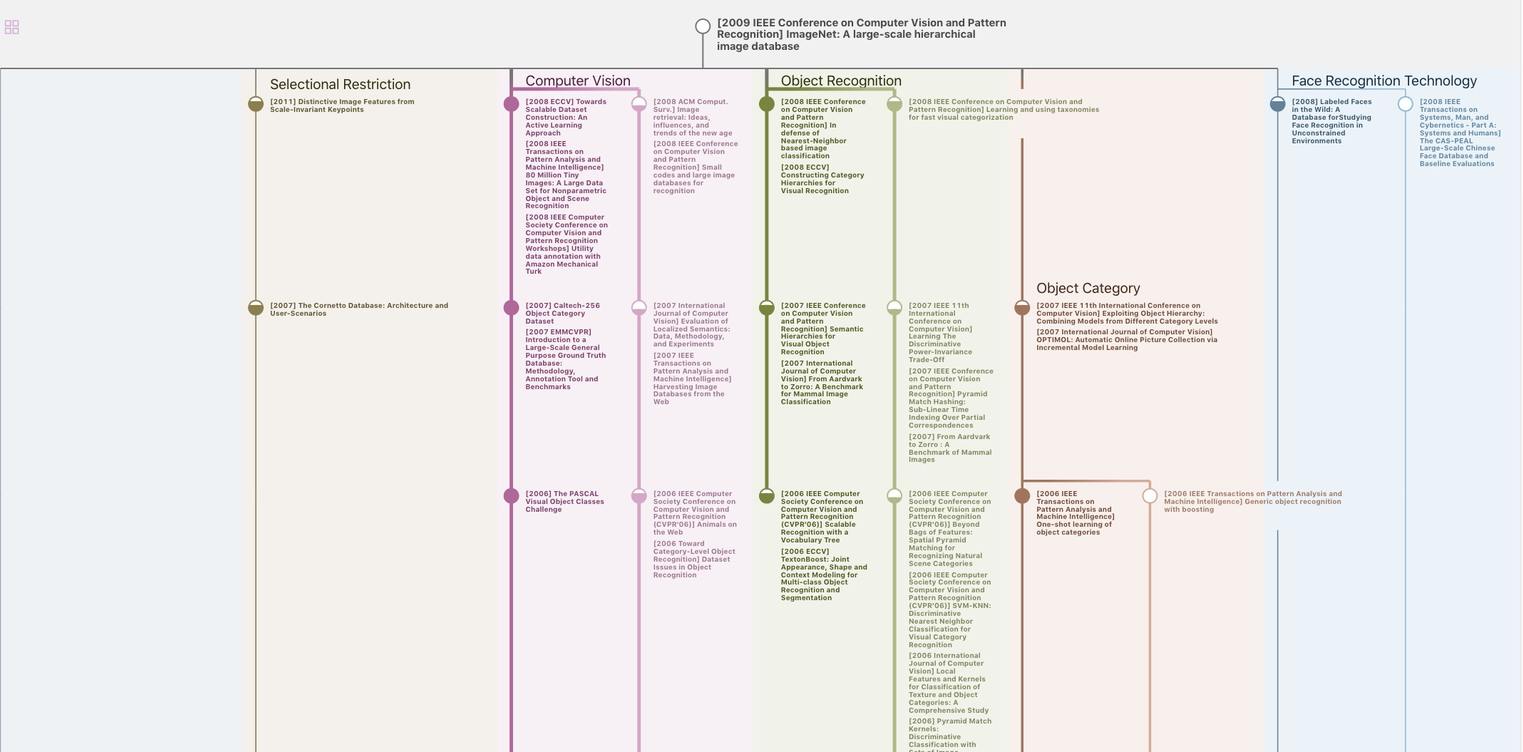
生成溯源树,研究论文发展脉络
Chat Paper
正在生成论文摘要