Worst case search over a set of forecasting scenarios applied to financial stress-testing.
GECCO(2019)
摘要
Stress testing is part of today's bank risk management and often required by the governing regulatory authority. Performing such a stress test with stress scenarios derived from a distribution, instead of pre-defined expert scenarios, results in a systematic approach in which new severe scenarios can be discovered. The required scenario distribution is obtained from historical time series via a Vector-Autoregressive time series model.
The worst-case search, i.e. finding the scenario yielding the most severe situation for the bank, can be stated as an optimization problem. The problem itself is a constrained optimization problem in a high-dimensional search space. The constraints are the box constraints on the scenario variables and the plausibility of a scenario. The latter is expressed by an elliptic constraint.
As the evaluation of the stress scenarios is performed with a simulation tool, the optimization problem can be seen as black-box optimization problem. Evolution Strategy, a well-known optimizer for black-box problems, is applied here. The necessary adaptations to the algorithm are explained and a set of different algorithm design choices are investigated. It is shown that a simple box constraint handling method, i.e. setting variables which violate a box constraint to the respective boundary of the feasible domain, in combination with a repair of implausible scenarios provides good results.
更多查看译文
关键词
risk management, simulation-based optimization, high-dimensional search space, constrained optimization, stress-testing
AI 理解论文
溯源树
样例
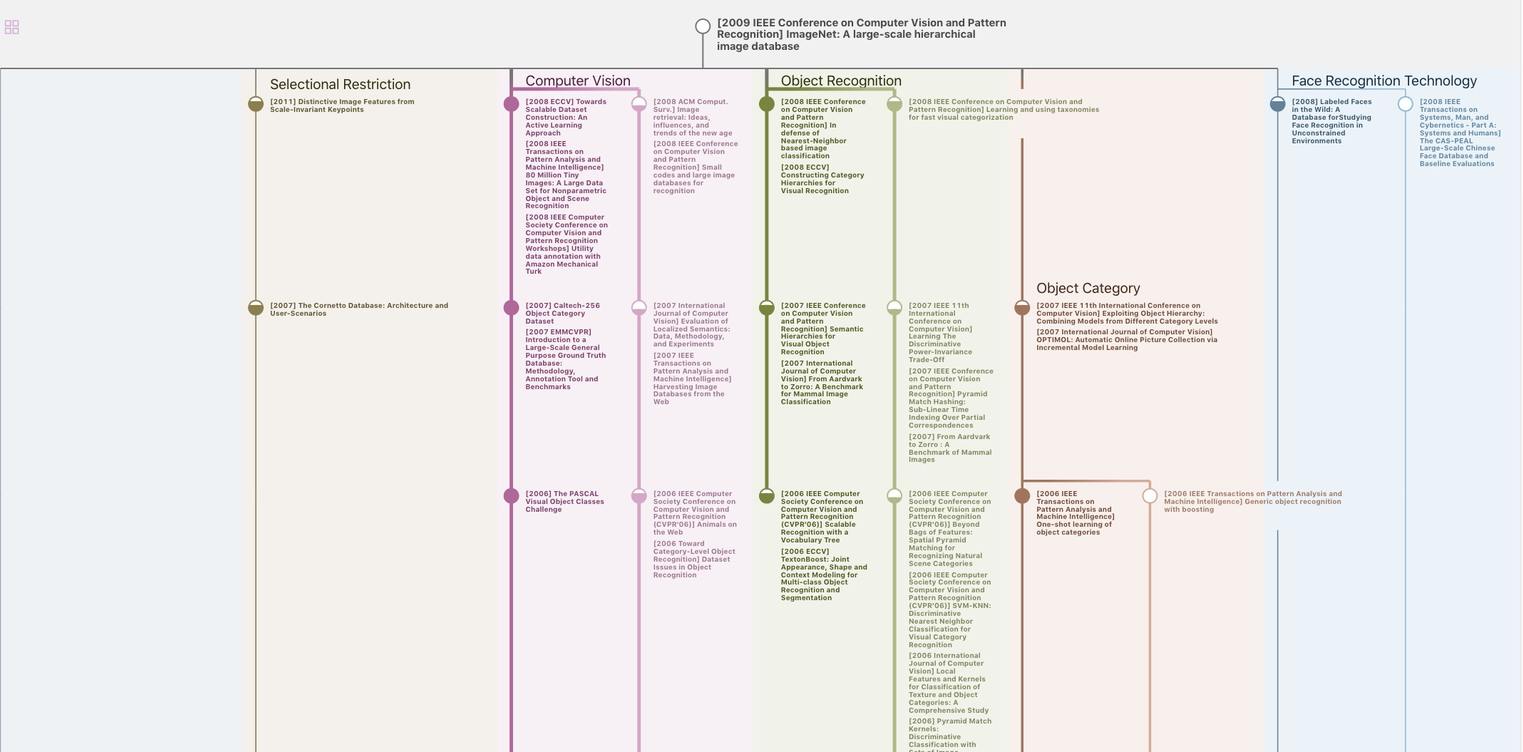
生成溯源树,研究论文发展脉络
Chat Paper
正在生成论文摘要