Regression with Support Vector Machines and VGG Neural Networks.
AMLTA(2019)
Abstract
In the area of machine learning, classification tasks have been well studied, while another important application of regression is not of the same level. In this paper, we propose parameterization and settings obtained from multiple experiments for traditional supervised machine learning of Support Vector Machine (SVM) and recently widely used deep unsupervised learning technology of Convolutional Neural Networks (CNN) based on Visual Geometry Group (VGGNet). In this study, different dataset used for regression task have been adopted. We have experimented on six data types obtained from the UCI Machine Learning Repository, and one converted handwritten image dataset from the MNIST. Accuracy of the regression results generated by the proposed models are validated with statistical methods of Mean Absolute Error (MAE) and R_square, i.e. coefficient of determination. Experimental results demonstrate that VGG has clear advantages over SVM in the cases of image recognition and attributes with strong correlation, and SVM performs better in the cases of discrete, irregular and weak correlation data than. By comparing the three kernel functions of SVM, it is found that in most cases, Rbt kernel function performs more effectively than Linear and Poly ones.
MoreTranslated text
Key words
Regression, VGG, SVM, R_square, Kernel function, Supervised and unsupervised learning
AI Read Science
Must-Reading Tree
Example
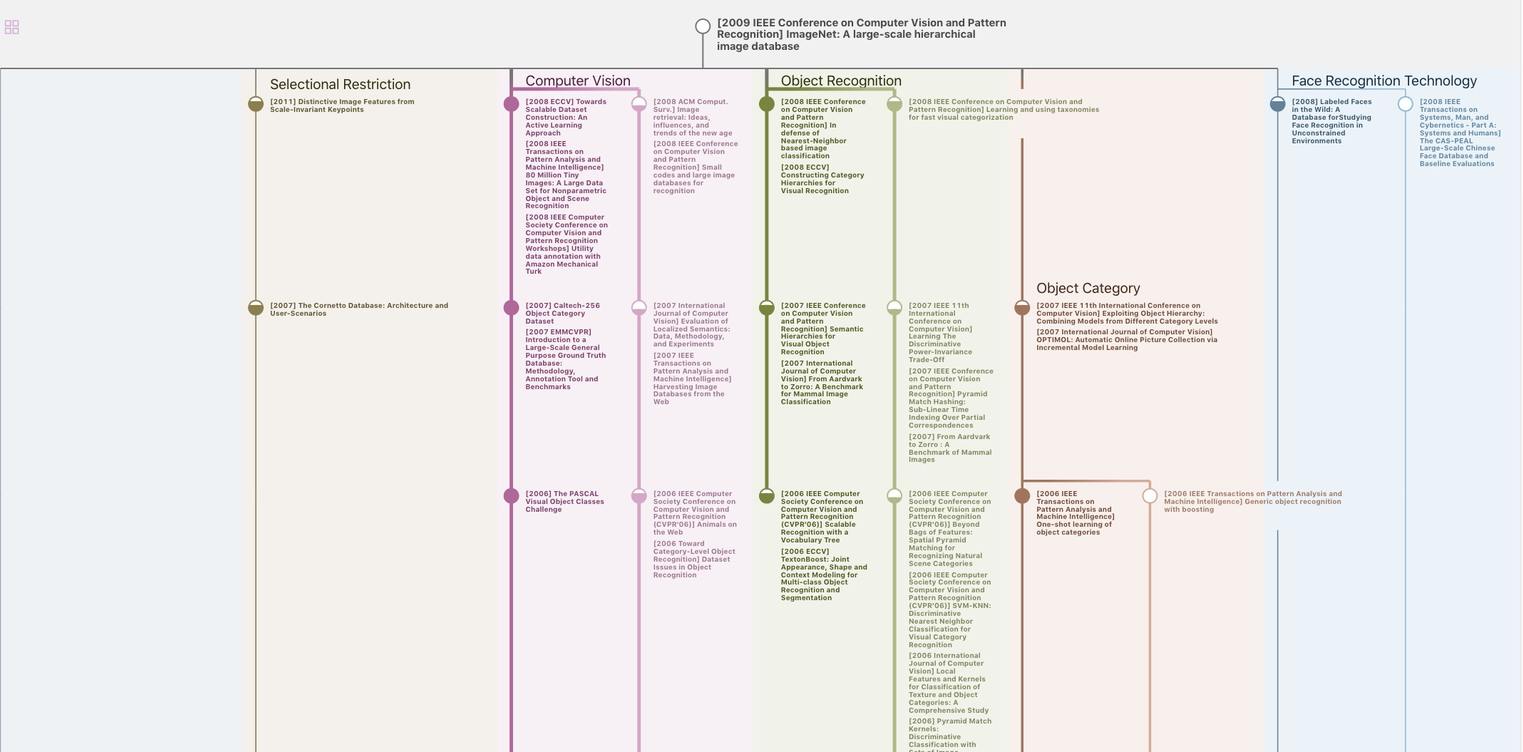
Generate MRT to find the research sequence of this paper
Chat Paper
Summary is being generated by the instructions you defined