Transformer-Based No-Reference Image Quality Assessment via Supervised Contrastive Learning
AAAI 2024(2024)
摘要
Image Quality Assessment (IQA) has long been a research hotspot in the field of image processing, especially No-Reference Image Quality Assessment (NR-IQA). Due to the powerful feature extraction ability, existing Convolution Neural Network (CNN) and Transformers based NR-IQA methods have achieved considerable progress. However, they still exhibit limited capability when facing unknown authentic distortion datasets. To further improve NR-IQA performance, in this paper, a novel supervised contrastive learning (SCL) and Transformer-based NR-IQA model SaTQA is proposed. We first train a model on a large-scale synthetic dataset by SCL (no image subjective score is required) to extract degradation features of images with various distortion types and levels. To further extract distortion information from images, we propose a backbone network incorporating the Multi-Stream Block (MSB) by combining the CNN inductive bias and Transformer long-term dependence modeling capability. Finally, we propose the Patch Attention Block (PAB) to obtain the final distorted image quality score by fusing the degradation features learned from contrastive learning with the perceptual distortion information extracted by the backbone network. Experimental results on six standard IQA datasets show that SaTQA outperforms the state-of-the-art methods for both synthetic and authentic datasets. Code is available at https://github.com/I2-Multimedia-Lab/SaTQA.
更多查看译文
关键词
CV: Low Level & Physics-based Vision,CV: Applications
AI 理解论文
溯源树
样例
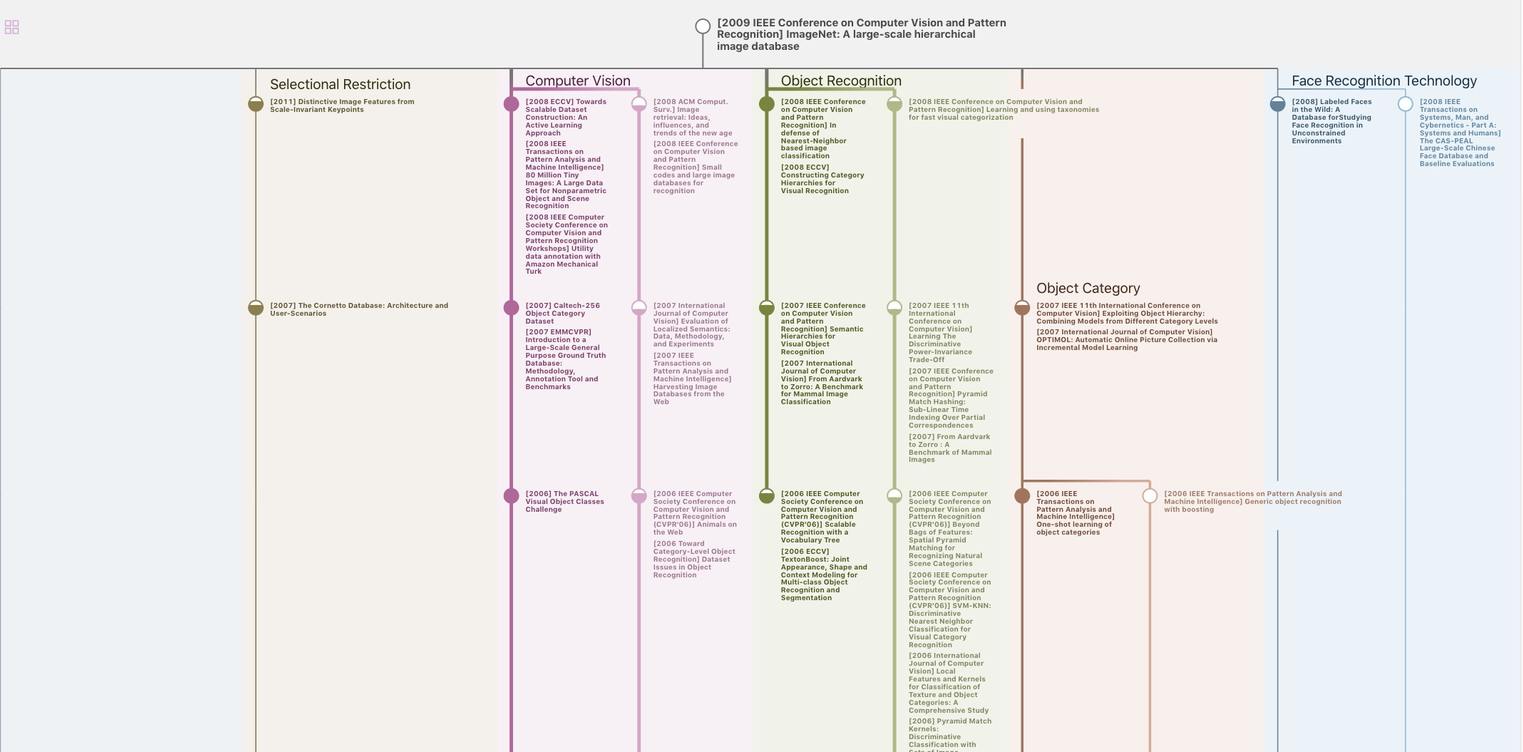
生成溯源树,研究论文发展脉络
Chat Paper
正在生成论文摘要