Radiomics And Machine Learning Of Multisequence Multiparametric Prostate Mri: Towards Improved Non-Invasive Prostate Cancer Characterization
PLOS ONE(2019)
摘要
PurposeTo develop and validate a classifier system for prediction of prostate cancer (PCa) Gleason score (GS) using radiomics and texture features of T-2-weighted imaging (T(2)w), diffusion weighted imaging (DWI) acquired using high b values, and T-2-mapping (T-2).MethodsT(2)w, DWI (12 b values, 0-2000 s/mm(2)), and T-2 data sets of 62 patients with histologically confirmed PCa were acquired at 3T using surface array coils. The DWI data sets were post processed using monoexponential and kurtosis models, while T(2)w was standardized to a common scale. Local statistics and 8 different radiomics/texture descriptors were utilized at different configurations to extract a total of 7105 unique per-tumor features. Regularized logistic regression with implicit feature selection and leave pair out cross validation was used to discriminate tumors with 3+3 vs >3+3 GS.ResultsIn total, 100 PCa lesions were analysed, of those 20 and 80 had GS of 3+3 and >3+3, respectively. The best model performance was obtained by selecting the top 1% features of T(2)w, ADC(m) and K with ROC AUC of 0.88 (95% CI of 0.82-0.95). Features from T-2 mapping provided little added value. The most useful texture features were based on the gray-level co-occurrence matrix, Gabor transform, and Zernike moments.ConclusionTexture feature analysis of DWI, post-processed using monoexponential and kurtosis models, and T(2)w demonstrated good classification performance for GS of PCa. In multisequence setting, the optimal radiomics based texture extraction methods and parameters differed between different image types.
更多查看译文
关键词
multiparametric prostate mri,prostate cancer,machine learning,non-invasive
AI 理解论文
溯源树
样例
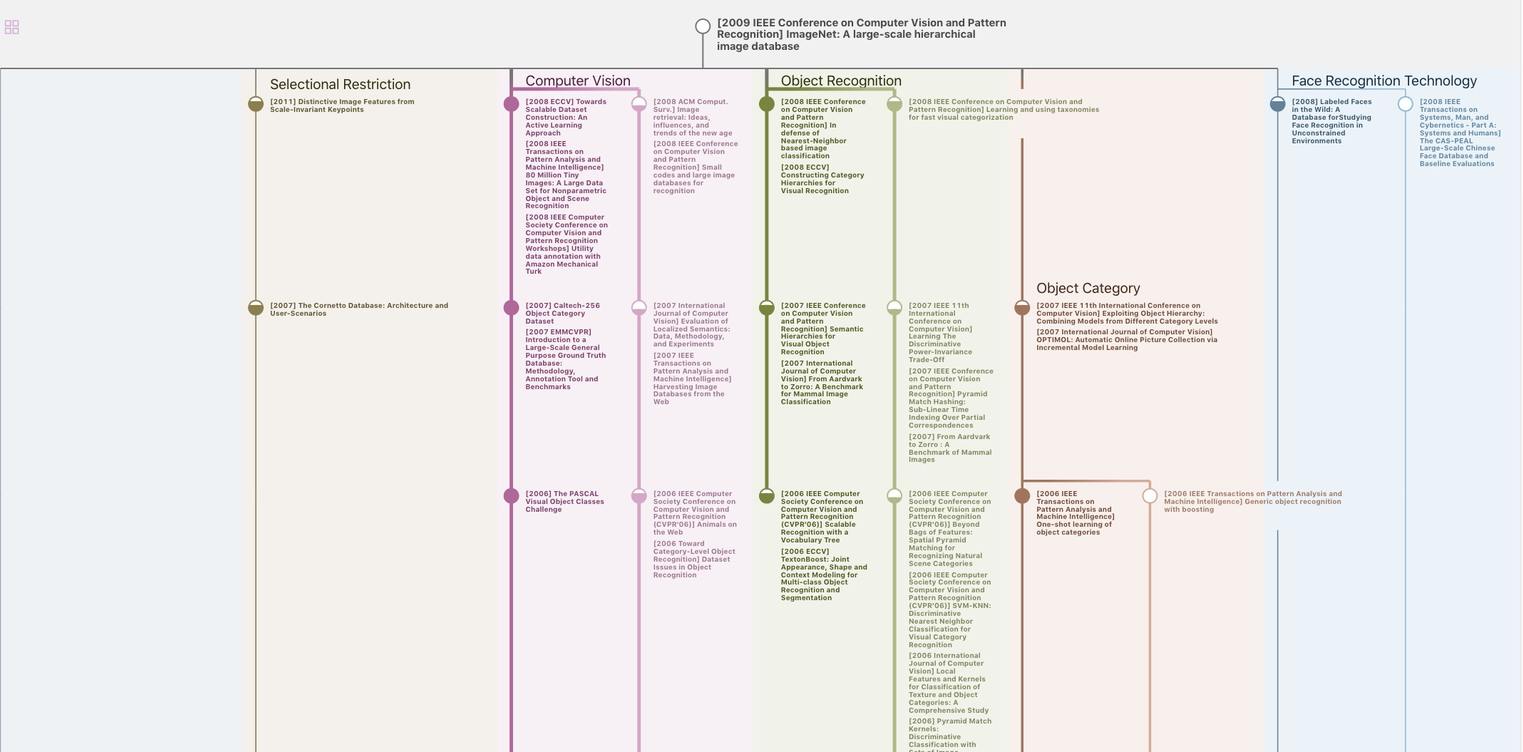
生成溯源树,研究论文发展脉络
Chat Paper
正在生成论文摘要