Improving Lasso for model selection and prediction
SCANDINAVIAN JOURNAL OF STATISTICS(2022)
Abstract
It is known that the Thresholded Lasso (TL), SCAD or MCP correct intrinsic estimation bias of the Lasso. In this paper we propose an alternative method of improving the Lasso for predictive models with general convex loss functions which encompass normal linear models, logistic regression, quantile regression, or support vector machines. For a given penalty we order the absolute values of the Lasso nonzero coefficients and then select the final model from a small nested family by the Generalized Information Criterion. We derive exponential upper bounds on the selection error of the method. These results confirm that, at least for normal linear models, our algorithm seems to be the benchmark for the theory of model selection as it is constructive, computationally efficient and leads to consistent model selection under weak assumptions. Constructivity of the algorithm means that, in contrast to the TL, SCAD or MCP, consistent selection does not rely on the unknown parameters as the cone invertibility factor. Instead, our algorithm only needs the sample size, the number of predictors and an upper bound on the noise parameter. We show in numerical experiments on synthetic and real-world datasets that an implementation of our algorithm is more accurate than implementations of studied concave regularizations. Our procedure is included in the R package DMRnet and available in the CRAN repository.
MoreTranslated text
Key words
convex loss function, empirical process, generalized information criterion, high-dimensional regression, penalized estimation, selection consistency
AI Read Science
Must-Reading Tree
Example
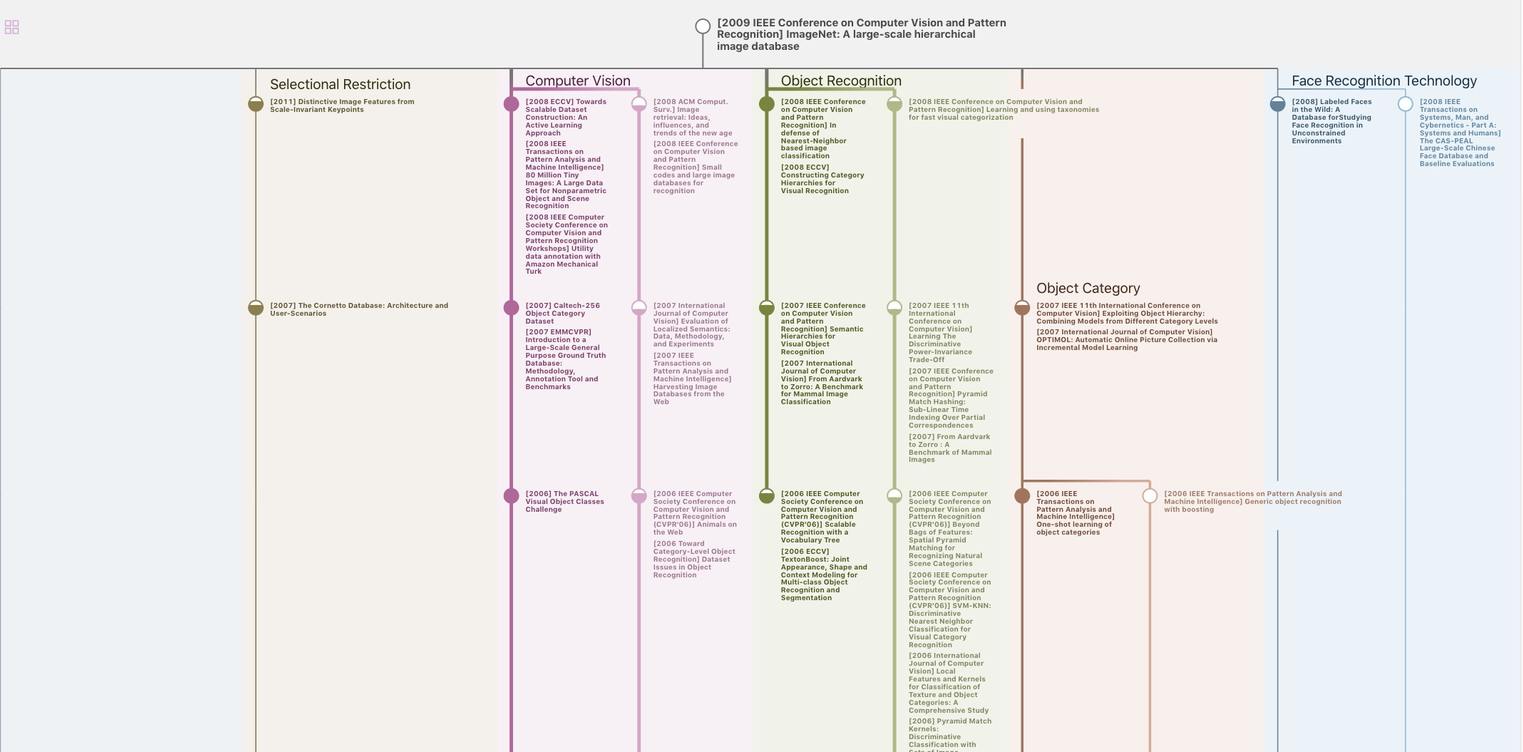
Generate MRT to find the research sequence of this paper
Chat Paper
Summary is being generated by the instructions you defined