Tiny People Pose
COMPUTER VISION - ACCV 2018, PT III(2018)
摘要
While recent progress in pose recognition has been impressive, there remains ample margin for improvement, particularly in challenging scenarios such as low resolution images. In this paper, we consider the problem of recognizing pose from tiny images of people, down to 24px high. This is relevant when interpreting people at a distance, which is important in applications such as autonomous driving and surveillance in crowds. Addressing this challenge, which has received little attention so far, can inspire modifications of traditional deep learning approaches that are likely to be applicable well beyond the case of pose recognition.Given the intrinsic ambiguity of recovering a person's pose from a small image, we propose to predict a posterior probability over pose configurations. In order to do so we: (1) define a new neural network architecture that explicitly expresses uncertainty; (2) train the network by explicitly minimizing a novel loss function based on the data log-likelihood; and (3) estimate posterior probability maps for all joints as a semi-dense sub-pixel Gaussian mixture model. We asses our method on downsampled versions of popular pose recognition benchmarks as well as on an additional newly-introduced testing dataset. Compared to state-of-the-art techniques, we show far superior performance at low resolution for both deterministic and probabilistic pose prediction.
更多查看译文
AI 理解论文
溯源树
样例
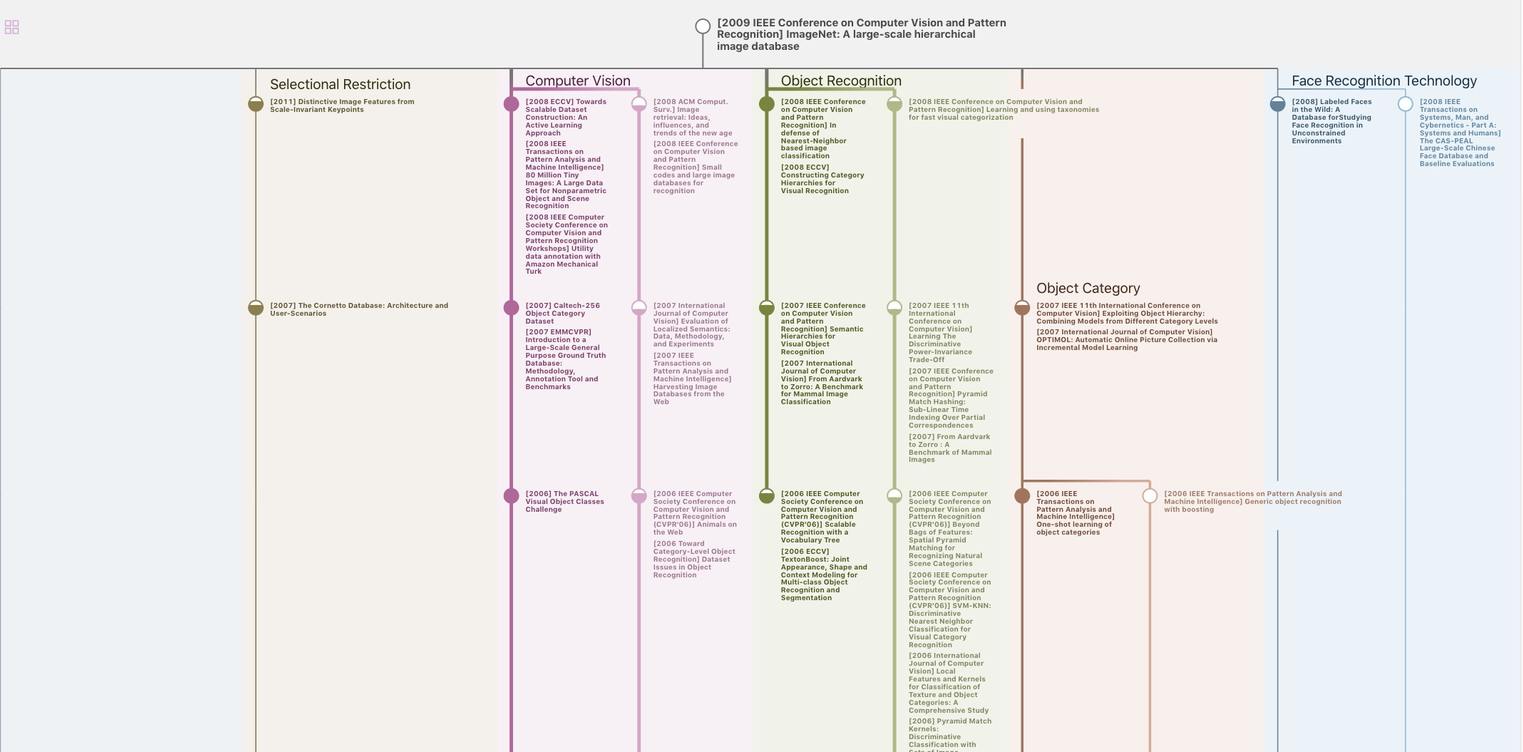
生成溯源树,研究论文发展脉络
Chat Paper
正在生成论文摘要