Asymmetric Deep Cross-Modal Hashing
COMPUTATIONAL SCIENCE - ICCS 2019, PT V(2019)
摘要
Cross-modal retrieval has attracted increasing attention in recent years. Deep supervised hashing methods have been widely used for cross-modal similarity retrieval on large-scale datasets, because the deep architectures can generate more discriminative feature representations. Traditional hash methods adopt a symmetric way to learn the hash function for both query points and database points. However, those methods take an immense amount of work and time for model training, which is inefficient with the explosive growth of data volume. To solve this issue, an Asymmetric Deep Cross-modal Hashing (ADCH) method is proposed to perform more effective hash learning by simultaneously preserving the semantic similarity and the underlying data structures. More specifically, ADCH treats the query points and database points in an asymmetric way. Furthermore, to provide more similarity information, a detailed definition for cross-modal similarity matrix is also proposed. The training of ADCH takes less time than traditional symmetric deep supervised hashing methods. Extensive experiments on two widely used datasets show that the proposed approach achieves the state-of-the-art performance in cross-modal retrieval.
更多查看译文
关键词
Asymmetric hashing, Cross-modal, Retrieval
AI 理解论文
溯源树
样例
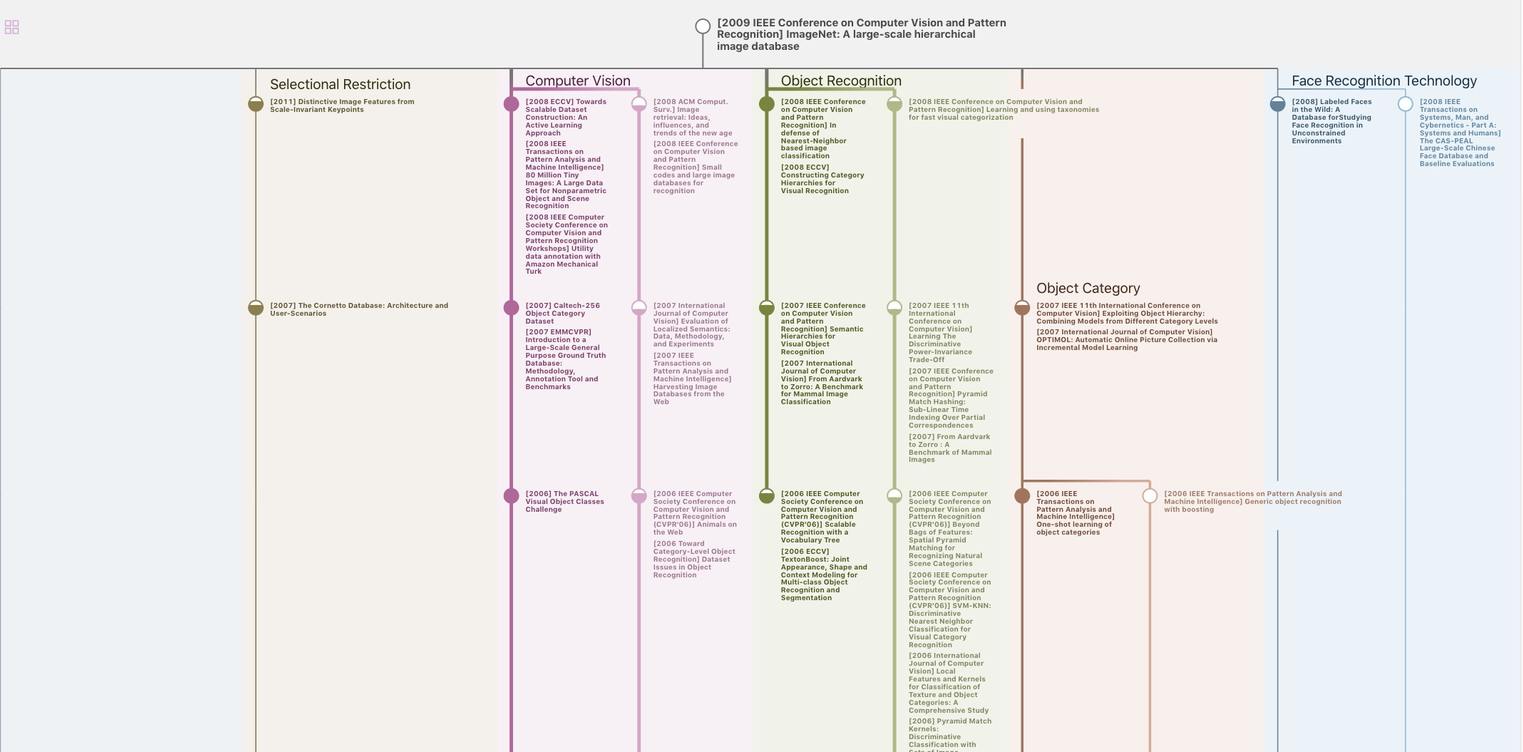
生成溯源树,研究论文发展脉络
Chat Paper
正在生成论文摘要