Machine Learning-Based Lithium-Ion Battery Capacity Estimation Exploiting Multi-Channel Charging Profiles.
IEEE ACCESS(2019)
摘要
Prognostics and health management is a promising methodology to cope with the risks of failure in advance and has been implemented in many well-known applications including battery systems. Since the estimation of battery capacity is critical for safe operation and decision making, battery capacity should be estimated precisely. In this regard, we leverage measurable data such as voltage, current, and temperature profiles from the battery management system whose patterns vary in cycles as aging. Based on these data, the relationship between capacity and charging profiles is learned by neural networks. Specifically, to estimate the state of health accurately we apply feedforward neural network, convolutional neural network, and long short-term memory. Our results show that the proposed multi-channel technique based on voltage, current, and temperature profiles outperforms the conventional method that uses only voltage profile by up to 25%-58% in terms of mean absolute percentage error.
更多查看译文
关键词
Lithium-ion battery,neural network,remaining useful life,capacity estimation,state of health
AI 理解论文
溯源树
样例
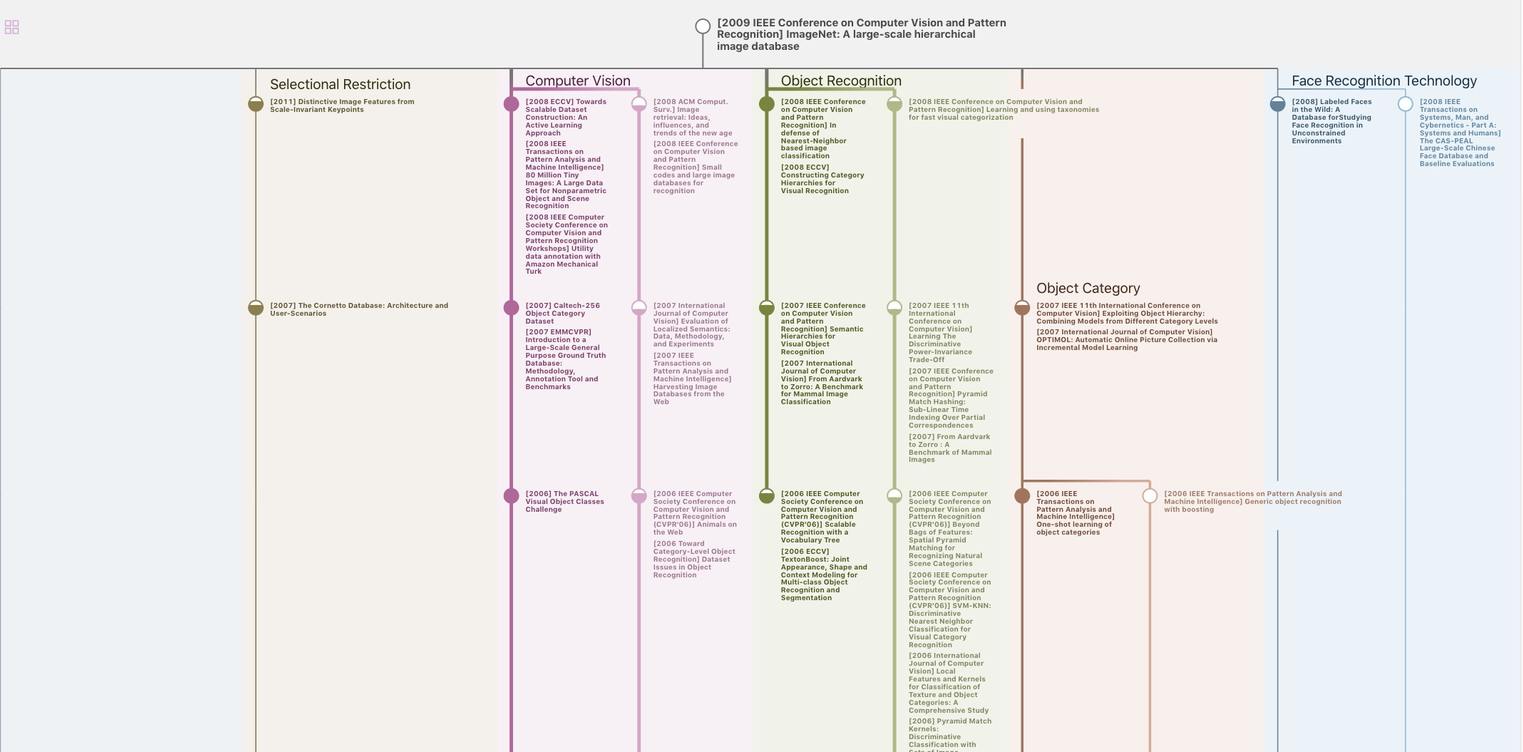
生成溯源树,研究论文发展脉络
Chat Paper
正在生成论文摘要