Thompson Sampling Based Active Learning in Probabilistic Programs with Application to Travel Time Estimation.
ADVANCES AND TRENDS IN ARTIFICIAL INTELLIGENCE: FROM THEORY TO PRACTICE(2019)
摘要
The pertinent problem of Traveling Time Estimation (TTE) is to estimate the travel time, given a start location and a destination, solely based on the coordinates of the points under consideration. This is typically solved by fitting a function based on a sequence of observations. However, it can be expensive or slow to obtain labeled data or measurements to calibrate the estimation function. Active Learning tries to alleviate this problem by actively selecting samples that minimize the total number of samples needed to do accurate inference. Probabilistic Programming Languages (PPL) give us the opportunities to apply powerful Bayesian inference to model problems that involve uncertainties. In this paper we combine Thompson Sampling with Probabilistic Programming to perform Active Learning in the Travel Time Estimation setting, outperforming traditional active learning methods.
更多查看译文
关键词
Thompson Sampling,Distance Estimation,Probabilistic Programming,Active Learning,Travel Time Estimation
AI 理解论文
溯源树
样例
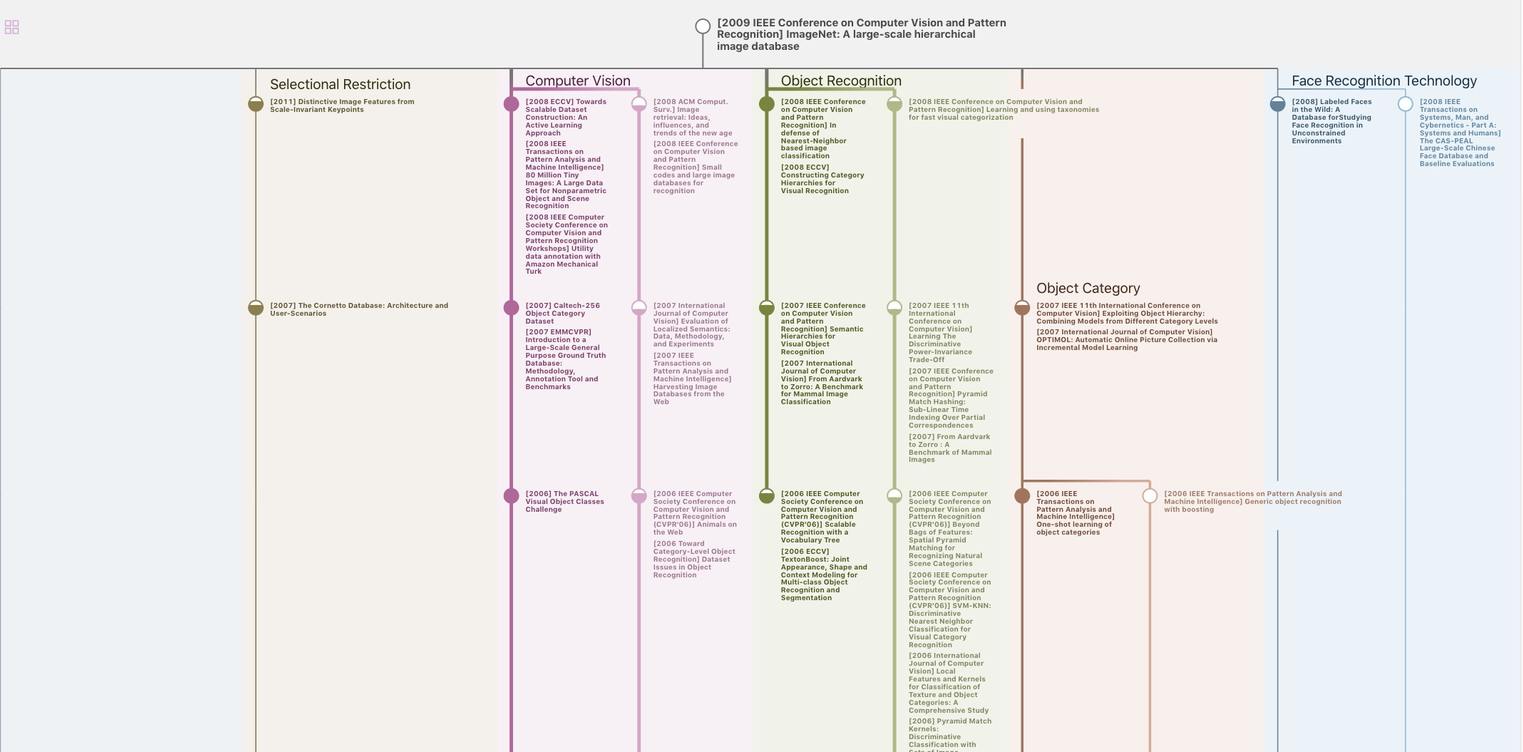
生成溯源树,研究论文发展脉络
Chat Paper
正在生成论文摘要