"Machine Llrning": Learning To Softly Demodulate
2019 IEEE GLOBECOM WORKSHOPS (GC WKSHPS)(2019)
摘要
Soft demodulation, or demapping, of received symbols back into their conveyed soft bits, or bit log-likelihood ratios (LLRs), is at the very heart of any modern receiver. In this paper, a trainable universal neural network-based demodulator architecture, dubbed "LLRnet", is introduced. LLRnet facilitates an improved performance with significantly reduced overall computational complexity. For instance for the commonly used quadrature amplitude modulation (QAM), LLRnet demonstrates LLR estimates approaching the optimal log maximum aposteriori inference with an order of magnitude less operations than that of the straightforward exact implementation. Link-level simulation examples for the application of LLRnet to 5G-NR and DVB-S.2 are provided. LLRnet is a (yet another) powerful example for the usefulness of applying machine learning to physical layer design.
更多查看译文
关键词
machine learning,link-level simulation examples,optimal log maximum a-posteriori inference,LLR estimates,commonly used quadrature amplitude modulation,reduced overall computational complexity,LLRnet,trainable universal neural network-based,modern receiver,LLRs,log-likelihood ratios,conveyed soft bits,received symbols,demapping,soft demodulation,machine LLRning
AI 理解论文
溯源树
样例
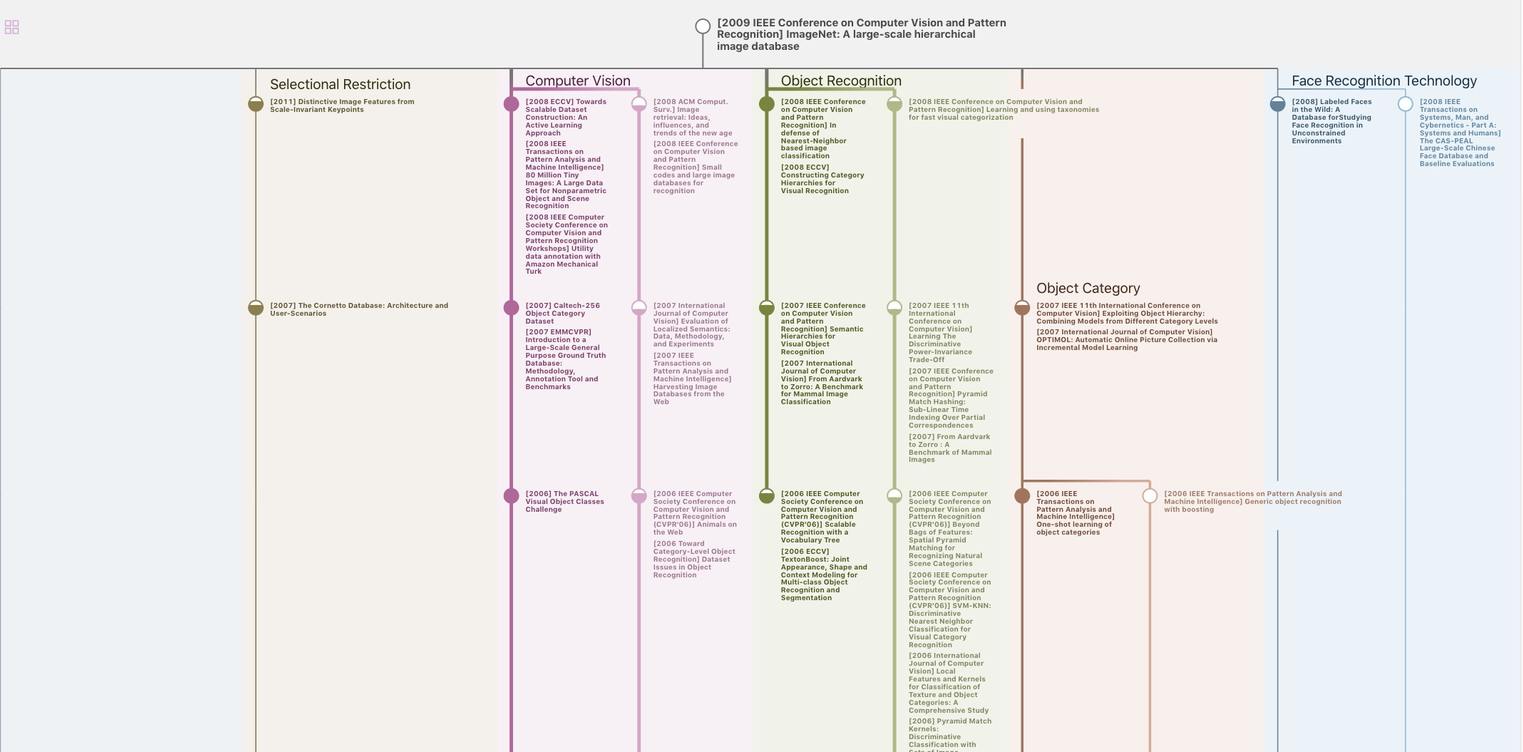
生成溯源树,研究论文发展脉络
Chat Paper
正在生成论文摘要