Sub-band Convolutional Neural Networks for Small-footprint Spoken Term Classification
INTERSPEECH(2019)
摘要
This paper proposes a Sub-band Convolutional Neural Network for spoken term classification. Convolutional neural networks (CNNs) have proven to be very effective in acoustic applications such as spoken term classification, keyword spotting, speaker identification, acoustic event detection, etc. Unlike applications in computer vision, the spatial invariance property of 2D convolutional kernels does not fit acoustic applications well since the meaning of a specific 2D kernel varies a lot along the feature axis in an input feature map. We propose a sub-band CNN architecture to apply different convolutional kernels on each feature sub-band, which makes the overall computation more efficient. Experimental results show that the computational efficiency brought by sub-band CNN is more beneficial for small-footprint models. Compared to a baseline full band CNN for spoken term classification on a publicly available Speech Commands dataset, the proposed sub-band CNN architecture reduces the computation by 39.7% on commands classification, and 49.3% on digits classification with accuracy maintained.
更多查看译文
关键词
spoken term classification, convolutional neural network (CNN), sub-band feature
AI 理解论文
溯源树
样例
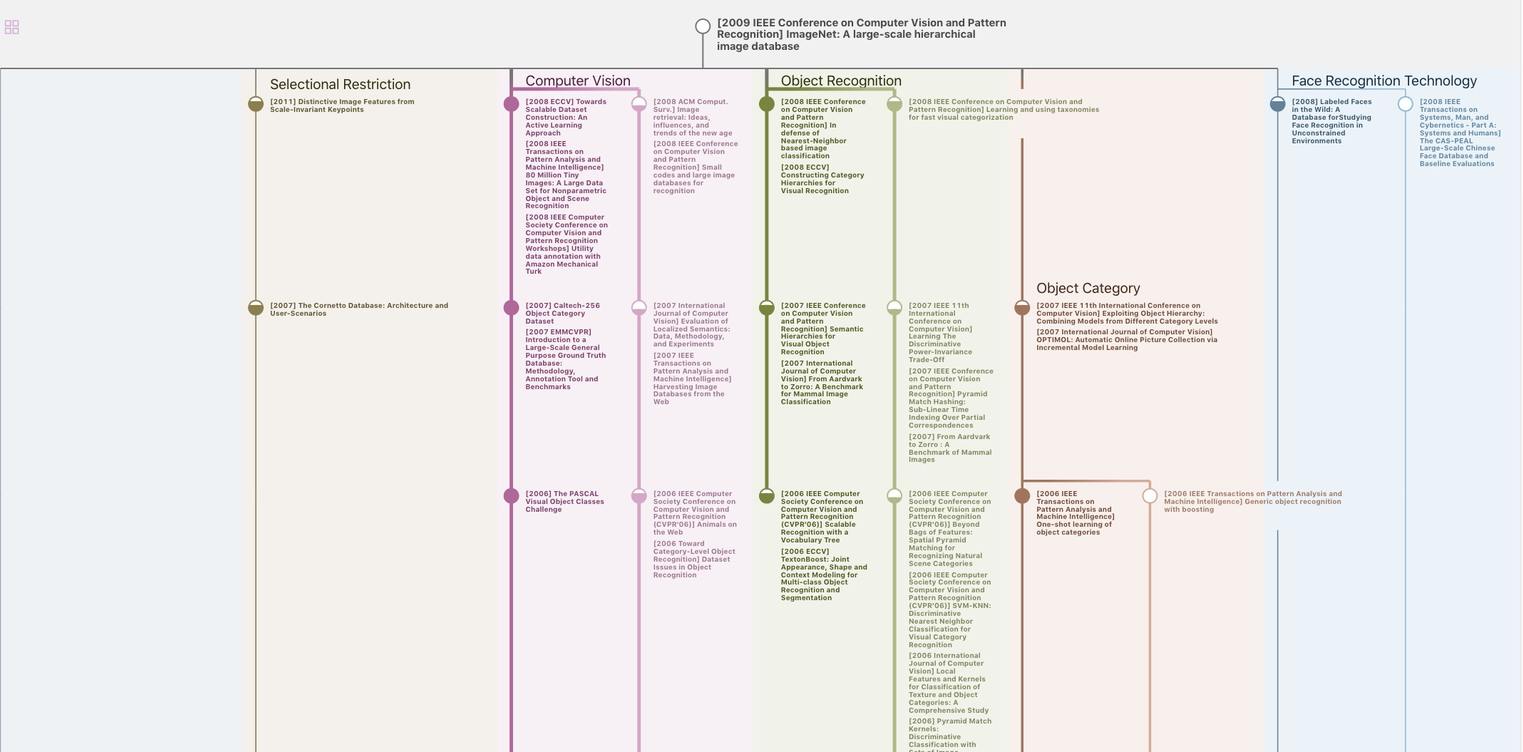
生成溯源树,研究论文发展脉络
Chat Paper
正在生成论文摘要