Anatomical context improves deep learning on the brain age estimation task
Magnetic Resonance Imaging(2019)
Abstract
Deep learning has shown remarkable improvements in the analysis of medical images without the need for engineered features. In this work, we hypothesize that deep learning is complementary to traditional feature estimation. We propose a network design to include traditional structural imaging features alongside deep convolutional ones and illustrate this approach on the task of imaging-based age prediction in two separate contexts: T1-weighted brain magnetic resonance imaging (MRI) (N = 5121, ages 4–96, healthy controls) and computed tomography (CT) of the head (N = 1313, ages 1–97, healthy controls). In brain MRI, we can predict age with a mean absolute error of 4.08 years by combining raw images along with engineered structural features, compared to 5.00 years using image-derived features alone and 8.23 years using structural features alone. In head CT, we can predict age with a median absolute error of 9.99 years combining features, compared to 11.02 years with image-derived features alone and 13.28 years with structural features alone. These results show that we can complement traditional feature estimation using deep learning to improve prediction tasks. As the field of medical image processing continues to integrate deep learning, it will be important to use the new techniques to complement traditional imaging features instead of fully displacing them.
MoreTranslated text
Key words
Deep learning,Convolutional neural networks,Brain age,Medical image processing
AI Read Science
Must-Reading Tree
Example
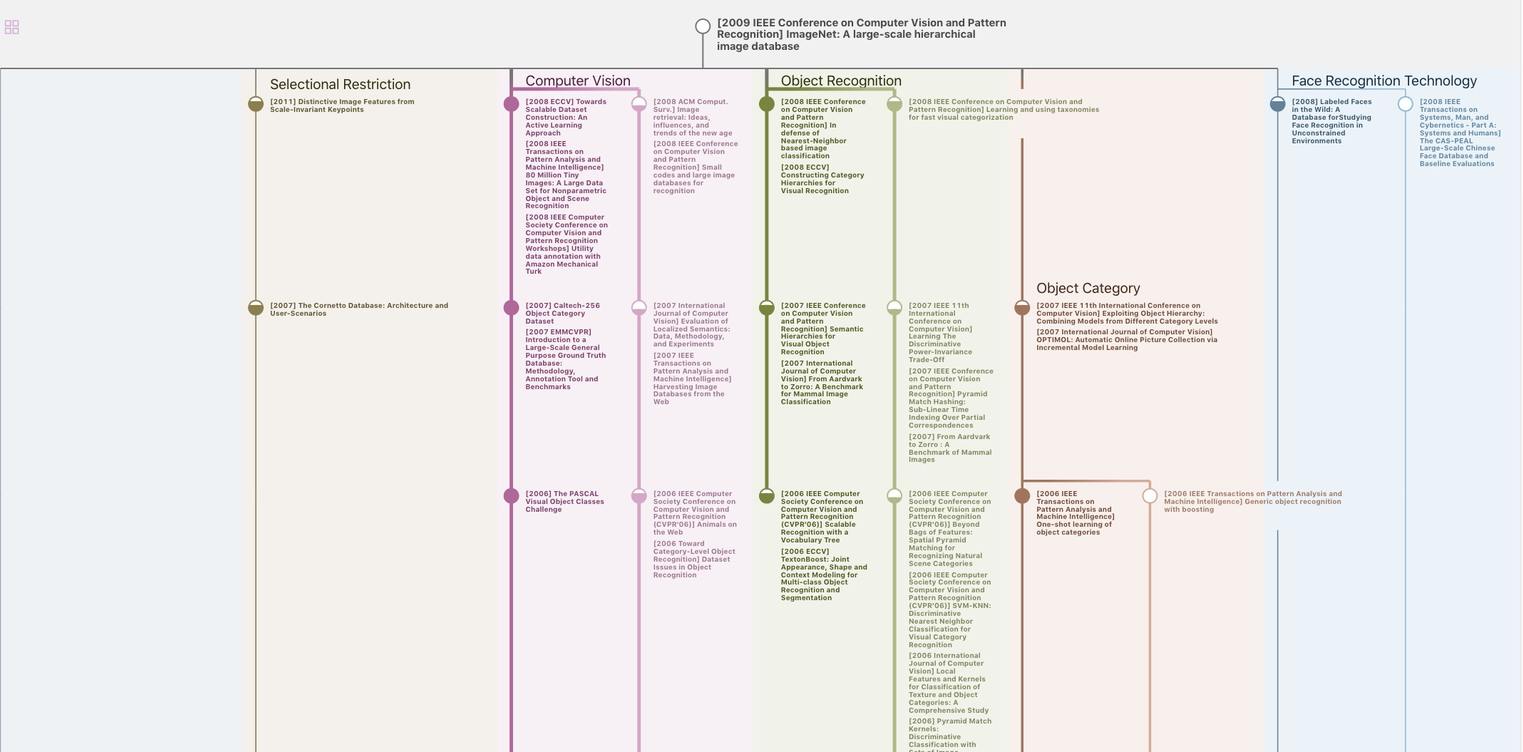
Generate MRT to find the research sequence of this paper
Chat Paper
Summary is being generated by the instructions you defined