A Stochastic Composite Gradient Method with Incremental Variance Reduction
ADVANCES IN NEURAL INFORMATION PROCESSING SYSTEMS 32 (NIPS 2019)(2019)
摘要
We consider the problem of minimizing the composition of a smooth (nonconvex) function and a smooth vector mapping, where the inner mapping is in the form of an expectation over some random variable or a finite sum. We propose a stochastic composite gradient method that employs an incremental variance-reduced estimator for both the inner vector mapping and its Jacobian. We show that this method achieves the same orders of complexity as the best known first-order methods for minimizing expected-value and finite-sum nonconvex functions, despite the additional outer composition which renders the composite gradient estimator biased. This finding enables a much broader range of applications in machine learning to benefit from the low complexity of incremental variance-reduction methods.
更多查看译文
关键词
machine learning,random variable
AI 理解论文
溯源树
样例
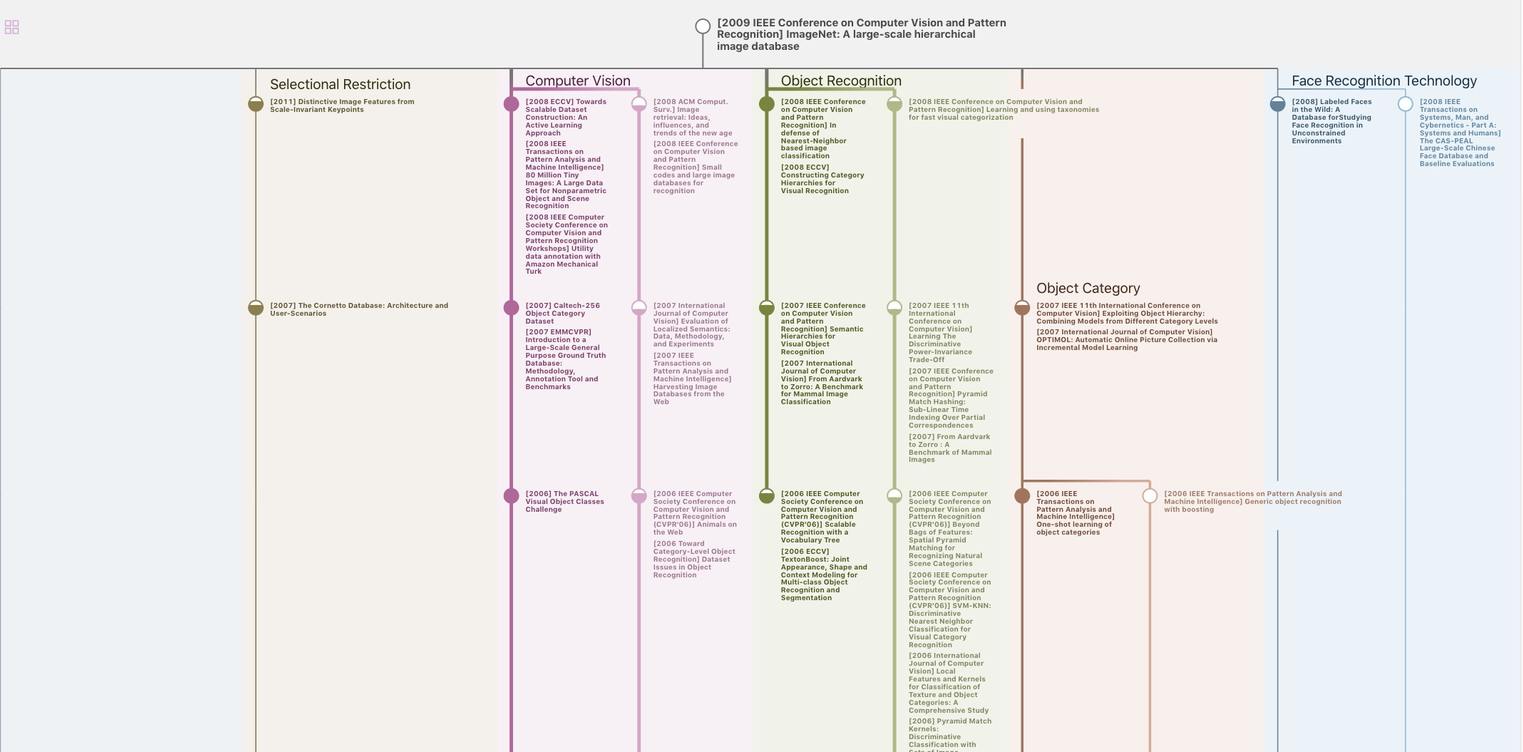
生成溯源树,研究论文发展脉络
Chat Paper
正在生成论文摘要