Fast Calculation of Probabilistic Optimal Power Flow: A Deep Learning Approach
CoRR(2019)
摘要
Probabilistic optimal power flow (POPF) is an important analytical tool to ensure the secure and economic operation of power systems. POPF needs to solve enormous nonlinear and nonconvex optimization problems. The huge computational burden has become the major bottleneck for the practical application. This paper presents a deep learning approach to solve the POPF problem efficiently and accurately. Taking advantage of the deep structure and reconstructive strategy of stacked denoising auto encoders (SDAE), a SDAE-based optimal power flow (OPF) is developed to extract the high-level nonlinear correlations between the system operating condition and the OPF solution. A training process is designed to learn the feature of POPF. The trained SDAE network can be utilized to conveniently calculate the OPF solution of random samples generated by Monte-Carlo simulation (MCS) without the need of optimization. A modified IEEE 118-bus power system is simulated to demonstrate the effectiveness of the proposed method.
更多查看译文
关键词
Probabilistic optimal power flow (POPF),deep learning,stacked denoising auto encoders (SDAE),Monte-Carlo simulation (MCS)
AI 理解论文
溯源树
样例
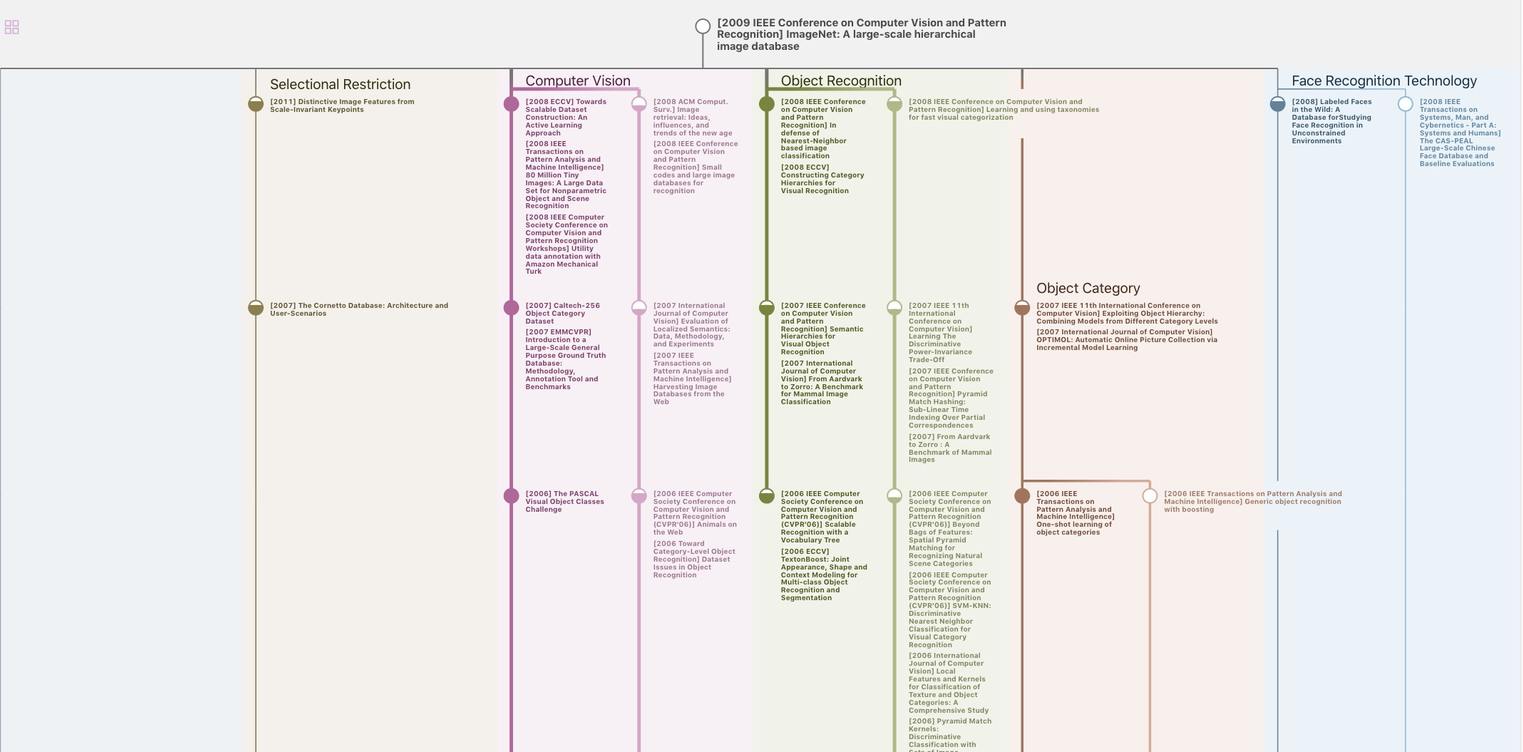
生成溯源树,研究论文发展脉络
Chat Paper
正在生成论文摘要