Bias Correction of Learned Generative Models using Likelihood-Free Importance Weighting.
ADVANCES IN NEURAL INFORMATION PROCESSING SYSTEMS 32 (NIPS 2019)(2019)
摘要
A learned generative model often produces biased statistics relative to the underlying data distribution. A standard technique to correct this bias is importance sampling, where samples from the model are weighted by the likelihood ratio under model and true distributions. When the likelihood ratio is unknown, it can be estimated by training a probabilistic classifier to distinguish samples from the two distributions. We show that this likelihood-free importance weighting method induces a new energy-based model and employ it to correct for the bias in existing models. We find that this technique consistently improves standard goodness-of-fit metrics for evaluating the sample quality of state-of-the-art deep generative models, suggesting reduced bias. Finally, we demonstrate its utility on representative applications in a) data augmentation for classification using generative adversarial networks, and b) model-based policy evaluation using off-policy data.
更多查看译文
关键词
generative adversarial network (gan),importance sampling
AI 理解论文
溯源树
样例
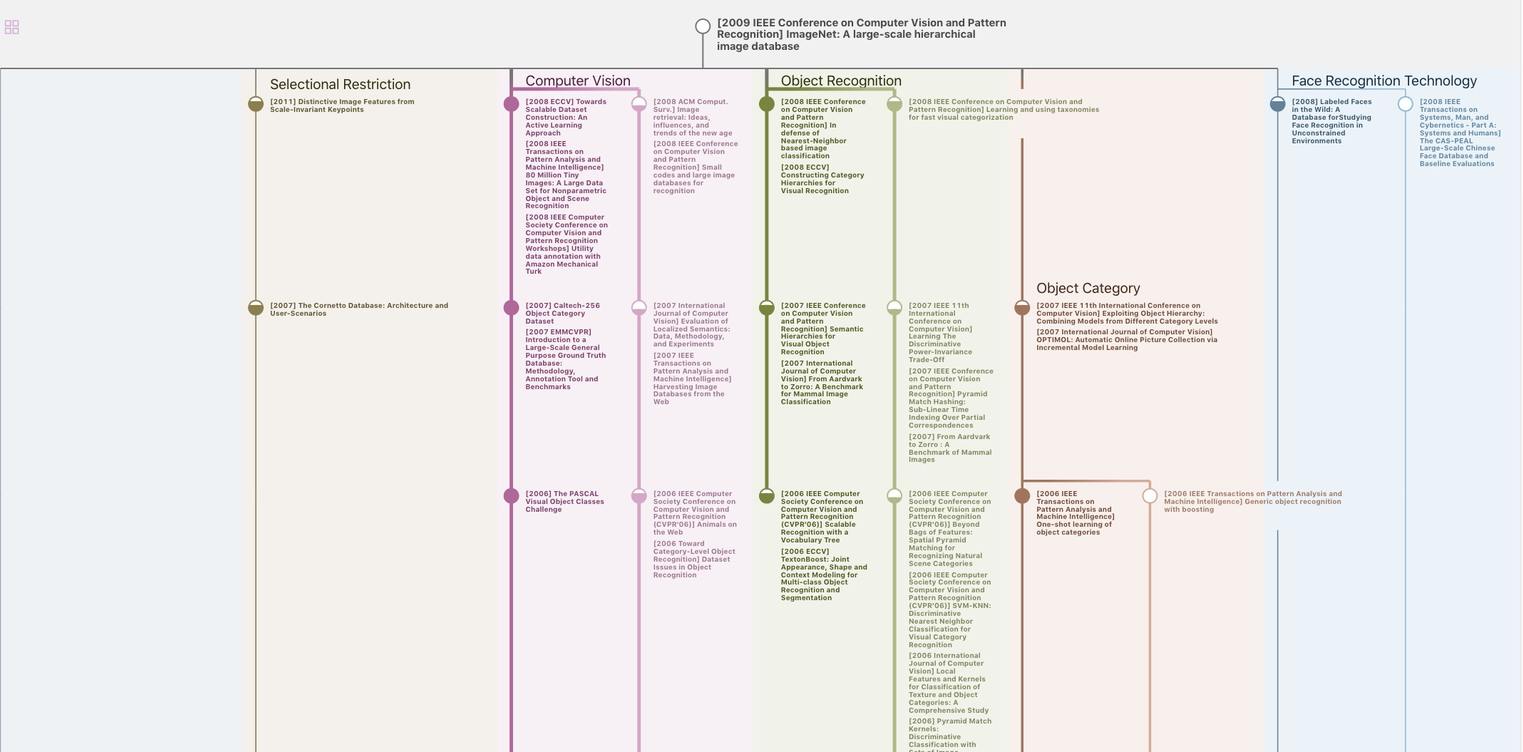
生成溯源树,研究论文发展脉络
Chat Paper
正在生成论文摘要