Flattening A Hierarchical Clustering Through Active Learning
ADVANCES IN NEURAL INFORMATION PROCESSING SYSTEMS 32 (NIPS 2019)(2019)
摘要
We investigate active learning by pairwise similarity over the leaves of trees originating from hierarchical clustering procedures. In the realizable setting, we provide a full characterization of the number of queries needed to achieve perfect reconstruction of the tree cut. In the non-realizable setting, we rely on known important-sampling procedures to obtain regret and query complexity bounds. Our algorithms come with theoretical guarantees on the statistical error and, more importantly, lend themselves to linear-time implementations in the relevant parameters of the problem. We discuss such implementations, prove running time guarantees for them, and present preliminary experiments on real-world datasets showing the compelling practical performance of our algorithms as compared to both passive learning and simple active learning baselines.
更多查看译文
关键词
hierarchical clustering,passive learning
AI 理解论文
溯源树
样例
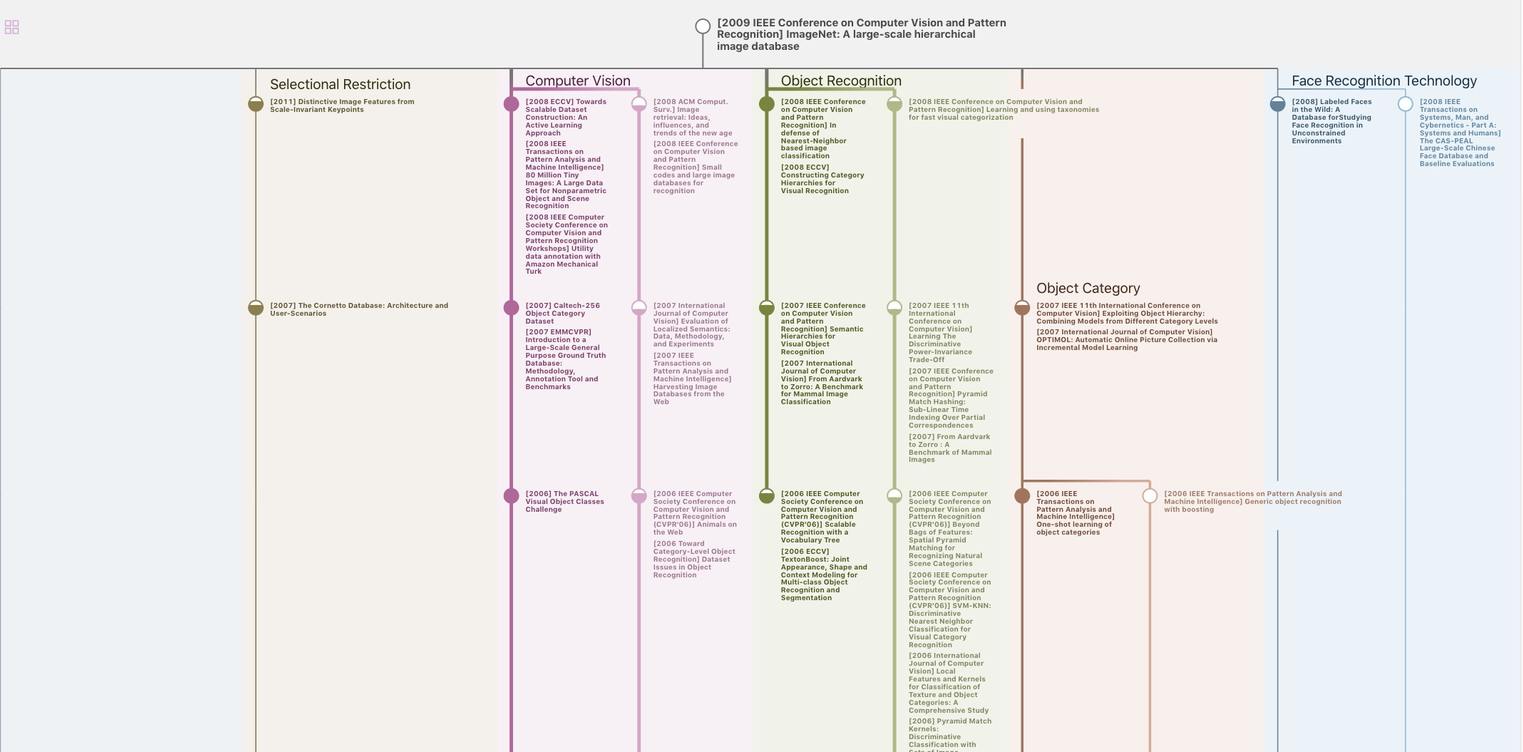
生成溯源树,研究论文发展脉络
Chat Paper
正在生成论文摘要