Genetic Algorithm-Based Sensor Allocation With Nonlinear Centralized Fusion Observable Degree.
IEEE transactions on neural networks and learning systems(2019)
摘要
As the main performance self-evaluation index of the Kalman filter, the estimation error covariance (EEC) has been used to design the allocation cost function of task and resources for sensor tracking networks. For nonlinear systems, the sensor allocation method based on the EEC needs to adjust the allocation plans after obtaining the filtering results. Meanwhile, recent investigations have indicated that the self-evaluation function EEC of the Kalman filtering is universally inapplicable in practical applications, for which the estimation models are generally mismatched due to difficulty in accurately training parameters and approximation of nonlinear systems. Thereby, the sensors cannot be properly allocated by using the EEC as a preliminary criterion. Alternatively, observable degree (OD) is a naturally quantitative measure on observability and can be utilized to effectively measure the estimation performance. In this paper, the OD analysis with scale transform invariance for nonlinear systems is studied by using the unscented Kalman filter, the pseudostate transition matrix, and the pseudo observation matrix on the basis of the results of linear systems. Afterward, the OD of nonlinear fusion systems, the sensor utilization efficiency, the priority of tasks, and the sensor performance and sensitivity are jointly considered to formulate the optimization problem for sensor allocation. The genetic algorithm with intelligent learning function is employed to solve the optimization problem. Moreover, extensive simulation demonstrates the feasibility of the proposed approach.
更多查看译文
关键词
Resource management,Kalman filters,Observability,Optimization,Nonlinear systems,Genetic algorithms,Linear systems
AI 理解论文
溯源树
样例
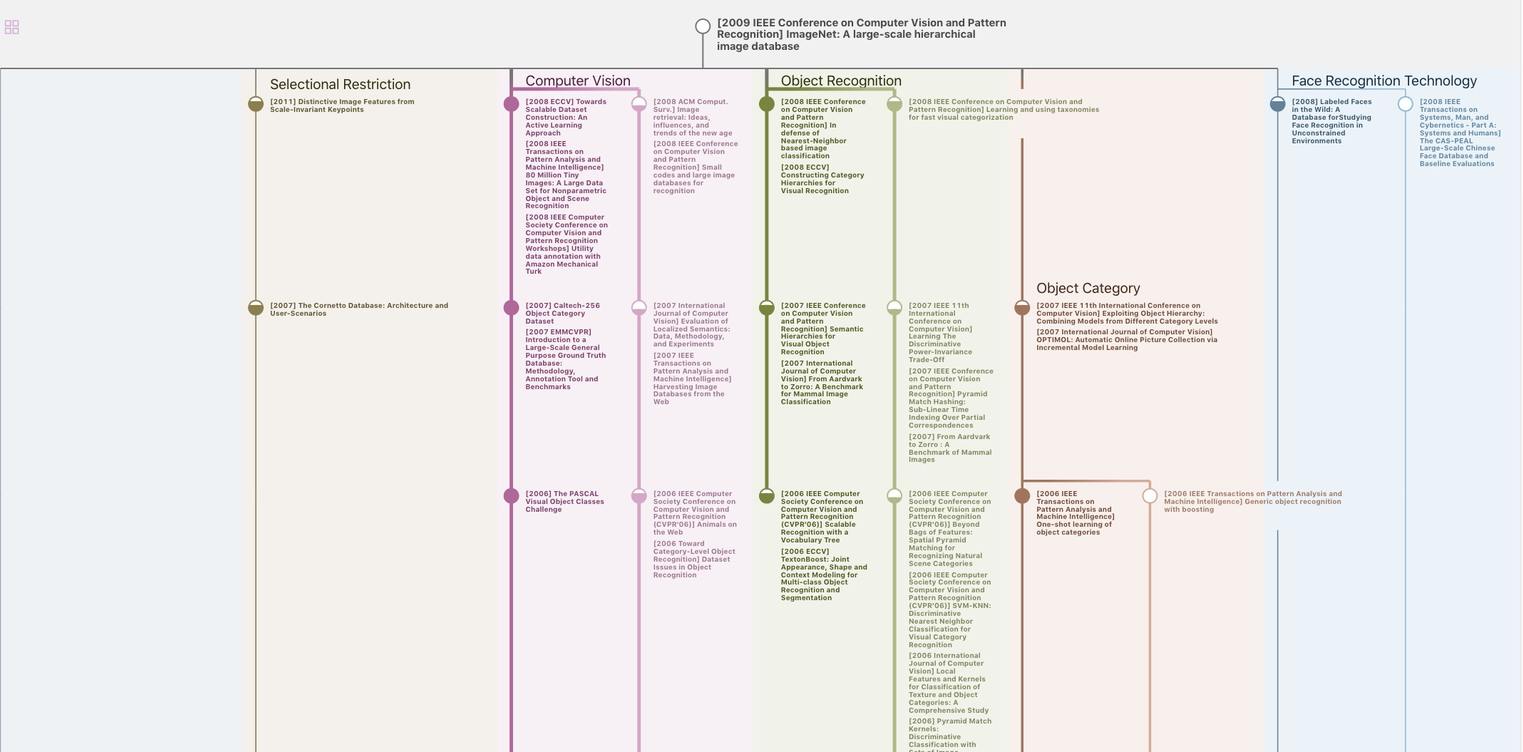
生成溯源树,研究论文发展脉络
Chat Paper
正在生成论文摘要