NEIVA: Environment Identification based Video Bitrate Adaption in Cellular Networks
2019 IEEE/ACM 27th International Symposium on Quality of Service (IWQoS)(2019)
摘要
With the popularization of advanced cellular networks, mobile video occupies nearly three quarters of cellular network traffic. While previous adaptive bitrate (ABR) algorithms perform well under broadband network, their performance degrades in cellular networks due to throughput fluctuation. Through real world 4G/LTE network measurement, we find that throughput in cellular networks exhibits high fluctuation. It follows Markov behaviors with different states and different transition probability among states. We further find that the transition probability is stable along time but varies significantly under different environments. This inspires us to design ABR algorithms by improving throughput prediction in cellular networks. We propose NEIVA, a network environment identification based video bitrate adaption method in cellular networks. NEIVA trains a network environment identifier based on throughput data and trains a hidden Markov model (HMM) based throughput predictor for different environments. In online video bitrate selection, NEIVA utilizes the environment identifier to select the model for corresponding environment. Then NEIVA predicts future network performance by combining offline model and online throughput data. We implement NEIVA with MPC and evaluate it in real environment. The evaluation results show that with manually identifying environment, NEIVA improves 20%–25% bandwidth prediction accuracy and 11%–20% QoE improvement over the baseline predictors. With online environment identification, online NEIVA achieves 3.8% and 11.1% average QoE improvement over MPC and HMM, respectively.
更多查看译文
关键词
4G/LTE bandwidth measurement,quality of experience,adaptive bitrate selection,environment identification,throughput prediction
AI 理解论文
溯源树
样例
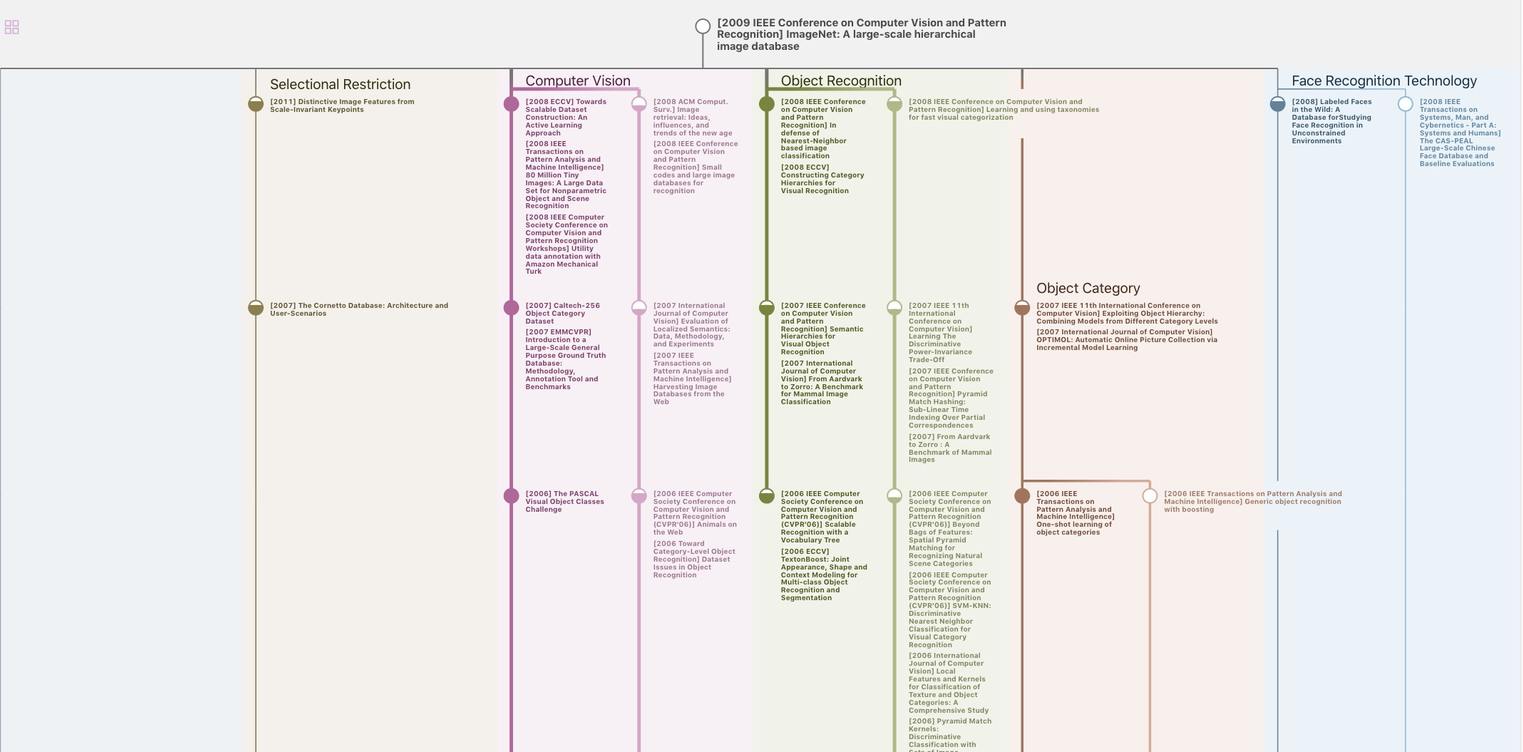
生成溯源树,研究论文发展脉络
Chat Paper
正在生成论文摘要