Application of Extreme Learning Machine for Predicting Chlorophyll-a Concentration Inartificial Upwelling Processes
MATHEMATICAL PROBLEMS IN ENGINEERING(2019)
摘要
Artificial upwelling, artificially pumping up nutrient-rich ocean waters from deep to surface, is increasingly applied to stimulating phytoplankton activity. As a proxy for the amount of phytoplankton present in the ocean, the concentration of chlorophyll a (chl-a) may be influenced by water physical factors altered in artificial upwelling processes. However, the accuracy and convenience of measuring chl-a are limited by present technologies and equipment. Our research intends to study the correlations between chl-a concentration and five water physical factors, i.e., salinity, temperature, depth, dissolved oxygen (DO), and pH, possibly affected by artificial upwelling. In this paper, seven models are presented to predict chl-a concentration, respectively. Two of them are based on traditional regression algorithms, i.e., multiple linear regression (MLR) and multivariate quadratic regression (MQR), while five are based on intelligent algorithms, i.e., backpropagation-neural network (BP-NN), extreme learning machine (ELM), genetic algorithm-ELM (GA-ELM), particle swarm optimization-ELM (PSO-ELM), and ant colony optimization-ELM (ACO-ELM). These models provide a quick prediction to study the concentration of chl-a. With the experimental data collected from Xinanjiang Experiment Station in China, the results show that chl-a concentration has a strong correlation with salinity, temperature, DO, and pH in the process of artificial upwelling and PSO-ELM has the best overall prediction ability.
更多查看译文
关键词
extreme learning machine,concentration inartificial upwelling processes
AI 理解论文
溯源树
样例
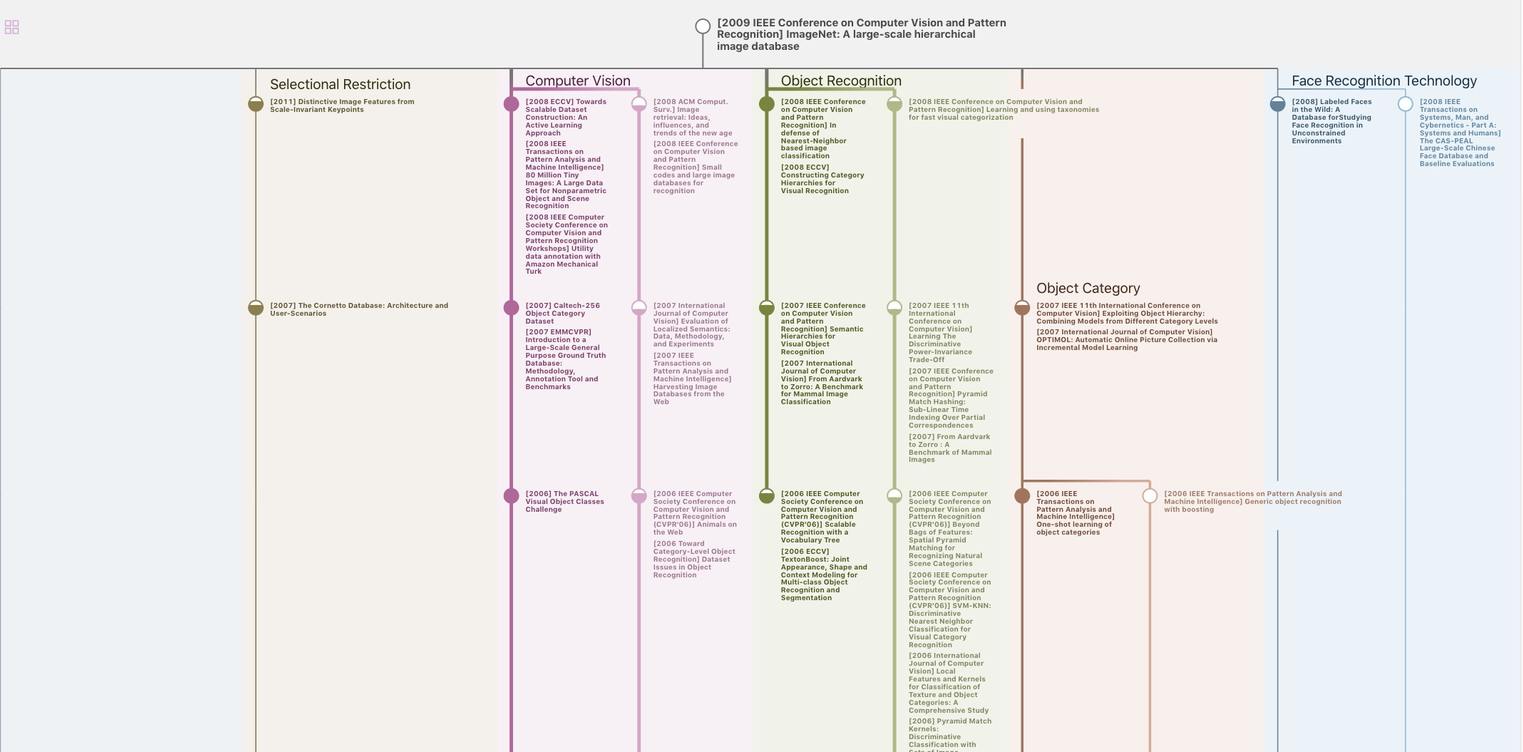
生成溯源树,研究论文发展脉络
Chat Paper
正在生成论文摘要