Confined teaching-learning-based optimization with variable search strategies for continuous optimization
Information Sciences(2019)
摘要
The well-known optimization approach teaching-learning-based optimization (TLBO) is modified by using a confined TLBO (CTLBO) to eliminate the teaching factor. Different settings are suggested for various types of search factors, as they are used for different purposes. In addition, crossover frequencies are introduced into TLBO to prevent premature convergence. Furthermore, eight new mutation strategies are introduced to the teacher phase, and four new mutation strategies to the student phase to enhance the algorithm's exploitation and exploration capabilities. The experimental results show that the proposed versions, especially those that either adopted low crossover frequencies or implemented various mutation strategies, performed particularly well in achieving fast convergence speeds in the early stages, reaching convergence precision at lower cost, arriving at convergence plateaus at either lower cost or higher precision, handling tests of composition functions well, and achieving competitive performance on CEC2015 test problems.
更多查看译文
关键词
Teaching-learning-based optimization,Continuous optimization,Teaching factor,Crossover rates,Mutation strategies,Search factors
AI 理解论文
溯源树
样例
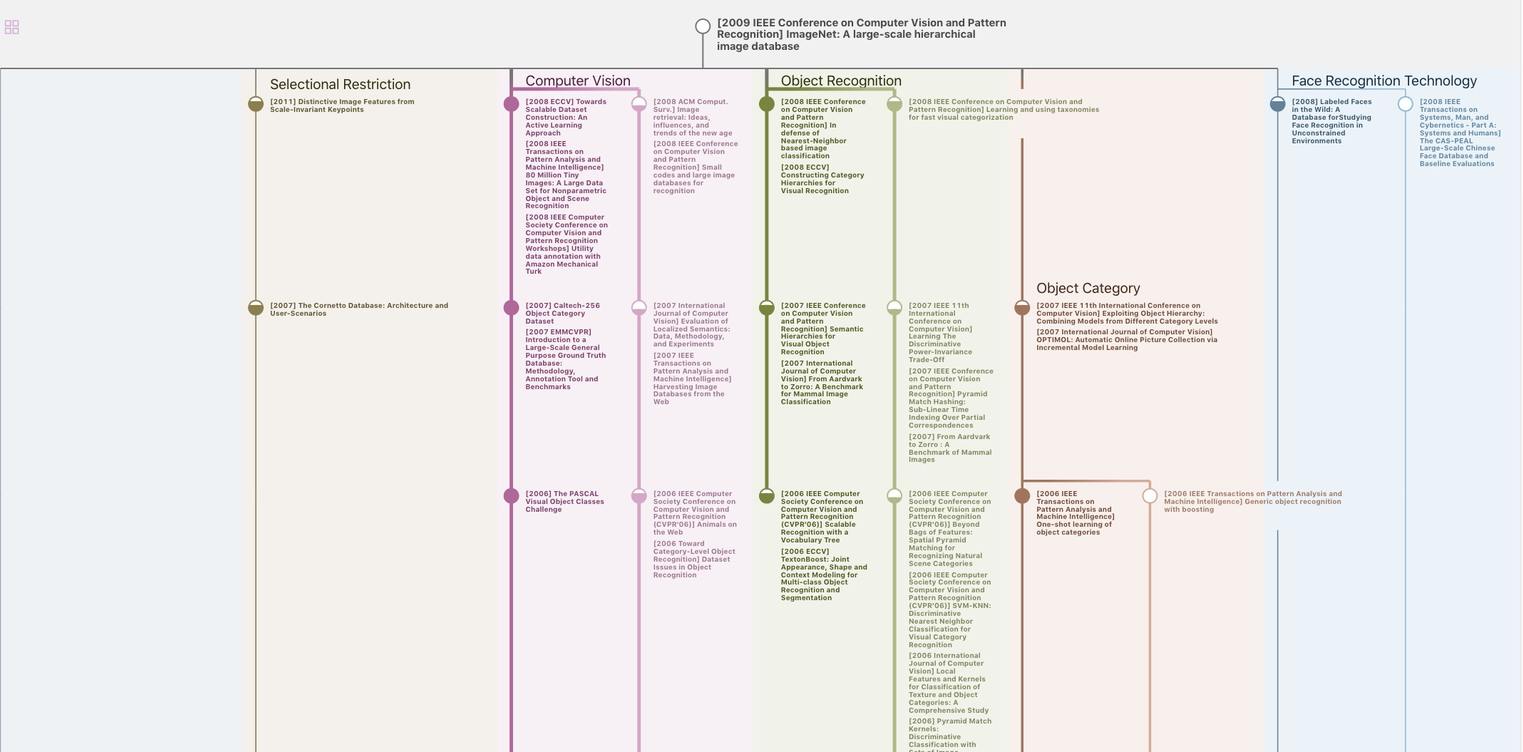
生成溯源树,研究论文发展脉络
Chat Paper
正在生成论文摘要