Machine Learning Prediction Approach to Enhance Congestion Control in 5G IoT Environment
ELECTRONICS(2019)
摘要
The 5G network is a next-generation wireless form of communication and the latest mobile technology. In practice, 5G utilizes the Internet of Things (IoT) to work in high-traffic networks with multiple nodes/sensors in an attempt to transmit their packets to a destination simultaneously, which is a characteristic of IoT applications. Due to this, 5G offers vast bandwidth, low delay, and extremely high data transfer speed. Thus, 5G presents opportunities and motivations for utilizing next-generation protocols, especially the stream control transmission protocol (SCTP). However, the congestion control mechanisms of the conventional SCTP negatively influence overall performance. Moreover, existing mechanisms contribute to reduce 5G and IoT performance. Thus, a new machine learning model based on a decision tree (DT) algorithm is proposed in this study to predict optimal enhancement of congestion control in the wireless sensors of 5G IoT networks. The model was implemented on a training dataset to determine the optimal parametric setting in a 5G environment. The dataset was used to train the machine learning model and enable the prediction of optimal alternatives that can enhance the performance of the congestion control approach. The DT approach can be used for other functions, especially prediction and classification. DT algorithms provide graphs that can be used by any user to understand the prediction approach. The DT C4.5 provided promising results, with more than 92% precision and recall.
更多查看译文
关键词
machine learning,decision tree algorithm,IoT,WSN,C4.5,congestion control,5G network
AI 理解论文
溯源树
样例
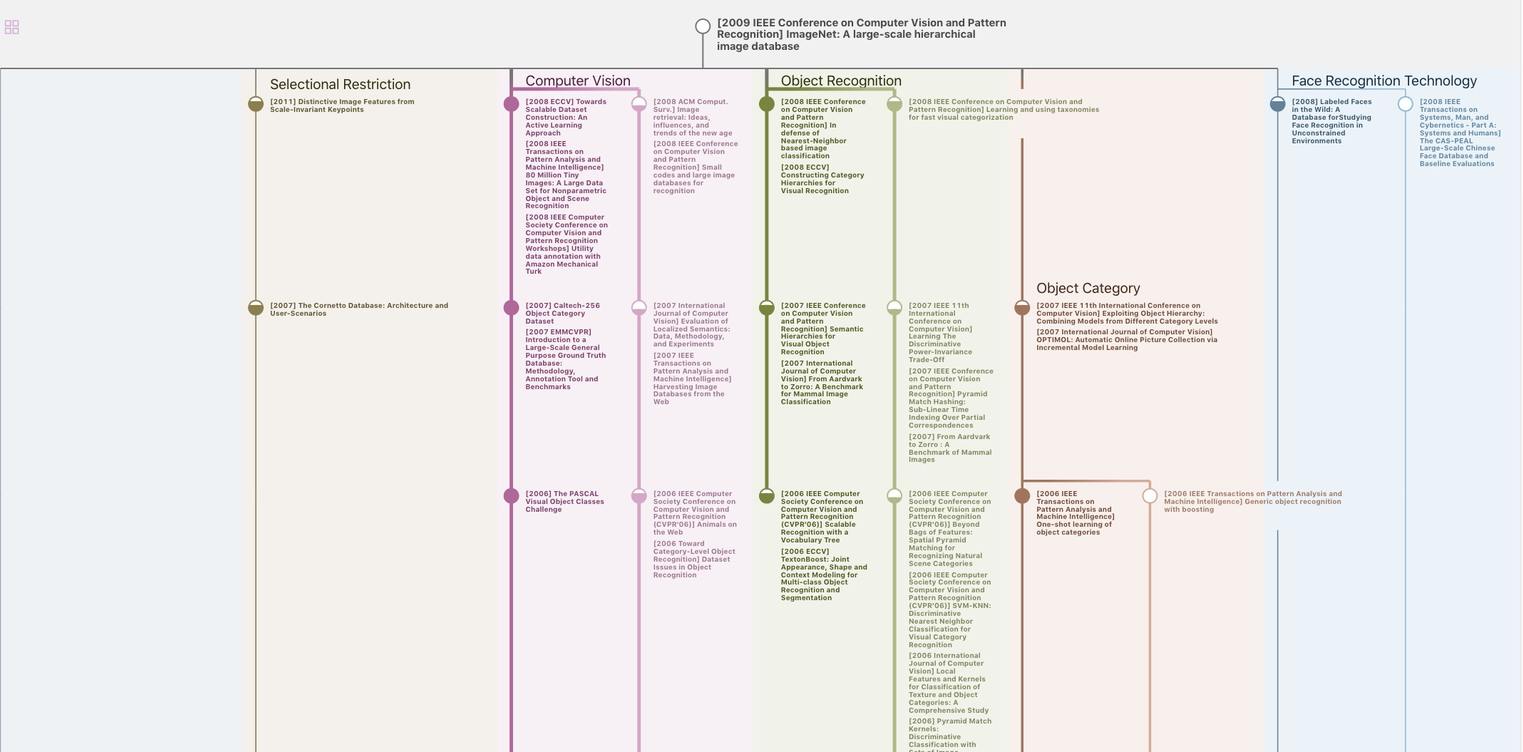
生成溯源树,研究论文发展脉络
Chat Paper
正在生成论文摘要