Riemannian Geometry Learning For Disease Progression Modelling
INFORMATION PROCESSING IN MEDICAL IMAGING, IPMI 2019(2019)
摘要
The analysis of longitudinal trajectories is a longstanding problem in medical imaging which is often tackled in the context of Riemannian geometry: the set of observations is assumed to lie on an a priori known Riemannian manifold. When dealing with high-dimensional or complex data, it is in general not possible to design a Riemannian geometry of relevance. In this paper, we perform Riemannian manifold learning in association with the statistical task of longitudinal trajectory analysis. After inference, we obtain both a submanifold of observations and a Riemannian metric so that the observed progressions are geodesics. This is achieved using a deep generative network, which maps trajectories in a low-dimensional Euclidean space to the observation space.
更多查看译文
关键词
Riemannian geometry, Longitudinal progression, Medical imaging
AI 理解论文
溯源树
样例
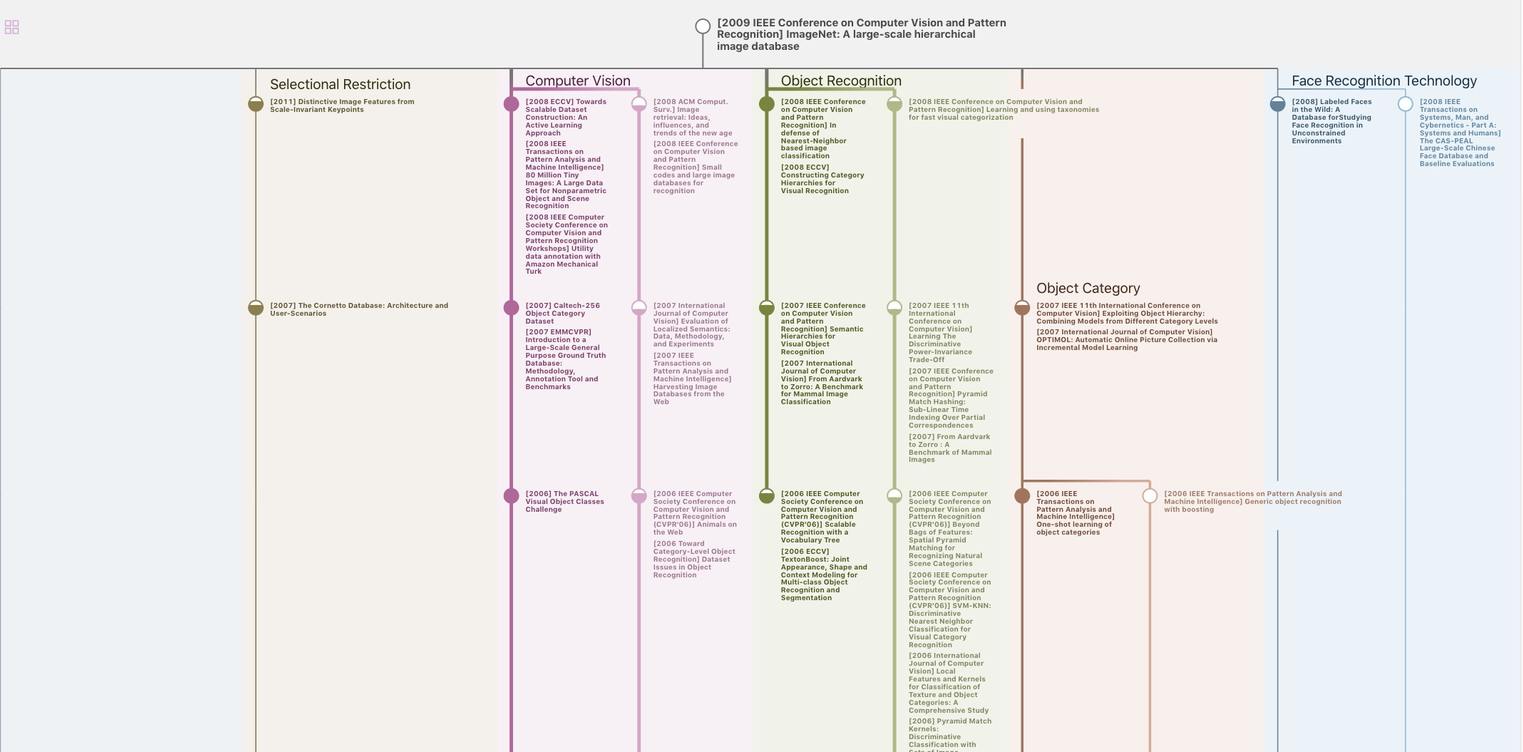
生成溯源树,研究论文发展脉络
Chat Paper
正在生成论文摘要