A Crowdsourcing Framework for Collecting Tabular Data
IEEE Transactions on Knowledge and Data Engineering(2020)
摘要
In crowdsourcing, human workers are employed to tackle problems that are traditionally difficult for computers (e.g., data cleaning, missing value filling, and sentiment analysis). In this paper, we study the effective use of crowdsourcing in filling missing values in a given relation (e.g., a table containing different attributes of celebrity stars, such as nationality and age). A task given to a worker typically consists of questions about the missing attribute values (e.g., What is the age of Jet Li?). Although this problem has been studied before, existing work often treats related attributes independently, leading to suboptimal performance. In this paper, we present T-Crowd, which is a crowdsourcing system that considers attribute relationships. Particularly, T-Crowd integrates each worker's answers on different attributes to effectively learn his/her trustworthiness and the true data values. The attribute relationship information is used to guide task allocation to workers. Our solution seamlessly supports categorical and continuous attributes. Our extensive experiments on real and synthetic datasets show that T-Crowd outperforms state-of-the-art methods, improving the quality of truth inference and reducing the monetary cost of crowdsourcing.
更多查看译文
关键词
Task analysis,Crowdsourcing,Computers,Cleaning,Data models,Inference algorithms,Estimation
AI 理解论文
溯源树
样例
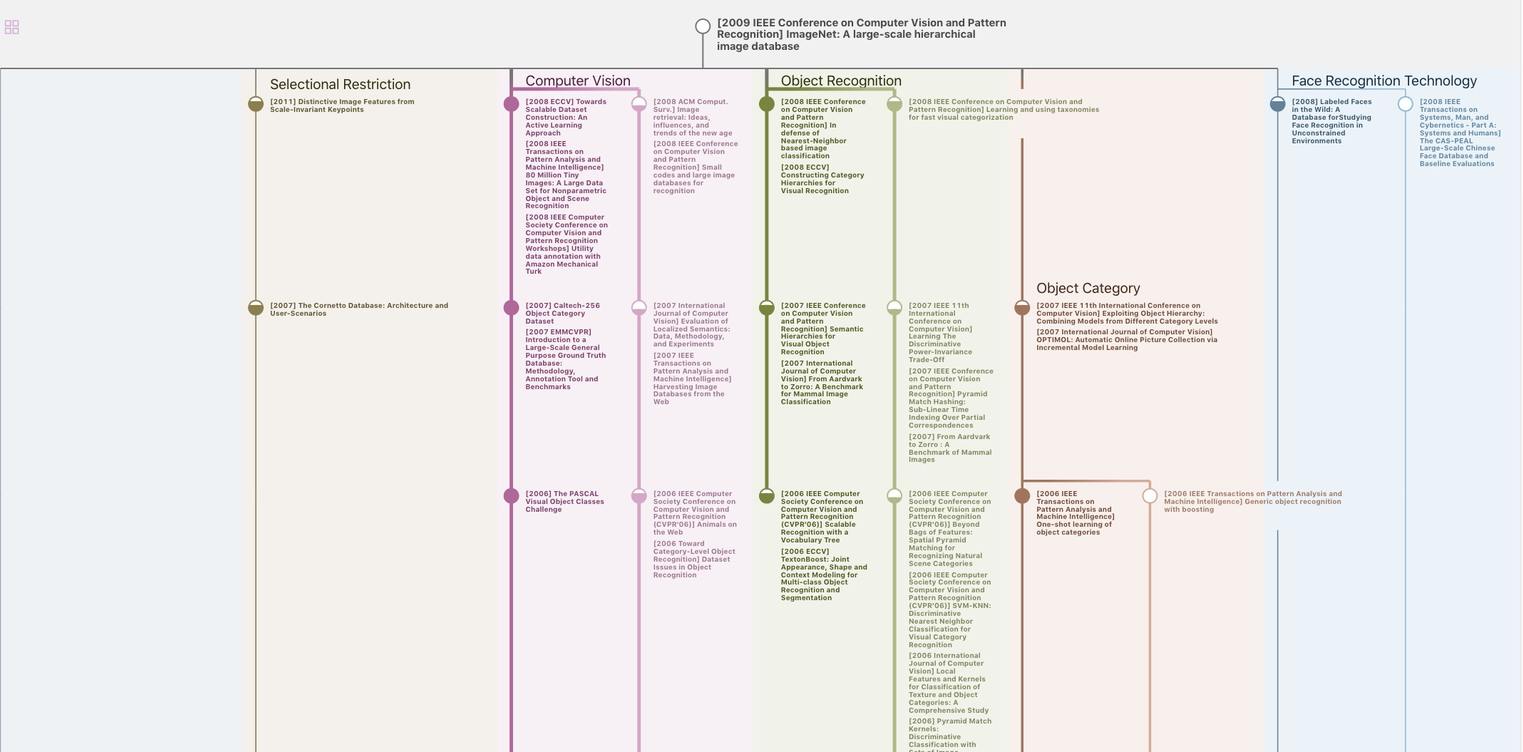
生成溯源树,研究论文发展脉络
Chat Paper
正在生成论文摘要