Trimmed Robust Loss Function For Training Deep Neural Networks With Label Noise
ARTIFICIAL INTELLIGENCEAND SOFT COMPUTING, PT I(2019)
摘要
Deep neural networks obtain nowadays outstanding results on many vision, speech recognition and natural language processing-related tasks. Such deep structures need to be trained on very large datasets, what makes annotating the data for supervised learning, particularly difficult and time-consuming task. In the supervised datasets label noise may occur, which makes the whole training process less reliable. In this paper we present a novel robust loss function based on categorical cross-entropy. We demonstrate its robustness for several amounts of noisy labels, on popular MNIST and CIFAR-10 datasets.
更多查看译文
关键词
Neural networks, Deep learning, Robust learning, Label noise, Categorical cross-entropy
AI 理解论文
溯源树
样例
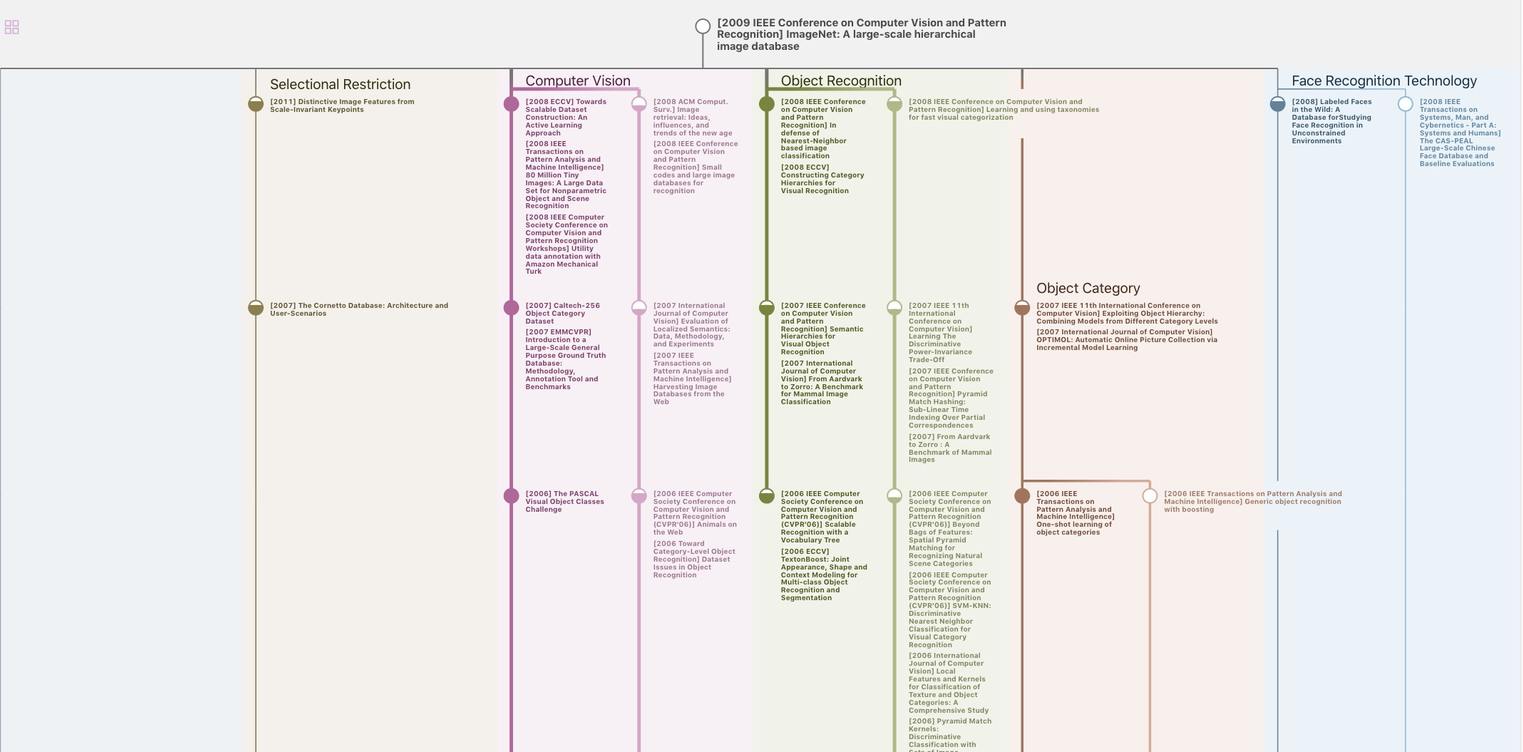
生成溯源树,研究论文发展脉络
Chat Paper
正在生成论文摘要