Decoding Kinematics From Human Parietal Cortex Using Neural Networks
2019 9TH INTERNATIONAL IEEE/EMBS CONFERENCE ON NEURAL ENGINEERING (NER)(2019)
摘要
Brain-machine interfaces have shown promising results in providing control over assistive devices for paralyzed patients. In this work we describe a BMI system using electrodes implanted in the parietal lobe of a tetraplegic subject. Neural data used for the decoding was recorded in five 3-minute blocks during the same session. Within each block, the subject uses motor imagery to control a cursor in a 2D center-out task. We compare performance for four different algorithms: Kalman filter, a two-layer Deep Neural Network (DNN), a Recurrent Neural Network (RNN) with SimpleRNN unit cell (SimpleRNN), and a RNN with Long-Short-Term Memory (LSTM) unit cell. The decoders achieved Pearson Correlation Coefficients (rho) of 0.48, 0.39, 0.77 and 0.75, respectively, in the Y-coordinate, and 0.24, 0.20, 0.46 and 0.47, respectively, in the X-coordinate.
更多查看译文
关键词
neural data,two-layer deep neural network,recurrent neural network,Pearson correlation coefficients,long-short-term memory unit cell,decoders,SimpleRNN unit cell,Kalman filter,2D center-out task,subject uses motor imagery,3-minute blocks,tetraplegic subject,parietal lobe,BMI system,paralyzed patients,assistive devices,brain-machine interfaces,Neural networks,human parietal cortex,decoding kinematics
AI 理解论文
溯源树
样例
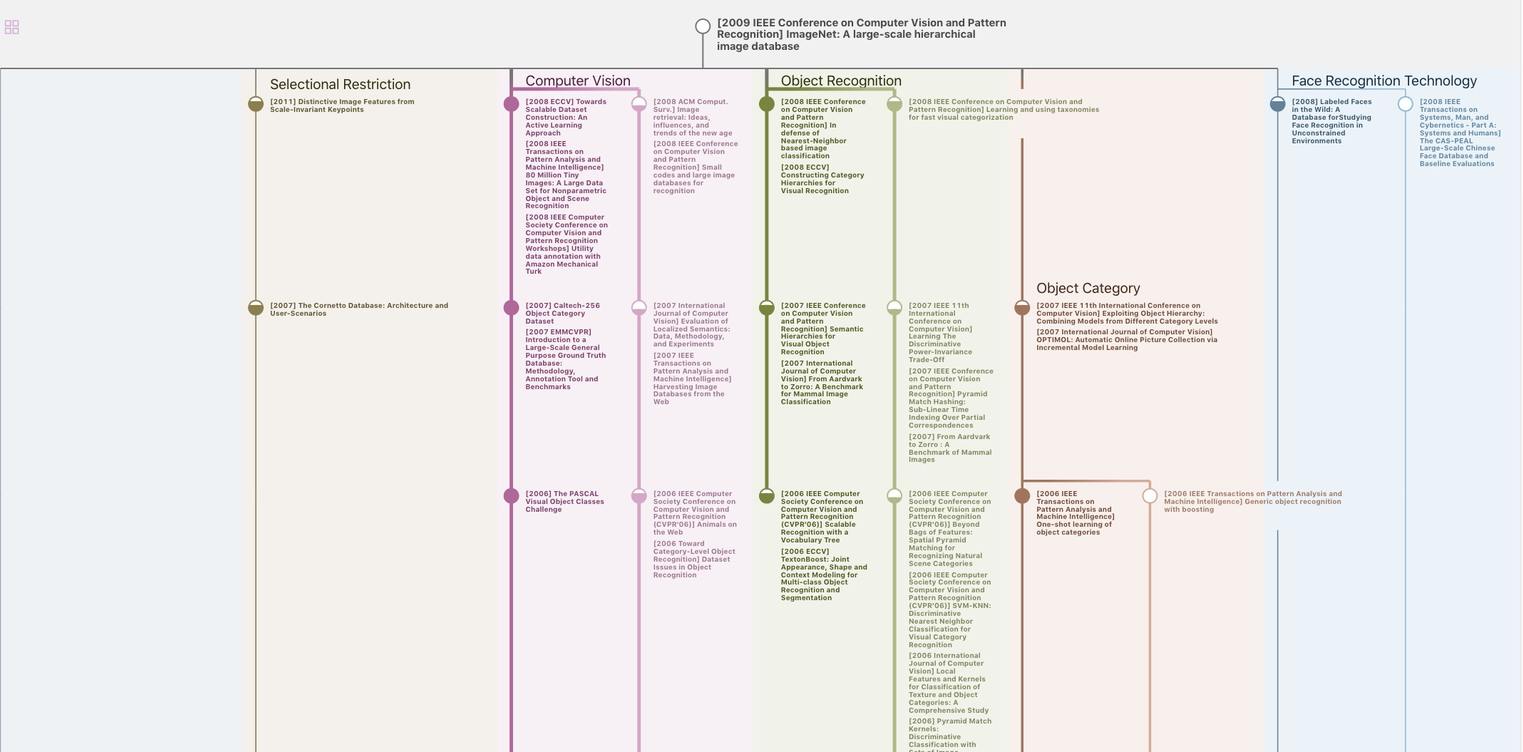
生成溯源树,研究论文发展脉络
Chat Paper
正在生成论文摘要