Learning MILP Resolution Outcomes Before Reaching Time-Limit.
integration of ai and or techniques in constraint programming(2019)
摘要
The resolution of some Mixed-Integer Linear Programming (MILP) problems still presents challenges for state-of-the-art optimization solvers and may require hours of computations, so that a time-limit to the resolution process is typically provided by a user. Nevertheless, it could be useful to get a sense of the optimization trends after only a fraction of the specified total time has passed, and ideally be able to tailor the use of the remaining resolution time accordingly, in a more strategic and flexible way. Looking at the evolution of a partial branch-and-bound tree for a MILP instance, developed up to a certain fraction of the time-limit, we aim to predict whether the problem will be solved to proven optimality before timing out. We exploit machine learning tools, and summarize the development and progress of a MILP resolution process to cast a prediction within a classification framework. Experiments on benchmark instances show that a valuable statistical pattern can indeed be learned during MILP resolution, with key predictive features reflecting the know-how and experience of field's practitioners.
更多查看译文
关键词
MILP resolution, Branch and Bound, Machine learning
AI 理解论文
溯源树
样例
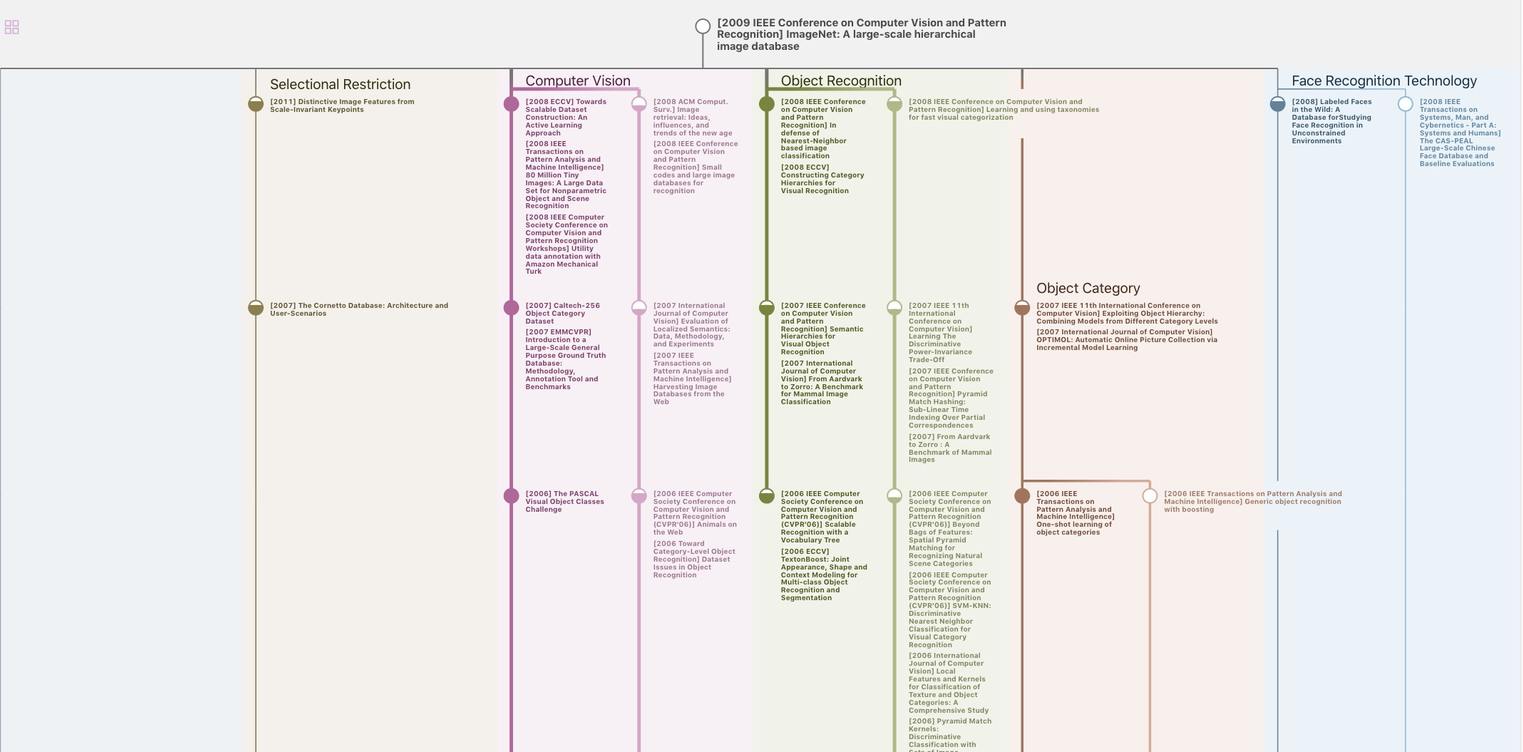
生成溯源树,研究论文发展脉络
Chat Paper
正在生成论文摘要