A minimum entropy deconvolution-enhanced convolutional neural networks for fault diagnosis of axial piston pumps
Soft Computing(2019)
摘要
Condition monitoring of piston pumps has great significance to ensure the reliability and security of hydraulic systems. However, the complex working conditions of the integrated electromechanical systems make the fault mechanism unclear which is difficult for fault diagnosis by feature matching techniques. In this paper, a novel minimum entropy deconvolution (MED)-based convolutional neural network (CNN) is presented to classify faults in axial piston pumps. Firstly, the collected raw signals are preprocessed using the MED technique. Then, the filtered signals are used to construct training samples and testing samples. Finally, the constructed samples are fed into the CNN to classify the multi-faults of axial piston pumps. With the convolution and subsampling operations, the present model can automatically obtain data features via iterative learning processes, which is suitable for the unknown fault mechanism problems. The learned features are visualized by t-distributed stochastic neighbor embedding technique. A benchmark data simulation of mechanical transmission systems and an experimental data investigation of an axial piston pump are performed to manifest the superiority of the present method by comparing with the traditional CNN.
更多查看译文
关键词
Axial piston pumps, Minimum entropy deconvolution, Convolutional neural network, Fault classification
AI 理解论文
溯源树
样例
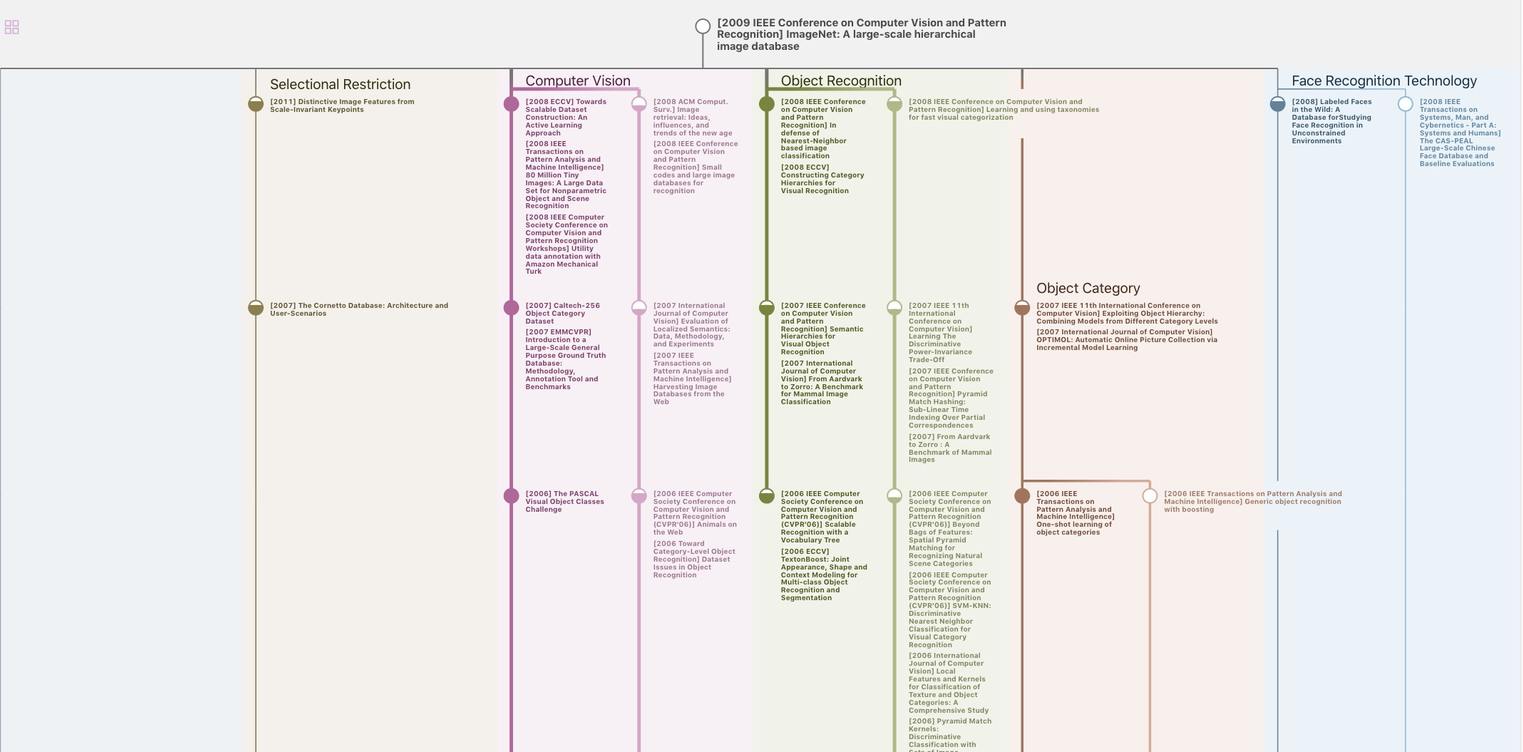
生成溯源树,研究论文发展脉络
Chat Paper
正在生成论文摘要