Learning the blending spikes using sparse dictionaries
GEOPHYSICAL JOURNAL INTERNATIONAL(2020)
摘要
Deblending plays an important role in preparing high-quality seismic data from modern blended simultaneous-source seismic acquisition. State-of-the-art deblending is based on the sparsity-constrained iterative inversion. Inversion-based deblending assumes that the ambient noise level is low and the data misfit during iterative inversion accounts for the random ambient noise. The traditional method becomes problematic when the random ambient noise becomes extremely strong and the inversion iteratively fits the random noise instead of the signal and blending interference. We propose a constrained inversion model that takes the strong random noise into consideration and can achieve satisfactory result even when strong random noise exists. The principle of this new method is that we use sparse dictionaries to learn the blending spikes and thus the learned dictionary atoms are able to distinguish between blending spikes and random noise. The separated signal and blending spikes can then be better fitted by the iterative inversion framework. Synthetic and field data examples are used to demonstrate the performance of the new approach.
更多查看译文
关键词
Image processing,Time-series analysis,Seismic noise
AI 理解论文
溯源树
样例
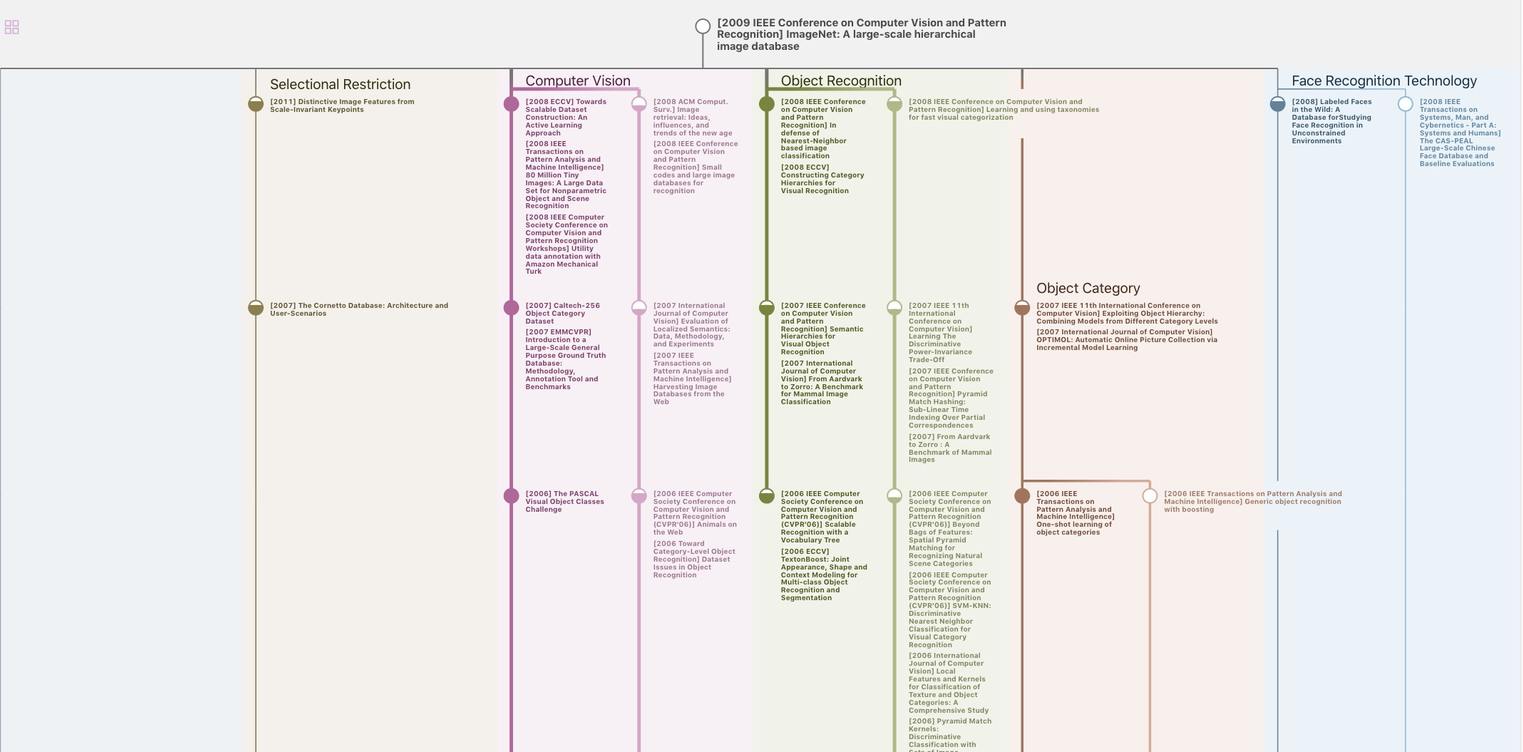
生成溯源树,研究论文发展脉络
Chat Paper
正在生成论文摘要